Enhancing In-Context Learning with Answer Feedback for Multi-Span Question Answering
CoRR(2023)
摘要
Whereas the recent emergence of large language models (LLMs) like ChatGPT has exhibited impressive general performance, it still has a large gap with fully-supervised models on specific tasks such as multi-span question answering. Previous researches found that in-context learning is an effective approach to exploiting LLM, by using a few task-related labeled data as demonstration examples to construct a few-shot prompt for answering new questions. A popular implementation is to concatenate a few questions and their correct answers through simple templates, informing LLM of the desired output. In this paper, we propose a novel way of employing labeled data such that it also informs LLM of some undesired output, by extending demonstration examples with feedback about answers predicted by an off-the-shelf model, e.g., correct, incorrect, or incomplete. Experiments on three multi-span question answering datasets as well as a keyphrase extraction dataset show that our new prompting strategy consistently improves LLM's in-context learning performance.
更多查看译文
关键词
answer feedback,learning,in-context,multi-span
AI 理解论文
溯源树
样例
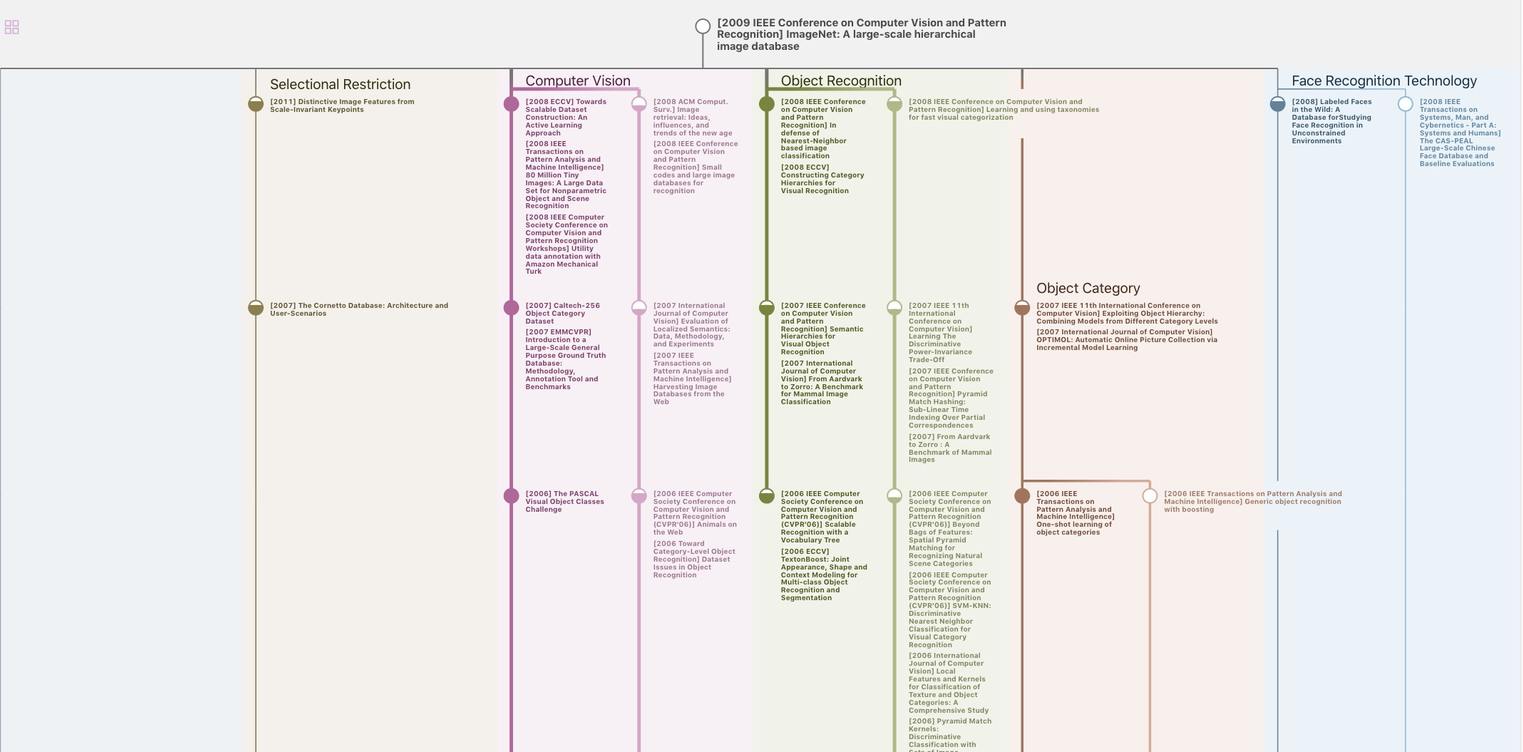
生成溯源树,研究论文发展脉络
Chat Paper
正在生成论文摘要