Continual Learning in Linear Classification on Separable Data
CoRR(2023)
摘要
We analyze continual learning on a sequence of separable linear classification tasks with binary labels. We show theoretically that learning with weak regularization reduces to solving a sequential max-margin problem, corresponding to a special case of the Projection Onto Convex Sets (POCS) framework. We then develop upper bounds on the forgetting and other quantities of interest under various settings with recurring tasks, including cyclic and random orderings of tasks. We discuss several practical implications to popular training practices like regularization scheduling and weighting. We point out several theoretical differences between our continual classification setting and a recently studied continual regression setting.
更多查看译文
关键词
linear classification,learning,data
AI 理解论文
溯源树
样例
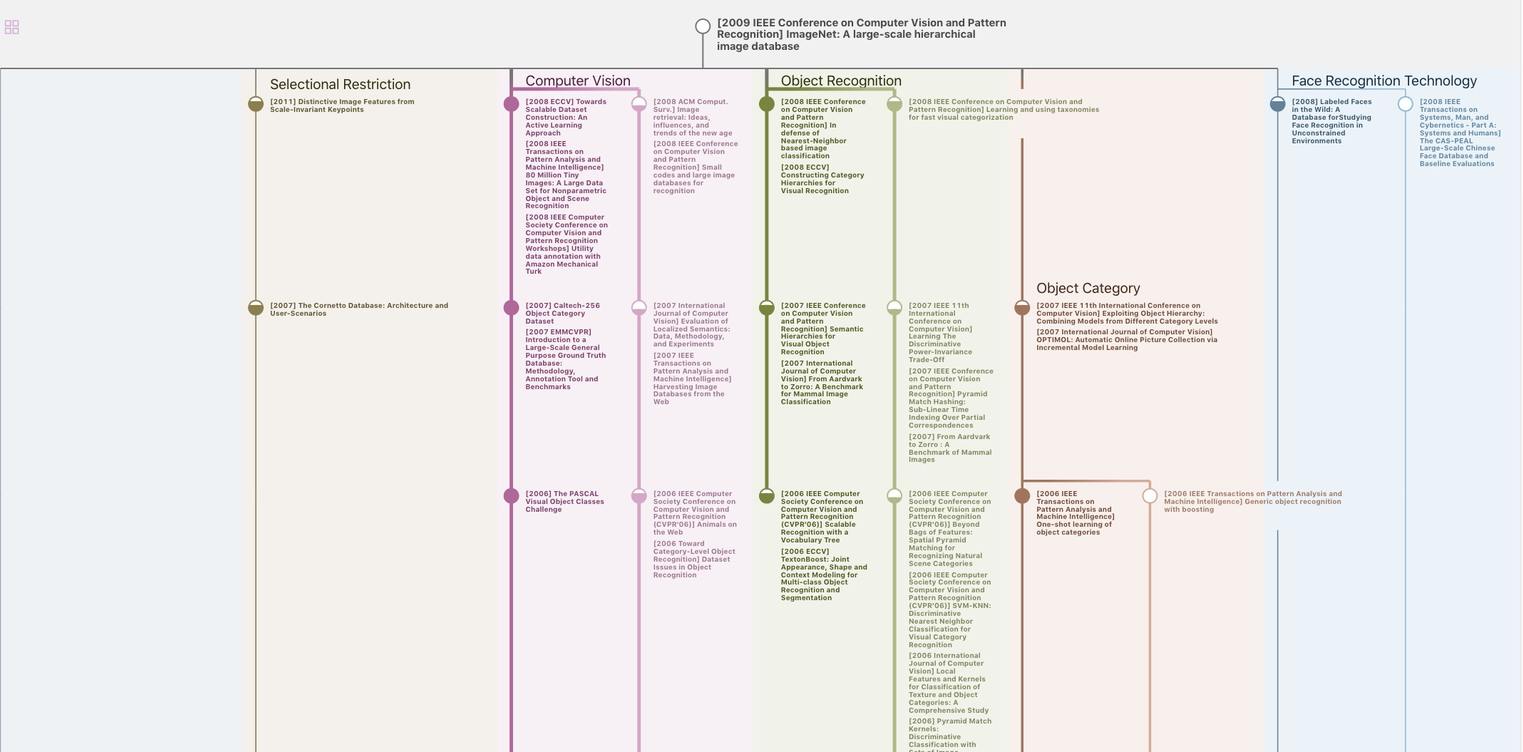
生成溯源树,研究论文发展脉络
Chat Paper
正在生成论文摘要