Logic Diffusion for Knowledge Graph Reasoning
CoRR(2023)
摘要
Most recent works focus on answering first order logical queries to explore the knowledge graph reasoning via multi-hop logic predictions. However, existing reasoning models are limited by the circumscribed logical paradigms of training samples, which leads to a weak generalization of unseen logic. To address these issues, we propose a plug-in module called Logic Diffusion (LoD) to discover unseen queries from surroundings and achieves dynamical equilibrium between different kinds of patterns. The basic idea of LoD is relation diffusion and sampling sub-logic by random walking as well as a special training mechanism called gradient adaption. Besides, LoD is accompanied by a novel loss function to further achieve the robust logical diffusion when facing noisy data in training or testing sets. Extensive experiments on four public datasets demonstrate the superiority of mainstream knowledge graph reasoning models with LoD over state-of-the-art. Moreover, our ablation study proves the general effectiveness of LoD on the noise-rich knowledge graph.
更多查看译文
关键词
logic,reasoning,knowledge,diffusion
AI 理解论文
溯源树
样例
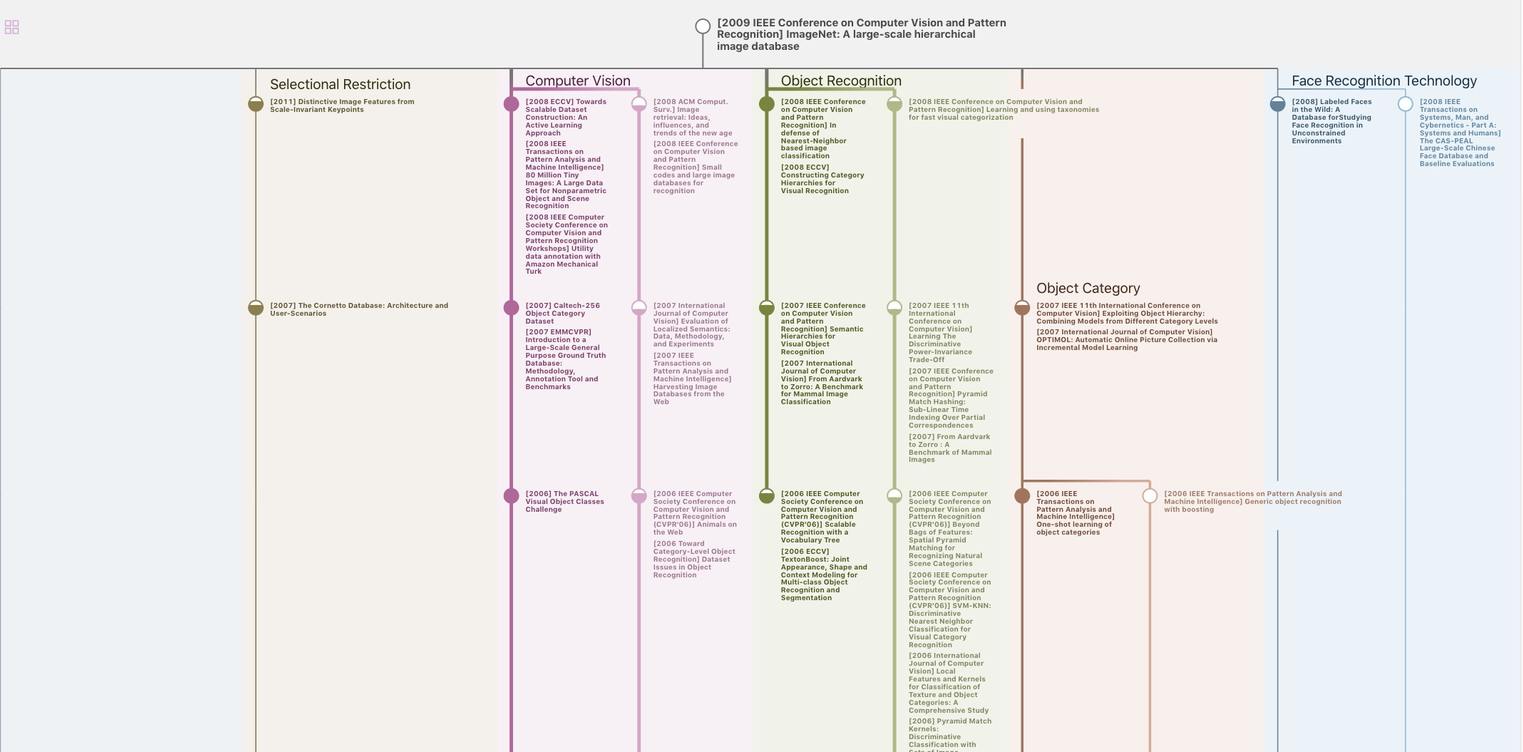
生成溯源树,研究论文发展脉络
Chat Paper
正在生成论文摘要