PQM: A Point Quality Evaluation Metric for Dense Maps
CoRR(2023)
摘要
LiDAR-based mapping/reconstruction are important for various applications, but evaluating the quality of the dense maps they produce is challenging. The current methods have limitations, including the inability to capture completeness, structural information, and local variations in error. In this paper, we propose a novel point quality evaluation metric (PQM) that consists of four sub-metrics to provide a more comprehensive evaluation of point cloud quality. The completeness sub-metric evaluates the proportion of missing data, the artifact score sub-metric recognizes and characterizes artifacts, the accuracy sub-metric measures registration accuracy, and the resolution sub-metric quantifies point cloud density. Through an ablation study using a prototype dataset, we demonstrate the effectiveness of each of the sub-metrics and compare them to popular point cloud distance measures. Using three LiDAR SLAM systems to generate maps, we evaluate their output map quality and demonstrate the metrics robustness to noise and artifacts. Our implementation of PQM, datasets and detailed documentation on how to integrate with your custom dense mapping pipeline can be found at github.com/droneslab/pqm
更多查看译文
关键词
point quality evaluation metric,maps,quality evaluation
AI 理解论文
溯源树
样例
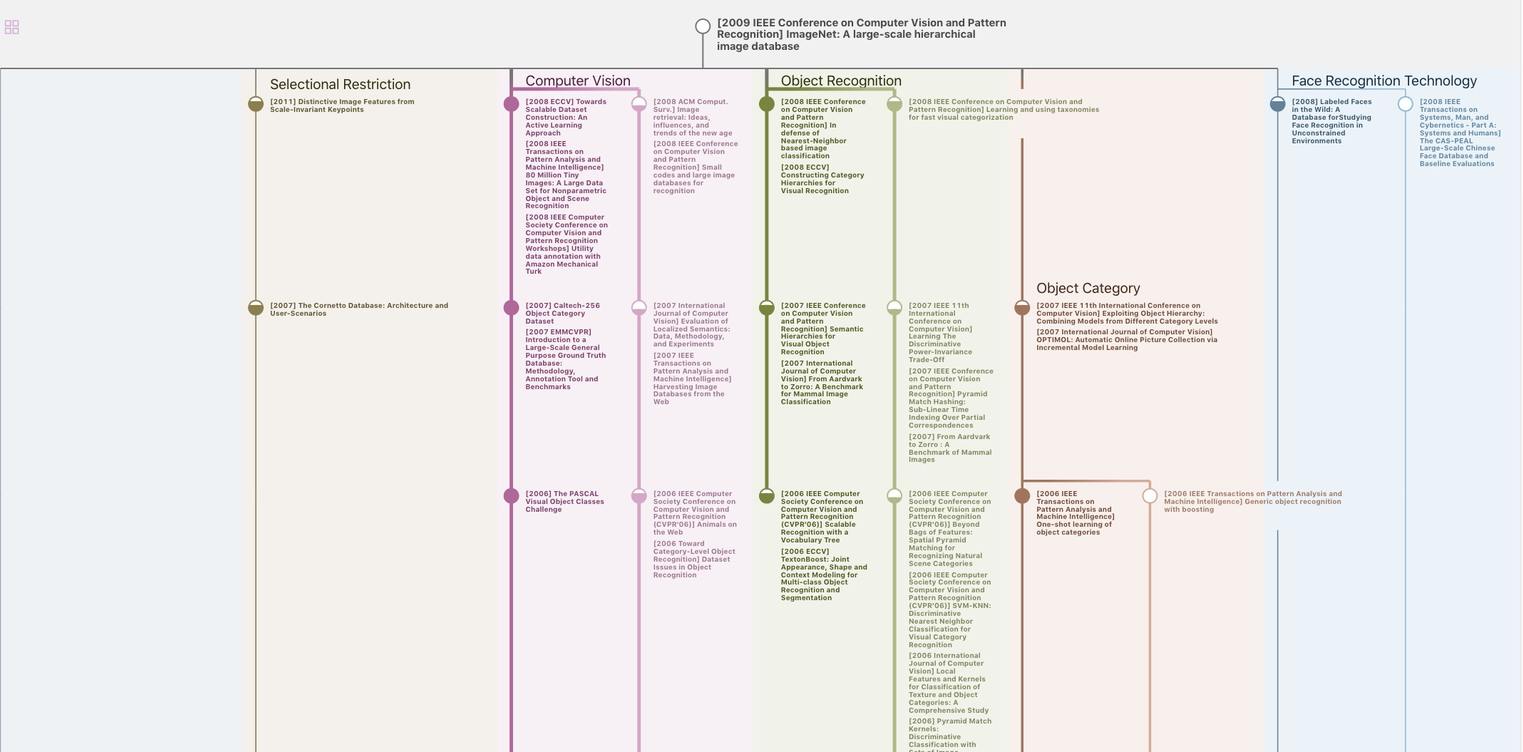
生成溯源树,研究论文发展脉络
Chat Paper
正在生成论文摘要