Attention-based BILSTM for the degradation trend prediction of lithium battery
ENERGY REPORTS(2023)
摘要
There is an irreversibility in the decline of Li-ion batteries, and the performance of individual cells in the battery pack will gradually decline as the number of times the on-board Li-ion battery is charged and discharged increases This situation can significantly affect the daily use of electric vehicles, for example by shortening the driving range, and in addition, the deterioration of the battery performance increases the probability of electric vehicle breakdowns. Very little work has been done on the prediction of lithium battery performance degradation in long-mileage states, accurate prediction of future battery performance degradation can significantly reduce the probability of EV failure, making battery performance prediction very important. In this paper, we propose a BILSTM network based on an attention mechanism and utilize grey relation analysis and empirical modal decomposition in the input link of the network to address the shortcomings exposed by deep learning in the work on temporal prediction. The adopted approach can effectively address the impact of data noise and redundant features on the prediction work that occurs in deep learning. According to the experimental results, the prediction performance of the model proposed in this paper is found to be higher than other networks in both types of data sets (c) 2023 The Author(s). Published by Elsevier Ltd. This is an open access article under theCCBY-NC-ND license (http://creativecommons.org/licenses/by-nc-nd/4.0/).
更多查看译文
关键词
Battery performance,Bi-directional long and short-term memory,Attention mechanism,Grey relation analysis,Empirical modal decomposition
AI 理解论文
溯源树
样例
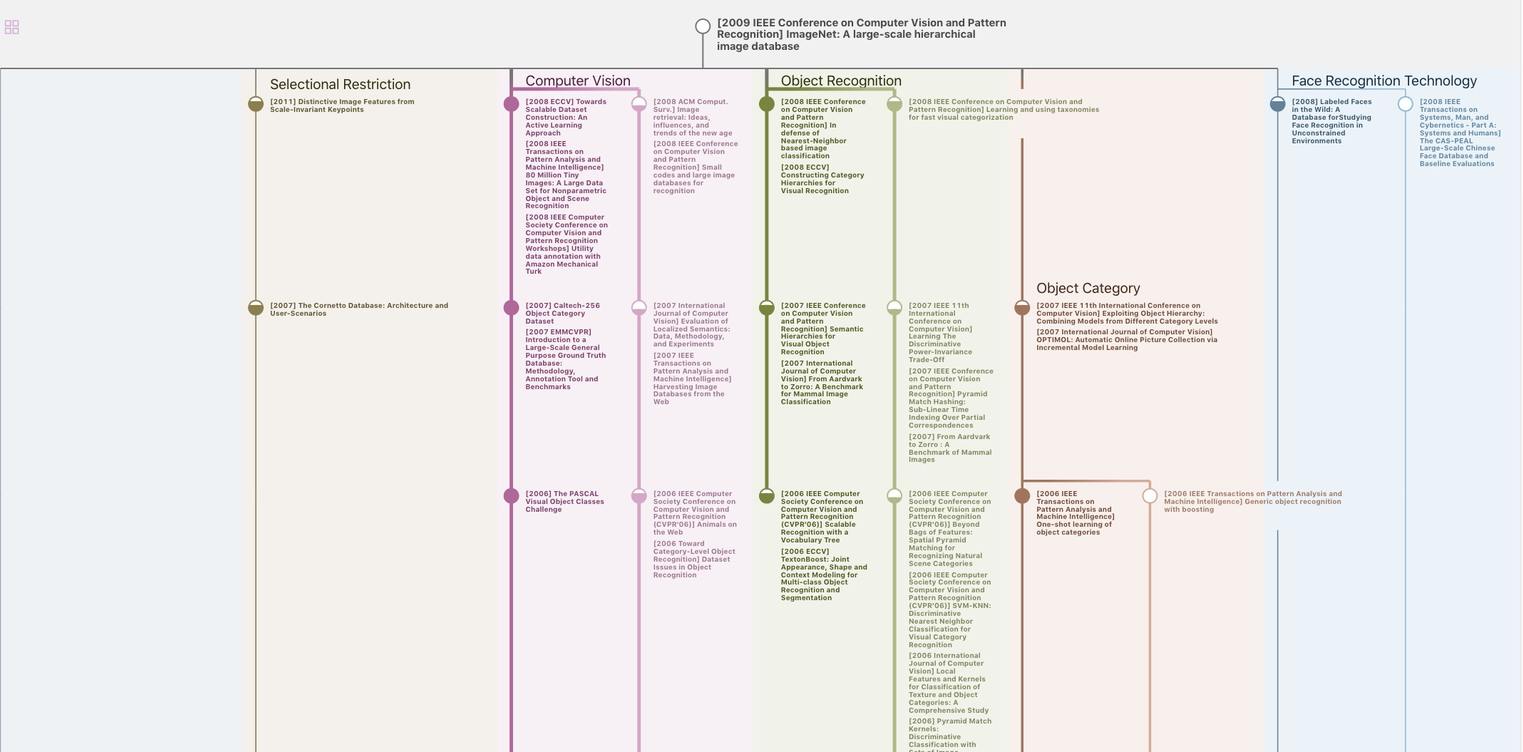
生成溯源树,研究论文发展脉络
Chat Paper
正在生成论文摘要