Learning Target Point Seeking Weights Spatial–Temporal Regularized Correlation Filters for Visual Tracking
NEURAL PROCESSING LETTERS(2023)
摘要
Spatial–temporal regularized correlation filtering is an effective tracking algorithm. Still, problems such as target occlusion, background clutter and out of view caused by target movement are inevitable in the running process. This paper proposes a target point seeking weights spatial–temporal regularized correlation filters to tackle target loss. The alternating direction method of multipliers is used to simplify the spatial–temporal regularized term in the objective function, obtain the optimal solution of the filter and auxiliary factor, reduce the algorithm’s complexity, and realize the adaptability of time and space. The target weight response model is obtained through the target point finding weight, the target motion information is extracted, the target motion state and motion trajectory are predicted, and the interference information such as the algorithm’s ability to identify the target and the ability to distinguish the target is enhanced. With the help of the weighted least squares method, the maximum response value of the weight is obtained, and the target position is determined. Experiments show that, compared with other mainstream correlation filtering algorithms, the proposed algorithm has higher tracking accuracy and stronger robustness under complex conditions.
更多查看译文
关键词
spatial–temporal regularized correlation filters,target point,weights spatial–temporal
AI 理解论文
溯源树
样例
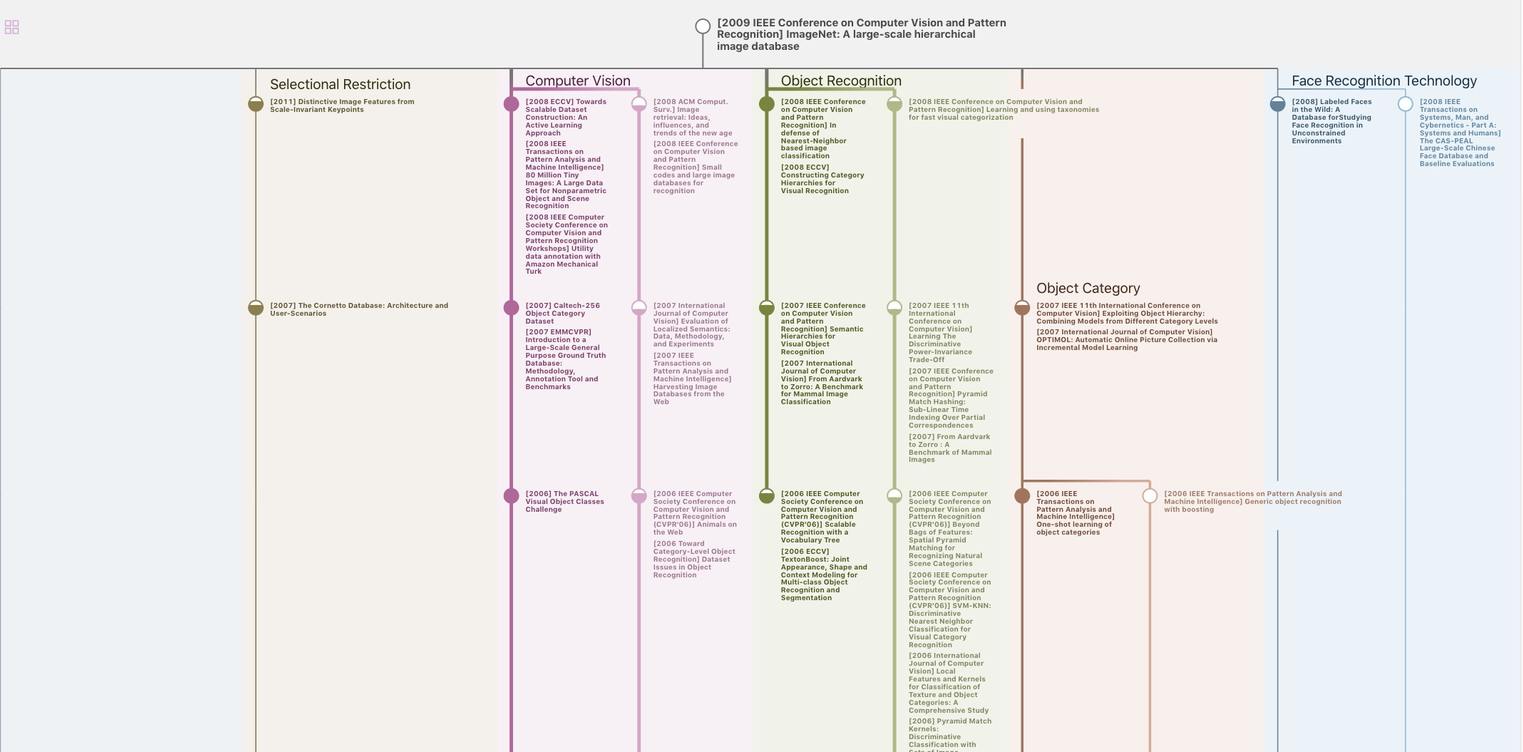
生成溯源树,研究论文发展脉络
Chat Paper
正在生成论文摘要