Automated Assessment of Cardiac Systolic Function From Coronary Angiograms With Video-Based Artificial Intelligence Algorithms.
JAMA CARDIOLOGY(2023)
摘要
Importance:Understanding left ventricular ejection fraction (LVEF) during coronary angiography can assist in disease management.
Objective:To develop an automated approach to predict LVEF from left coronary angiograms.
Design, Setting, and Participants:This was a cross-sectional study with external validation using patient data from December 12, 2012, to December 31, 2019, from the University of California, San Francisco (UCSF). Data were randomly split into training, development, and test data sets. External validation data were obtained from the University of Ottawa Heart Institute. Included in the analysis were all patients 18 years or older who received a coronary angiogram and transthoracic echocardiogram (TTE) within 3 months before or 1 month after the angiogram.
Exposure:A video-based deep neural network (DNN) called CathEF was used to discriminate (binary) reduced LVEF (≤40%) and to predict (continuous) LVEF percentage from standard angiogram videos of the left coronary artery. Guided class-discriminative gradient class activation mapping (GradCAM) was applied to visualize pixels in angiograms that contributed most to DNN LVEF prediction.
Results:A total of 4042 adult angiograms with corresponding TTE LVEF from 3679 UCSF patients were included in the analysis. Mean (SD) patient age was 64.3 (13.3) years, and 2212 patients were male (65%). In the UCSF test data set (n = 813), the video-based DNN discriminated (binary) reduced LVEF (≤40%) with an area under the receiver operating characteristic curve (AUROC) of 0.911 (95% CI, 0.887-0.934); diagnostic odds ratio for reduced LVEF was 22.7 (95% CI, 14.0-37.0). DNN-predicted continuous LVEF had a mean absolute error (MAE) of 8.5% (95% CI, 8.1%-9.0%) compared with TTE LVEF. Although DNN-predicted continuous LVEF differed 5% or less compared with TTE LVEF in 38.0% (309 of 813) of test data set studies, differences greater than 15% were observed in 15.2% (124 of 813). In external validation (n = 776), video-based DNN discriminated (binary) reduced LVEF (≤40%) with an AUROC of 0.906 (95% CI, 0.881-0.931), and DNN-predicted continuous LVEF had an MAE of 7.0% (95% CI, 6.6%-7.4%). Video-based DNN tended to overestimate low LVEFs and underestimate high LVEFs. Video-based DNN performance was consistent across sex, body mass index, low estimated glomerular filtration rate (≤45), presence of acute coronary syndromes, obstructive coronary artery disease, and left ventricular hypertrophy.
Conclusion and relevance:This cross-sectional study represents an early demonstration of estimating LVEF from standard angiogram videos of the left coronary artery using video-based DNNs. Further research can improve accuracy and reduce the variability of DNNs to maximize their clinical utility.
更多查看译文
关键词
coronary angiograms,cardiac systolic function,artificial intelligence,video-based
AI 理解论文
溯源树
样例
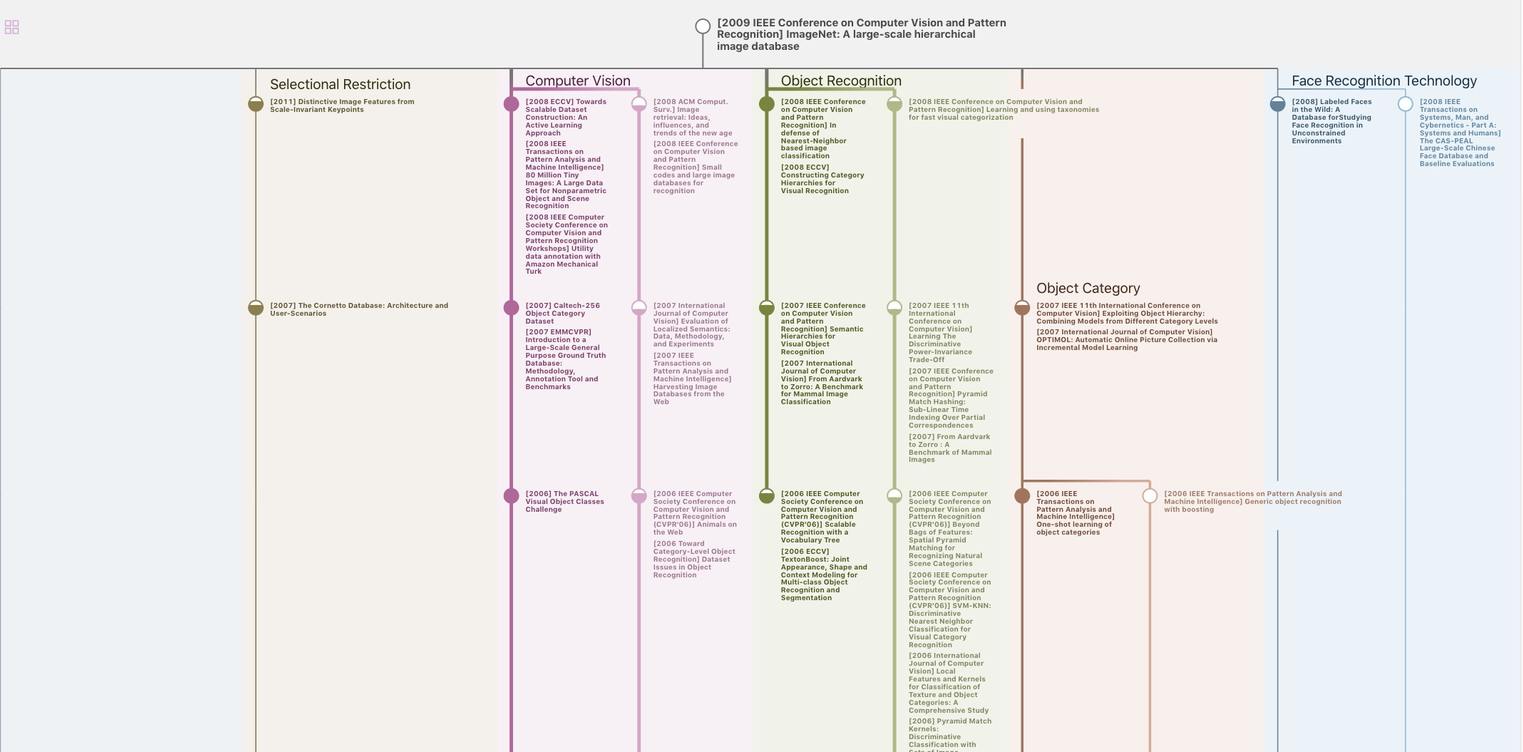
生成溯源树,研究论文发展脉络
Chat Paper
正在生成论文摘要