A dual-attention feature fusion network for imbalanced fault diagnosis with two-stream hybrid generated data
JOURNAL OF INTELLIGENT MANUFACTURING(2024)
摘要
Deep learning-based fault diagnosis models achieve great success with sufficient balanced data, but the imbalanced dataset in real industrial scenarios will seriously affect the performance of various popular deep learning models. Data generation-based strategy provides a solution by expanding the number of minority samples. However, many data-generation methods cannot generate high-quality samples when the imbalanced ratio is high. To address these problems, a dual-attention feature fusion network (DAFFN) with two-stream hybrid-generated data is proposed. First, the two-stream hybrid generator including a generative model and an oversampling technique is adopted to generate minority fault data. Then, the convolutional neural network is used to extract features from hybrid-generated data. In particular, a feature fusion network with a dual-attention mechanism, i.e., a channel attention mechanism and a layer attention mechanism are designed to learn channel-level and layer-level weights of the features. Extensive results on two bearing datasets indicate that the proposed framework achieves outstanding performance in various high imbalanced-ratio cases.
更多查看译文
关键词
Fault diagnosis,Imbalanced dataset,Hybrid generated data,Dual-attention mechanism,Feature fusion network
AI 理解论文
溯源树
样例
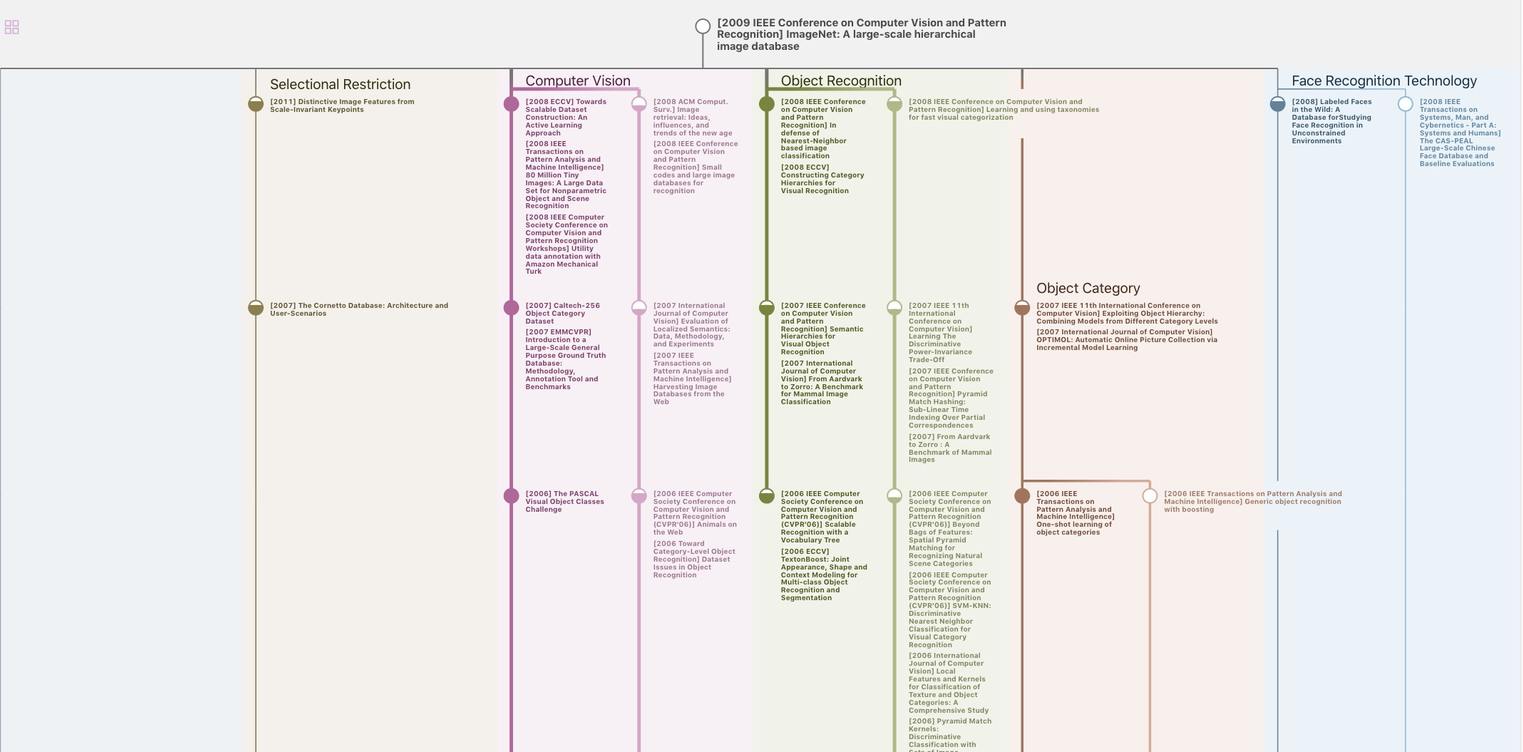
生成溯源树,研究论文发展脉络
Chat Paper
正在生成论文摘要