Consensus Cluster Center Guided Latent Multi-Kernel Clustering
IEEE Transactions on Circuits and Systems for Video Technology(2023)
摘要
Existing multi-kernel clustering (MKC) methods usually focus on constructing a fixed dimension consensus-partition from base kernels to demonstrate their superior in integrating complementary information. Despite their success, they still suffer from the following limitations: (1) The size of consensus-partition is always fixed as the upper bound (k = n) or lower bound (k = c), where n, c, and k are the number of samples, clusters, and partition dimension, respectively, resulting in suboptimal partition; (2) The learned consensus-partition cannot make full use of the global distribution information hidden in data. To address these issues, we propose a latent consensus-partition learning framework for MKC, namely Consensus Cluster Center Guided Latent Multi-kernel Clustering ((CLMC)-L-3), including two methods, i.e., (CLMCK)-L-3 and (CLMCH)-L-3. For (CLMCK)-L-3, we flexibly search for a more proper dimension of consensus-partition in a latent embedding space rather than the fixed partition dimension. Meanwhile, the generation of latent consensus-partition is guided by a consensus cluster center of base kernels, such that global distribution information hidden in base kernels can be captured fully. However, (CLMCK)-L-3 suffers from O(n(2)) computational complexity and memory complexity. Thus, we further propose (CLMCH)-L-3 to handle large-scale data by reducing both kinds of complexities to O(n). Two solvers with convergence proof are developed to validate our effectiveness, superiority, and efficiency on multiple public datasets with the recent advances.
更多查看译文
关键词
Multi-kernel clustering,latent consensus-partition learning,consensus cluster center
AI 理解论文
溯源树
样例
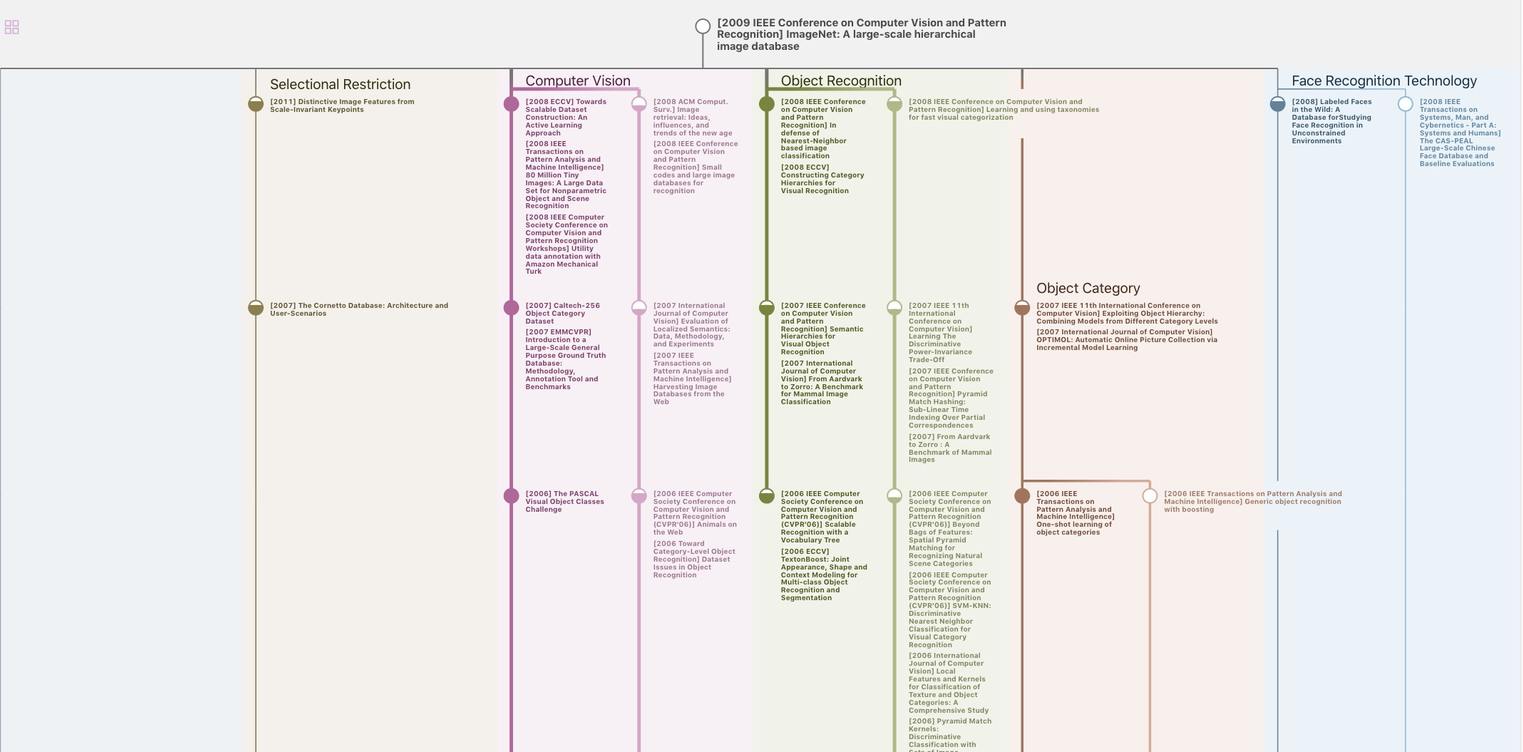
生成溯源树,研究论文发展脉络
Chat Paper
正在生成论文摘要