Multi-constrained Symmetric Nonnegative Latent Factor Analysis for Accurately Representing Large-scale Undirected Weighted Networks
CoRR(2023)
摘要
An Undirected Weighted Network (UWN) is frequently encountered in a big-data-related application concerning the complex interactions among numerous nodes, e.g., a protein interaction network from a bioinformatics application. A Symmetric High-Dimensional and Incomplete (SHDI) matrix can smoothly illustrate such an UWN, which contains rich knowledge like node interaction behaviors and local complexes. To extract desired knowledge from an SHDI matrix, an analysis model should carefully consider its symmetric-topology for describing an UWN's intrinsic symmetry. Representation learning to an UWN borrows the success of a pyramid of symmetry-aware models like a Symmetric Nonnegative Matrix Factorization (SNMF) model whose objective function utilizes a sole Latent Factor (LF) matrix for representing SHDI's symmetry rigorously. However, they suffer from the following drawbacks: 1) their computational complexity is high; and 2) their modeling strategy narrows their representation features, making them suffer from low learning ability. Aiming at addressing above critical issues, this paper proposes a Multi-constrained Symmetric Nonnegative Latent-factor-analysis (MSNL) model with two-fold ideas: 1) introducing multi-constraints composed of multiple LF matrices, i.e., inequality and equality ones into a data-density-oriented objective function for precisely representing the intrinsic symmetry of an SHDI matrix with broadened feature space; and 2) implementing an Alternating Direction Method of Multipliers (ADMM)-incorporated learning scheme for precisely solving such a multi-constrained model. Empirical studies on three SHDI matrices from a real bioinformatics or industrial application demonstrate that the proposed MSNL model achieves stronger representation learning ability to an SHDI matrix than state-of-the-art models do.
更多查看译文
关键词
multi-constrained,large-scale
AI 理解论文
溯源树
样例
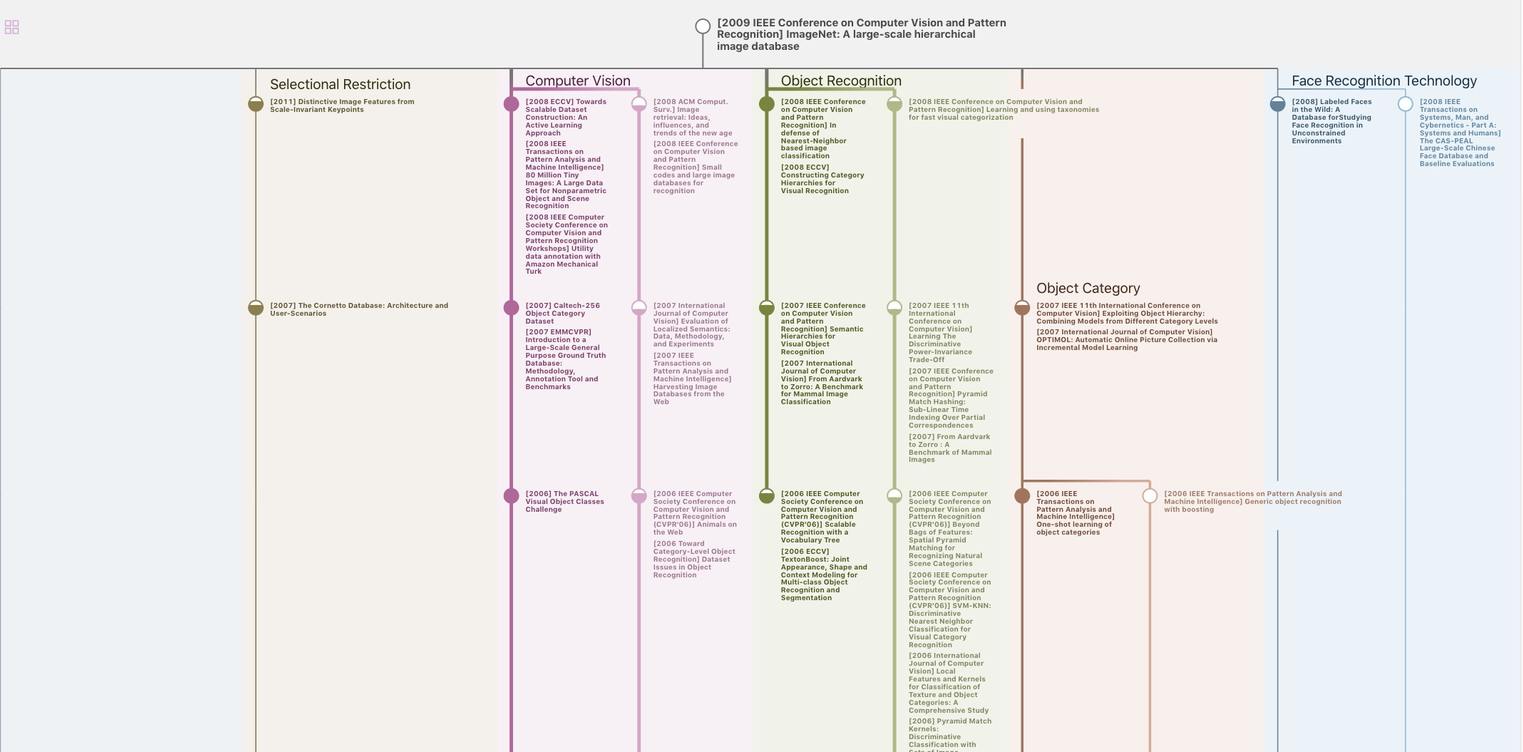
生成溯源树,研究论文发展脉络
Chat Paper
正在生成论文摘要