Stabilizing Contrastive RL: Techniques for Robotic Goal Reaching from Offline Data
arXiv (Cornell University)(2023)
Abstract
Robotic systems that rely primarily on self-supervised learning have the
potential to decrease the amount of human annotation and engineering effort
required to learn control strategies. In the same way that prior robotic
systems have leveraged self-supervised techniques from computer vision (CV) and
natural language processing (NLP), our work builds on prior work showing that
the reinforcement learning (RL) itself can be cast as a self-supervised
problem: learning to reach any goal without human-specified rewards or labels.
Despite the seeming appeal, little (if any) prior work has demonstrated how
self-supervised RL methods can be practically deployed on robotic systems. By
first studying a challenging simulated version of this task, we discover design
decisions about architectures and hyperparameters that increase the success
rate by 2 ×. These findings lay the groundwork for our main result: we
demonstrate that a self-supervised RL algorithm based on contrastive learning
can solve real-world, image-based robotic manipulation tasks, with tasks being
specified by a single goal image provided after training.
MoreTranslated text
Key words
contrastive rl,offline goal
AI Read Science
Must-Reading Tree
Example
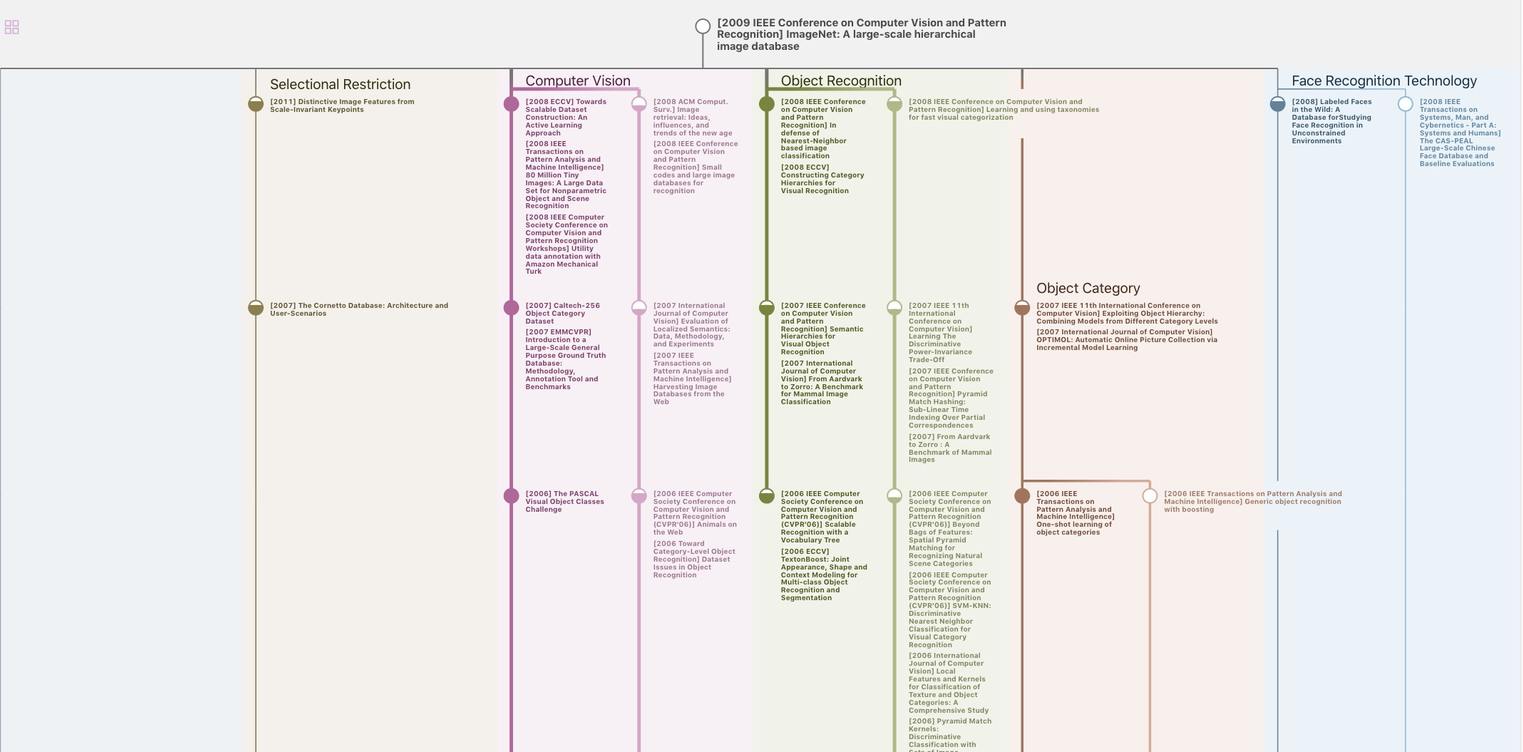
Generate MRT to find the research sequence of this paper
Chat Paper
Summary is being generated by the instructions you defined