Inference-Time Intervention: Eliciting Truthful Answers from a Language Model
NeurIPS(2023)
摘要
We introduce Inference-Time Intervention (ITI), a technique designed to enhance the truthfulness of large language models (LLMs). ITI operates by shifting model activations during inference, following a set of directions across a limited number of attention heads. This intervention significantly improves the performance of LLaMA models on the TruthfulQA benchmark. On an instruction-finetuned LLaMA called Alpaca, ITI improves its truthfulness from 32.5% to 65.1%. We identify a tradeoff between truthfulness and helpfulness and demonstrate how to balance it by tuning the intervention strength. ITI is minimally invasive and computationally inexpensive. Moreover, the technique is data efficient: while approaches like RLHF require extensive annotations, ITI locates truthful directions using only few hundred examples. Our findings suggest that LLMs may have an internal representation of the likelihood of something being true, even as they produce falsehoods on the surface.
更多查看译文
关键词
truthful answers,intervention,language
AI 理解论文
溯源树
样例
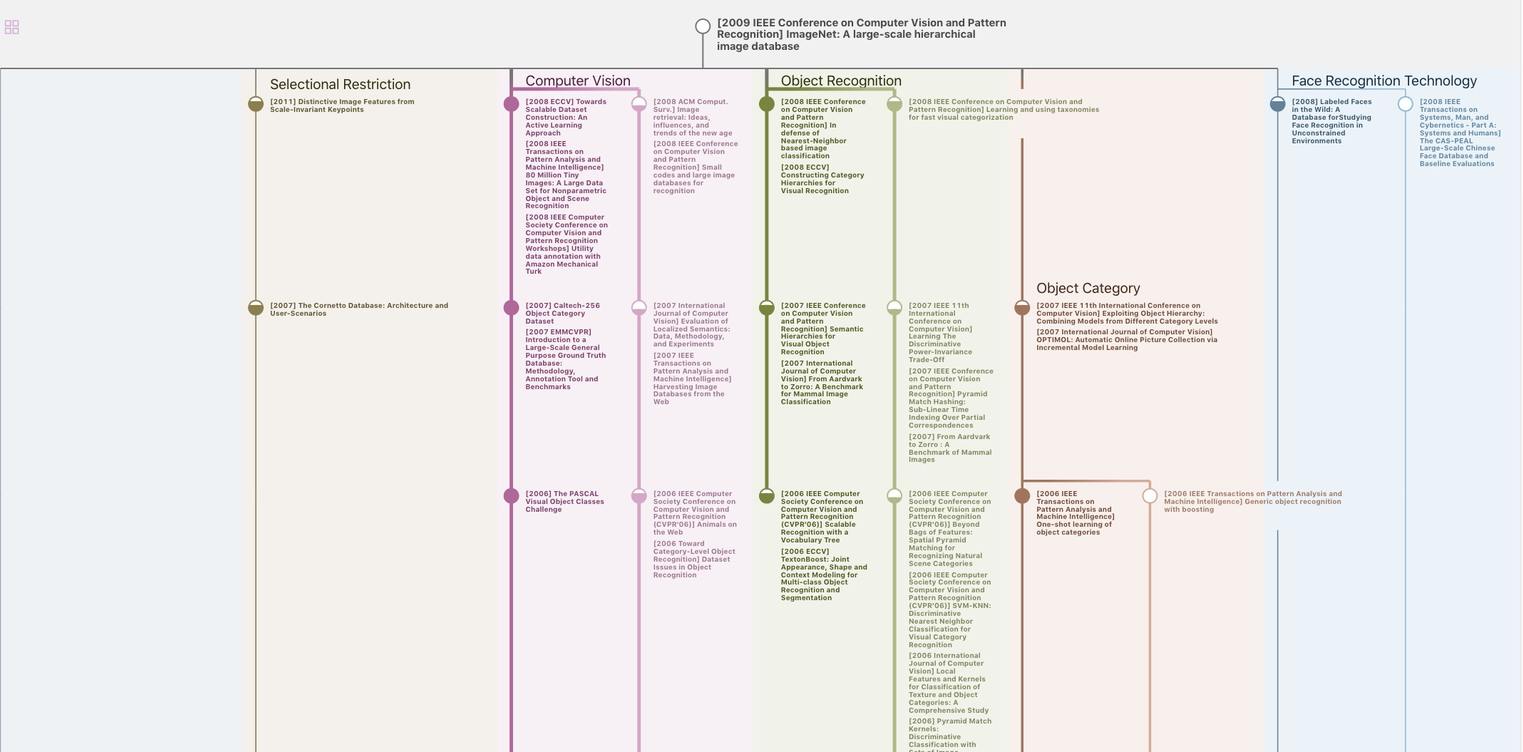
生成溯源树,研究论文发展脉络
Chat Paper
正在生成论文摘要