ScalaQC: a scalability optimization framework for full-state quantum simulation on CPU plus GPU heterogeneous clusters
CCF TRANSACTIONS ON HIGH PERFORMANCE COMPUTING(2023)
摘要
Quantum simulation on classical computers is one of the main approaches to evaluate quantum computation devices and develop quantum algorithms. Some quantum simulators have been proposed, mainly divided into two categories: full-state simulators and tensor network simulators. The former consumes a lot of memory to hold the quantum state vectors. Therefore, the time overhead cost by calculation is much lower than that cost by memory access and communication. Traditional optimization techniques such as latency hiding are not suitable for quantum simulation, and high-performance devices like GPGPUs cannot be fully utilized. This paper proposes ScalaQC optimizer to perform data locality and data layout optimizations, which can use CPU memory to scale the simulation, and reduce the data communication overhead between multiple nodes. We evaluate ScalaQC on a small-scale CPU+GPU cluster for 30-35 qubits. Theoretically, our optimizations will be more effective as the number of qubits increases.
更多查看译文
关键词
Quantum Circuit Simulation, Multi-GPU, Heterogeneous CPU plus GPU
AI 理解论文
溯源树
样例
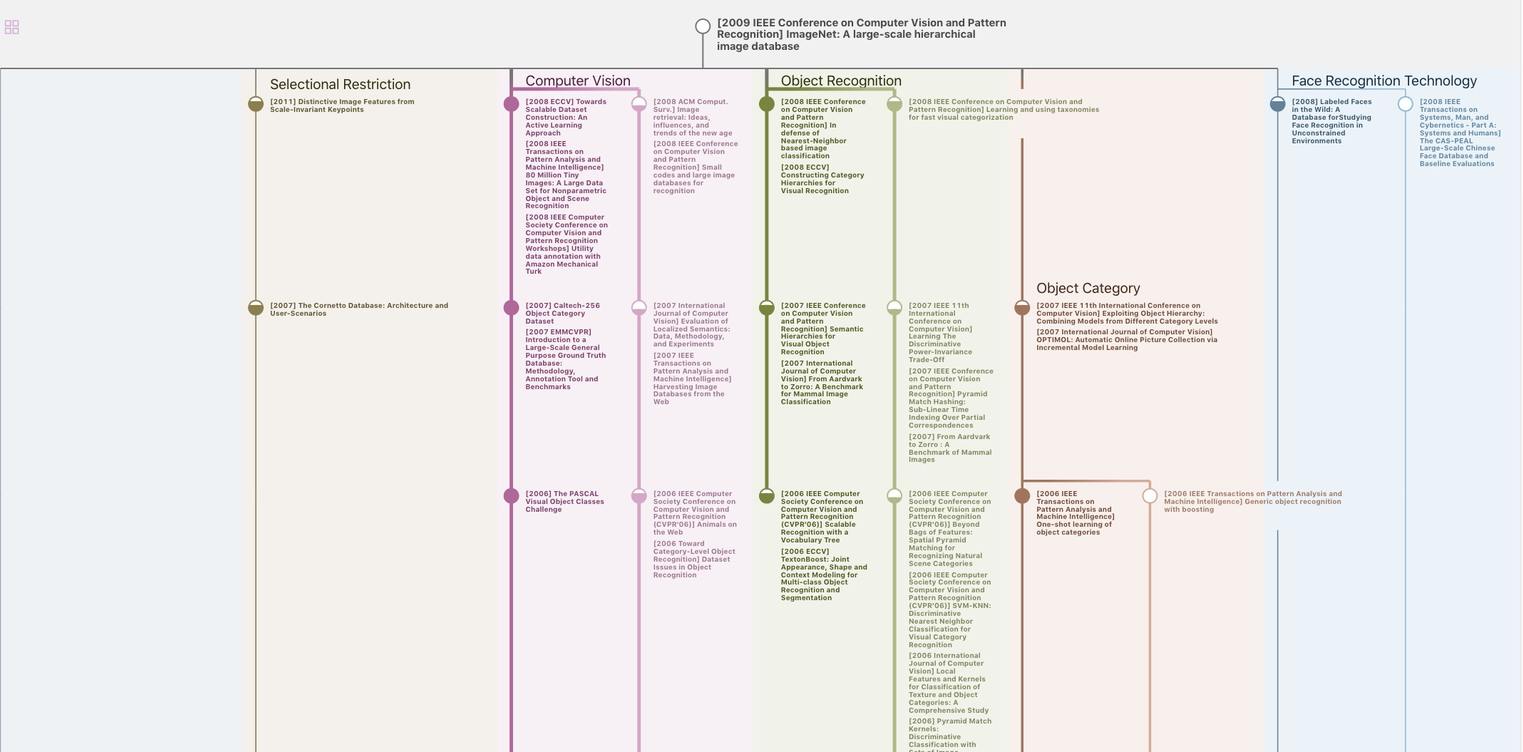
生成溯源树,研究论文发展脉络
Chat Paper
正在生成论文摘要