Adversarial Sample Detection Through Neural Network Transport Dynamics
MACHINE LEARNING AND KNOWLEDGE DISCOVERY IN DATABASES: RESEARCH TRACK, ECML PKDD 2023, PT I(2023)
摘要
We propose a detector of adversarial samples that is based on the view of neural networks as discrete dynamic systems. The detector tells clean inputs from abnormal ones by comparing the discrete vector fields they follow through the layers. We also show that regularizing this vector field during training makes the network more regular on the data distribution's support, thus making the activations of clean inputs more distinguishable from those of abnormal ones. Experimentally, we compare our detector favorably to other detectors on seen and unseen attacks, and show that the regularization of the network's dynamics improves the performance of adversarial detectors that use the internal embeddings as inputs, while also improving test accuracy.
更多查看译文
关键词
Deep learning,Adversarial detection,Optimal transport
AI 理解论文
溯源树
样例
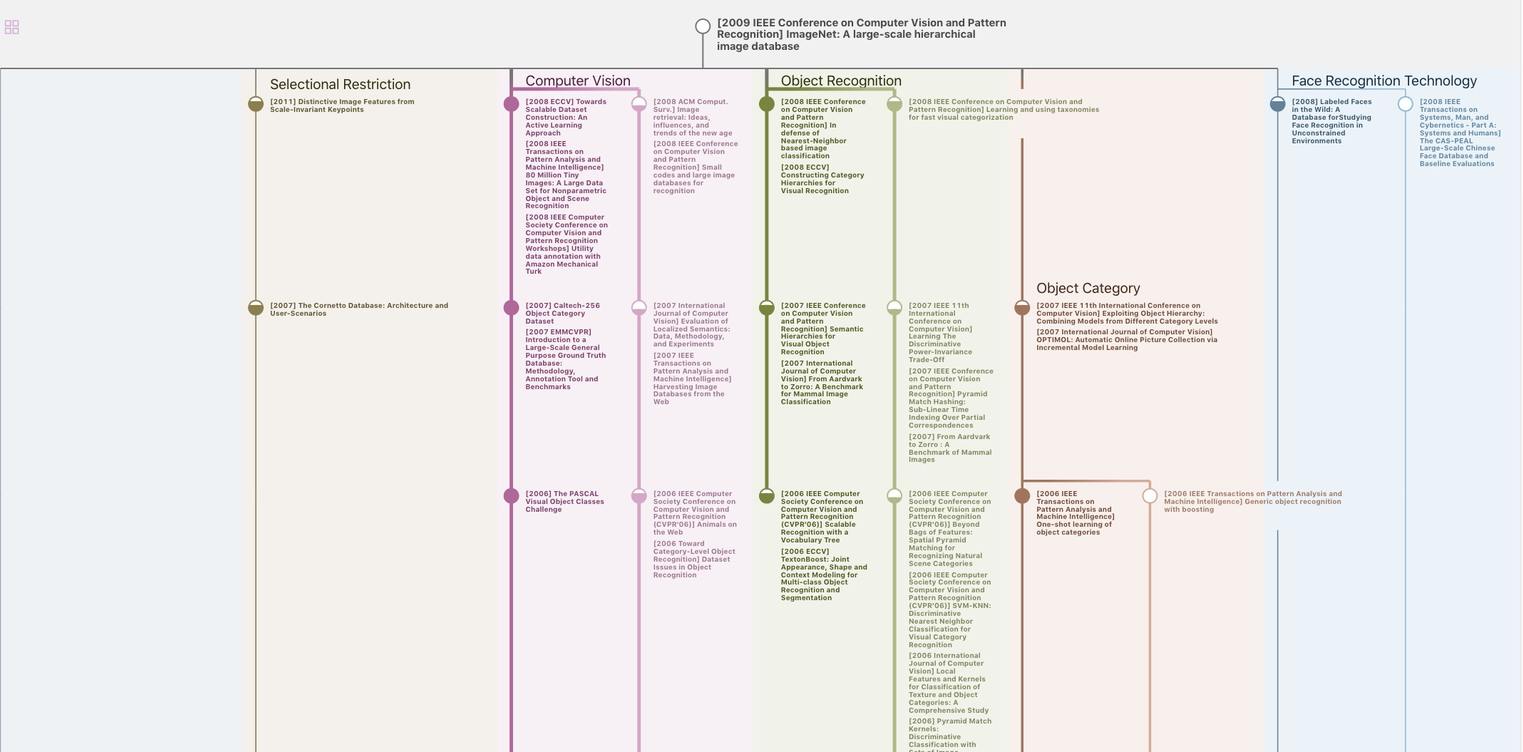
生成溯源树,研究论文发展脉络
Chat Paper
正在生成论文摘要