An adaptive variance vector-based evolutionary algorithm for large scale multi-objective optimization
NEURAL COMPUTING & APPLICATIONS(2023)
摘要
Large scale multi-objective optimization problems often involve hundreds or thousands of decision variables. Regular methods tend to divide decision variables into multiple groups by identifying the contributions to objectives. However, they may suffer from a large computational budget prior to the start of optimization, resulting in a less computational budget for the actual optimization of problems. Different from them, this paper proposes an adaptive variance vector strategy, which is able to identify convergence-related and diversity-related variables by the variance features of variables in the decision space. The adaptive variance vector not only consumes no additional computational budget, but also is proved to be empirically effective in categorizing decision variables. Based on the adaptive variance vector strategy, an adaptive variance vector-based evolutionary algorithm is designed for tackling large scale multi-objective optimization. Experimental results and empirical analyses on LSMOP and DTLZ test suites with up to 5000 decision variables demonstrate the effectiveness of the adaptive variance vector strategy in identifying the convergence-related and diversity-related variables, and the superiority of the proposed method over state-of-the-art methods in terms of the convergence and diversity.
更多查看译文
关键词
evolutionary algorithm,optimization,adaptive,vector-based,multi-objective
AI 理解论文
溯源树
样例
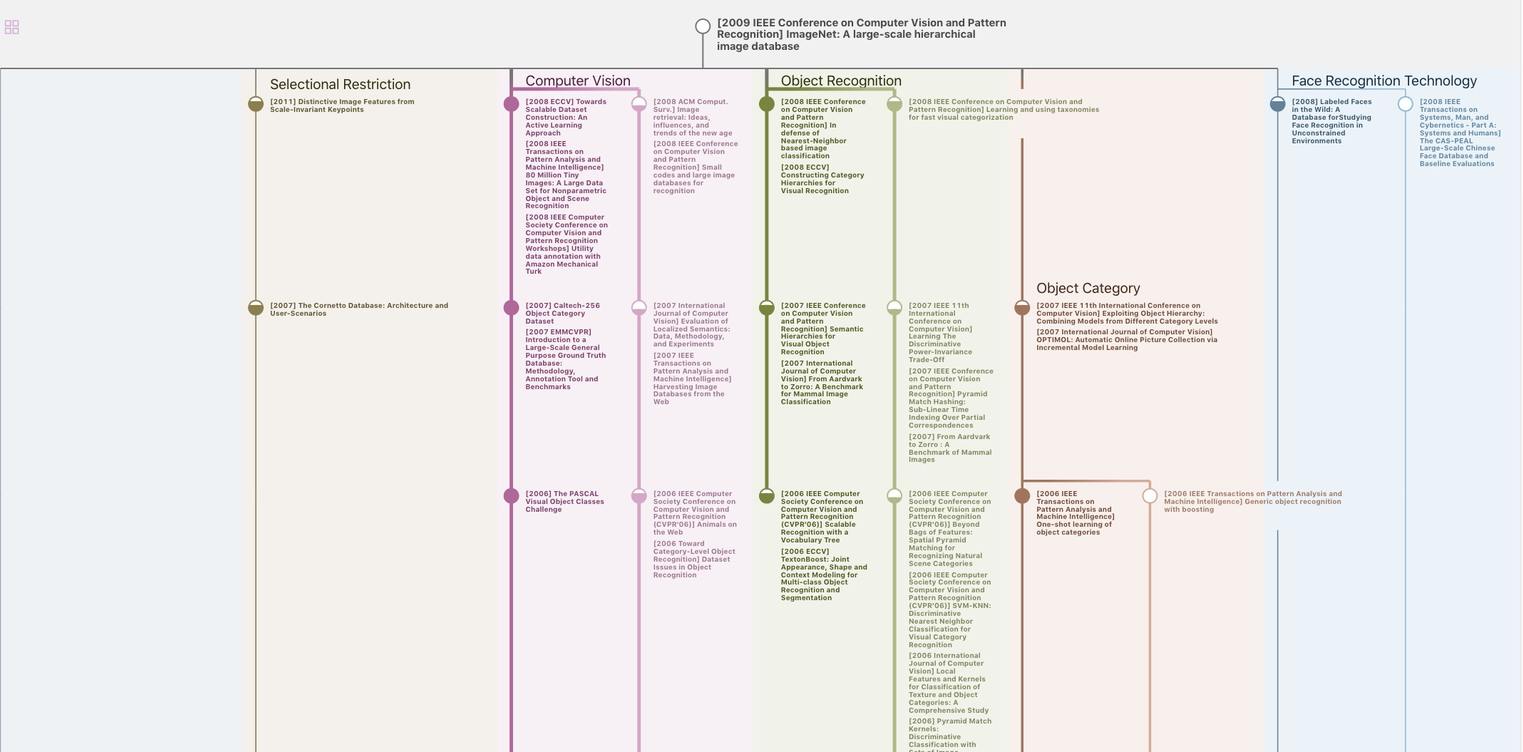
生成溯源树,研究论文发展脉络
Chat Paper
正在生成论文摘要