Machine learning methods for the detection of explosives, drugs and precursor chemicals gathered using a colorimetric sniffer sensor
ANALYTICAL METHODS(2023)
摘要
Colorimetric sensing technology for the detection of explosives, drugs, and their precursor chemicals is an important and effective approach. In this work, we use various machine learning models to detect these substances from colorimetric sensing experiments conducted in controlled environments. The detection experiments based on the response of a colorimetric chip containing 26 chemo-responsive dyes indicate that homemade explosives (HMEs) such as hexamethylene triperoxide diamine (HMTD), triacetone triperoxide (TATP), and methyl ethyl ketone peroxide (MEKP) used in improvised explosives devices are detected with true positive rate (TPR) of 70-75%, 73-90% and 60-82% respectively. Time series classifiers such as Convolutional Neural Networks (CNN) are explored, and the results indicate that improvements can be achieved with the use of kinetics of the chemical responses. The use of CNNs is limited, however, to scenarios where a large number of measurements, typically in the range of a few hundred, of each analyte are available. Feature selection of important dyes using the Group Lasso (GPLASSO) algorithm indicated that certain dyes are more important in discrimination of an analyte from ambient air. This information could be used for optimizing the colorimetric sensor and extend the detection to more analytes.
更多查看译文
关键词
explosives,detection,machine learning methods,machine learning,precursor chemicals
AI 理解论文
溯源树
样例
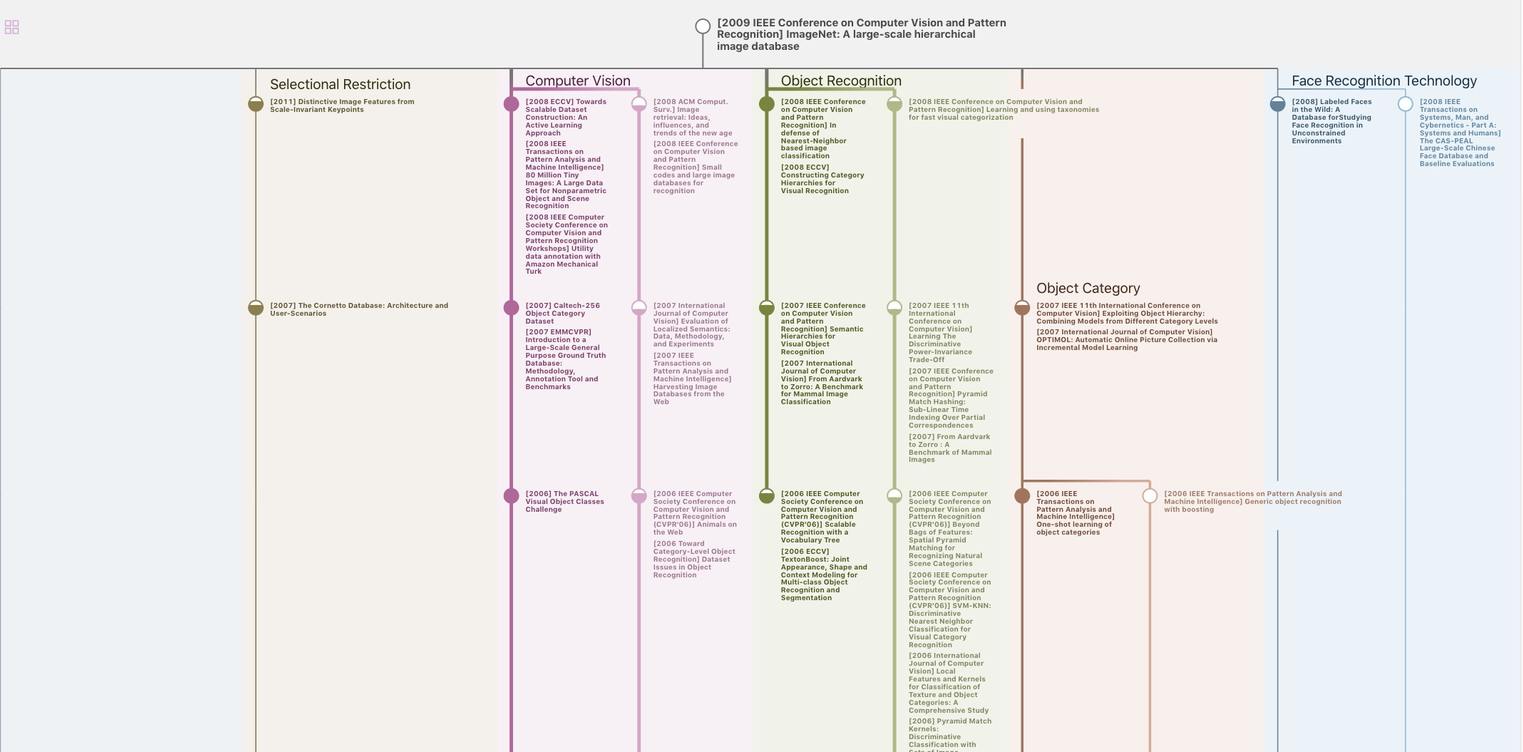
生成溯源树,研究论文发展脉络
Chat Paper
正在生成论文摘要