Understanding the Effectiveness of Early Weight Averaging for Training Large Language Models
CoRR(2023)
摘要
Training LLMs is expensive, and recent evidence indicates training all the way to convergence is inefficient. In this paper, we investigate the ability of a simple idea, checkpoint averaging along the trajectory of a training run to improve the quality of models before they have converged. This approach incurs no extra cost during training or inference. Specifically, we analyze the training trajectories of Pythia LLMs with 1 to 12 billion parameters and demonstrate that, particularly during the early to mid stages of training, this idea accelerates convergence and improves both test and zero-shot generalization. Loss spikes are a well recognized problem in LLM training; in our analysis we encountered two instances of this in the underlying trajectories, and both instances were mitigated by our averaging. For a 6.9B parameter LLM, for example, our early weight averaging recipe can save upto 4200 hours of GPU time, which corresponds to significant savings in cloud compute costs.
更多查看译文
关键词
large language models,early weight averaging,training
AI 理解论文
溯源树
样例
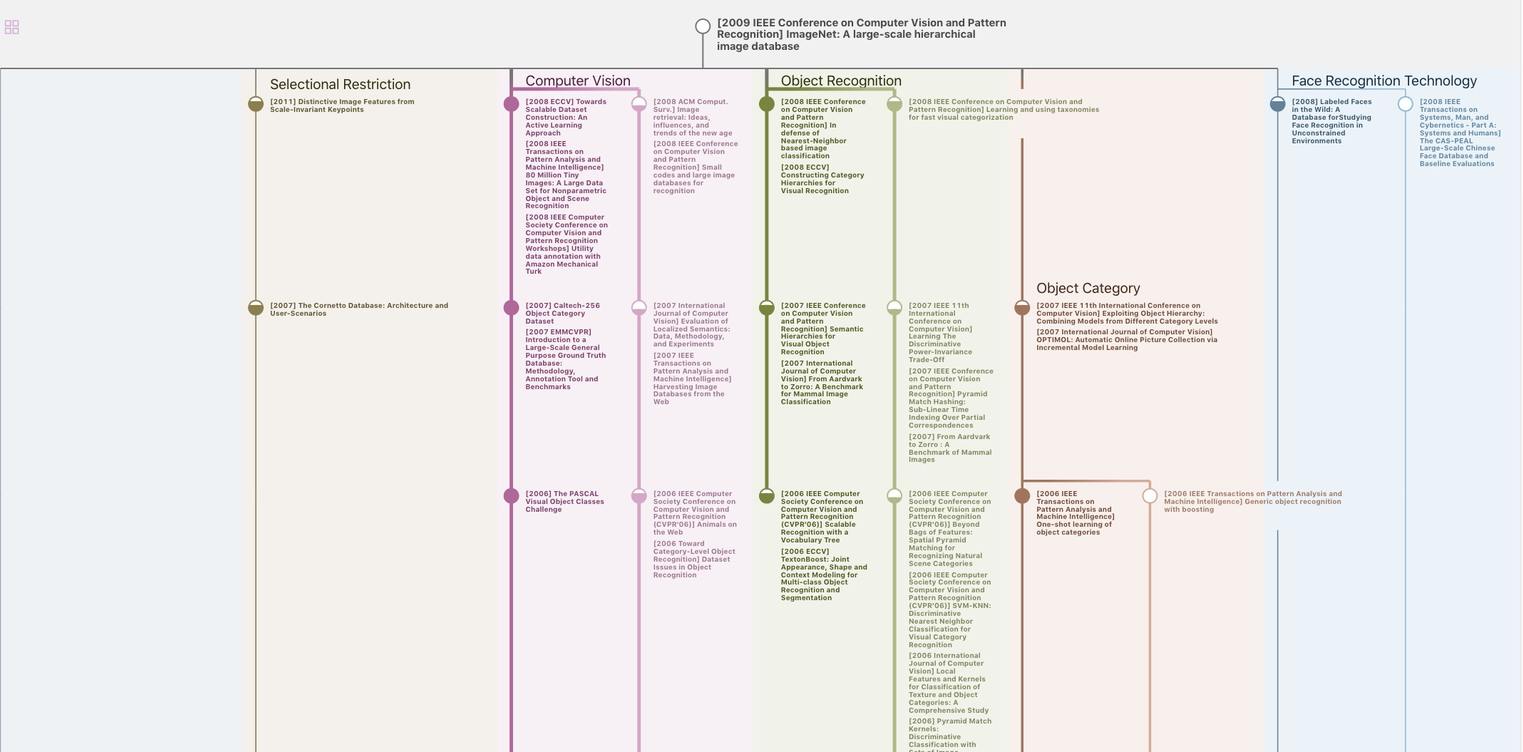
生成溯源树,研究论文发展脉络
Chat Paper
正在生成论文摘要