An expert knowledge-empowered CNN approach for welding radiographic image recognition
ADVANCED ENGINEERING INFORMATICS(2023)
摘要
Non-destructive testing of welds based on the radiographic image is crucial for improving the reliability of aerospace structural components. The deep learning method represented by the convolutional neural network (CNN) has received extensive attention in welding radiographic image recognition (WRIR) owing to its powerful feature adaptive extraction ability. However, CNN-based WRIR faces key challenges of small sample size and poor explainability. Inspired by the process of interpreting radiographic film by experts, expert knowledgeempowered CNN for WRIR is proposed. Two self-supervised learning (SSL) tasks for radiographic image deblurring and brightness adjustment are designed to model expert experience. The expert knowledge learned from the SSL process is used to guide the CNN to identify weld defects. The results show that the proposed method improves the inductive bias of the CNN model, owns a faster convergence speed and recognition accuracy under the condition of small sample size, and reaches 97.65% of the comprehensive evaluation index F1score. Moreover, the expert knowledge learned from the SSL process and the decision-making basis of the CNN model are visualized from both global and local aspects, which improve the explainability of CNN-based WRIR.
更多查看译文
关键词
cnn approach,recognition,image,knowledge-empowered
AI 理解论文
溯源树
样例
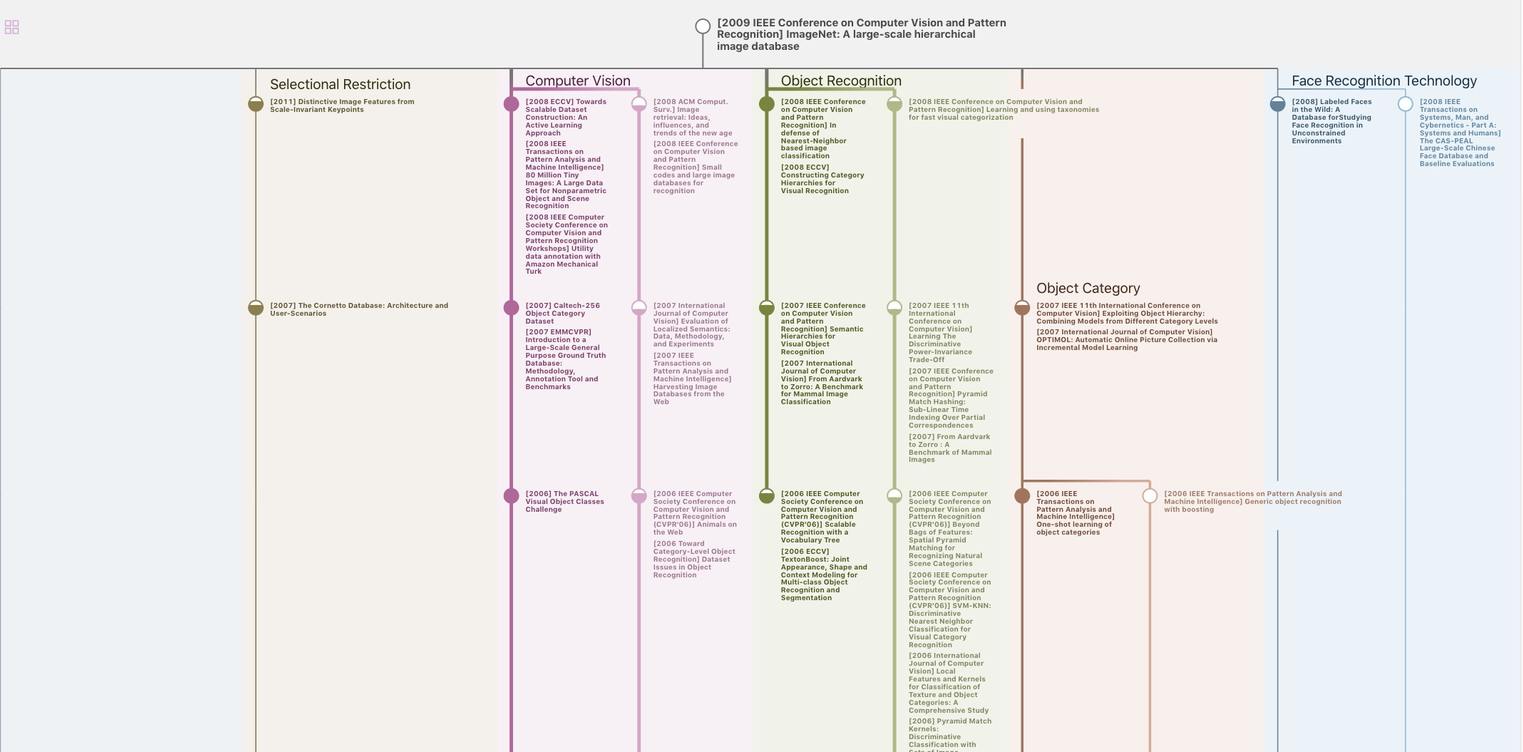
生成溯源树,研究论文发展脉络
Chat Paper
正在生成论文摘要