Digital Twin of a Driver-in-the-Loop Race Car Simulation With Contextual Reinforcement Learning
IEEE Robotics and Automation Letters(2023)
摘要
In order to facilitate rapid prototyping and testing in the advanced motorsport industry, we consider the problem of imitating and outperforming professional race car drivers based on demonstrations collected on a high-fidelity Driver-in-the-Loop (DiL) hardware simulator. We formulate a contextual reinforcement learning problem to learn a human-like and stochastic policy with domain-informed choices for states, actions, and reward functions. To leverage very limited training data and build human-like diverse behavior, we fit a probabilistic model to the expert demonstrations called the reference distribution, draw samples out of it, and use them as context for the reinforcement learning agent with context-specific states and rewards. In contrast to the non-human-like stochasticity introduced by Gaussian noise, our method contributes to a more effective exploration, better performance and a policy with human-like variance in evaluation metrics. Compared to previous work using a behavioral cloning agent, which is unable to complete competitive laps robustly, our agent outperforms the professional driver used to collect the demonstrations by around 0.4 seconds per lap on average, which is the first time known to the authors that an autonomous agent has outperformed a top-class professional race driver in a state-of-the-art, high-fidelity simulation. Being robust and sensitive to vehicle setup changes, our agent is able to predict plausible lap time and other performance metrics. Furthermore, unlike traditional lap time calculation methods, our agent indicates not only the gain in performance but also the driveability when faced with modified car balance, facilitating the digital twin of the DiL simulation.
更多查看译文
关键词
Intelligent vehicles,Behavioral sciences,Reinforcement learning,Predictive models,Digital twins,Vehicle dynamics,Automotive engineering,imitation leaning,autonomous agent,autonomous racing
AI 理解论文
溯源树
样例
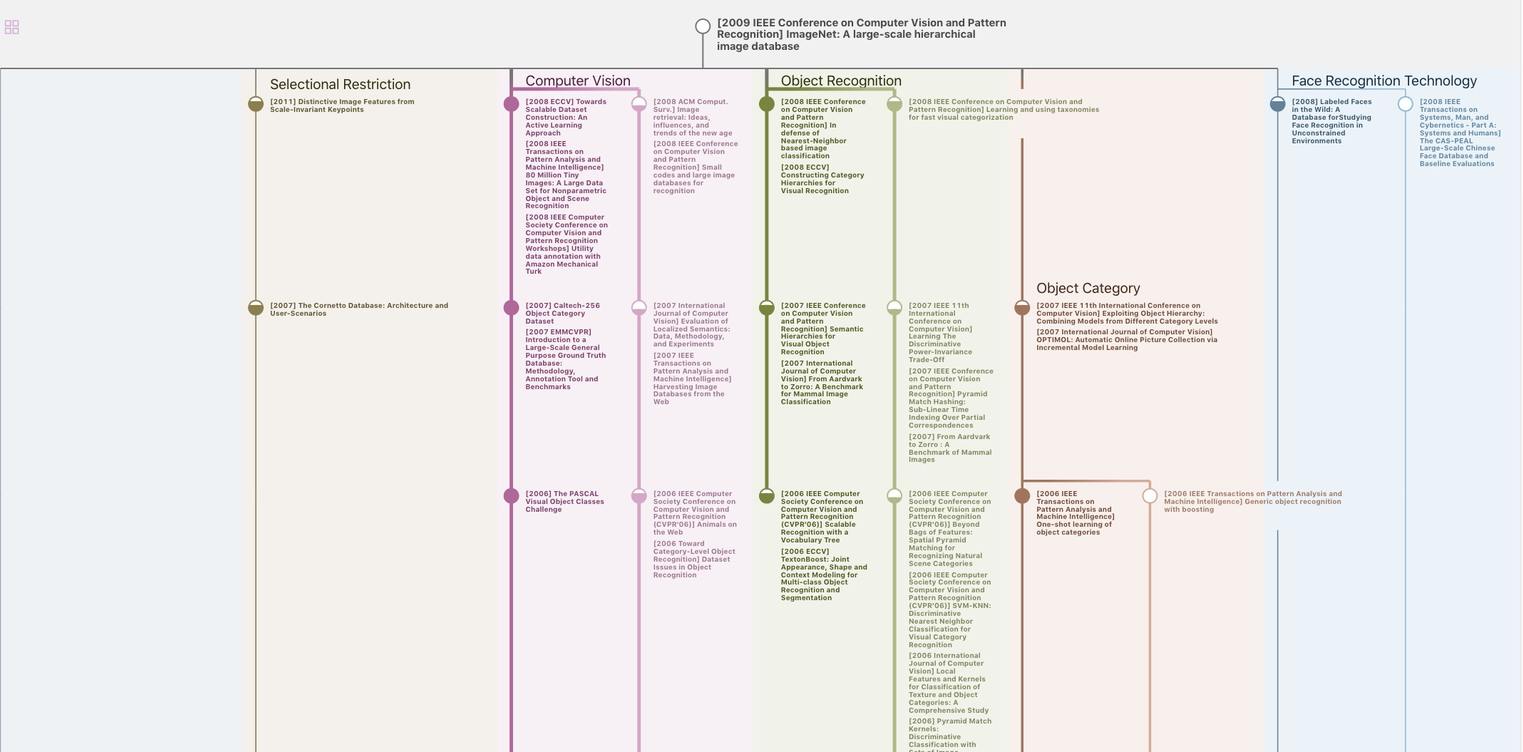
生成溯源树,研究论文发展脉络
Chat Paper
正在生成论文摘要