Using a Multi-Site RCT to Predict Impacts for a Single Site: Do Better Data and Methods Yield More Accurate Predictions?
JOURNAL OF RESEARCH ON EDUCATIONAL EFFECTIVENESS(2024)
摘要
Multi-site randomized controlled trials (RCTs) provide unbiased estimates of the average impact in the study sample. However, their ability to accurately predict the impact for individual sites outside the study sample, to inform local policy decisions, is largely unknown. To extend prior research on this question, we analyzed six multi-site RCTs and tested modern prediction methods-lasso regression and Bayesian Additive Regression Trees (BART)-using a wide range of moderator variables. The main study findings are that: (1) all of the methods yielded accurate impact predictions when the variation in impacts across sites was close to zero (as expected); (2) none of the methods yielded accurate impact predictions when the variation in impacts across sites was substantial; and (3) BART typically produced "less inaccurate" predictions than lasso regression or than the Sample Average Treatment Effect. These results raise concerns that when the impact of an intervention varies considerably across sites, statistical modeling using the data commonly collected by multi-site RCTs will be insufficient to explain the variation in impacts across sites and accurately predict impacts for individual sites.
更多查看译文
关键词
Randomized controlled trials,generalizability,external validity,evidence-based policy,transportability
AI 理解论文
溯源树
样例
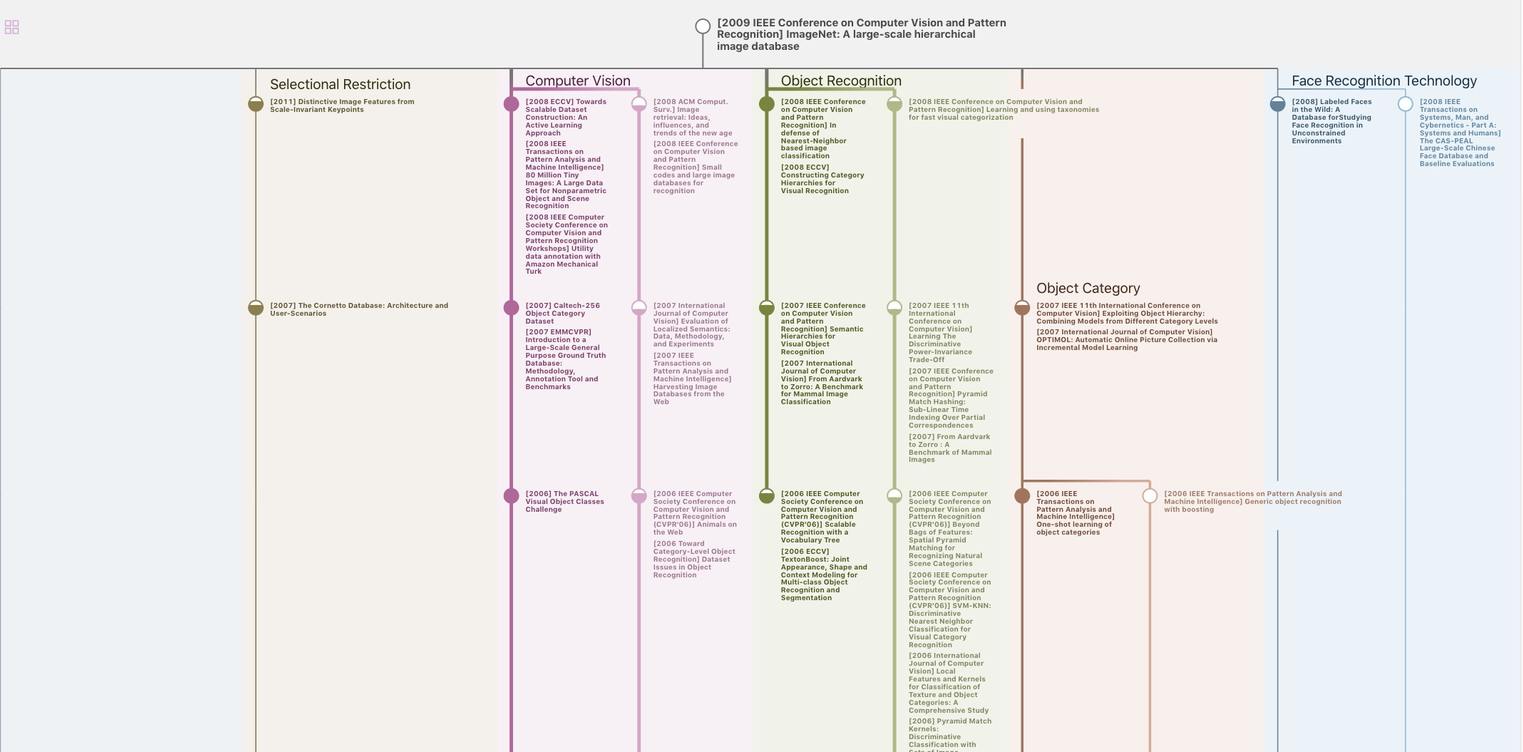
生成溯源树,研究论文发展脉络
Chat Paper
正在生成论文摘要