cFEM: a cluster based feature extraction method for network intrusion detection
INTERNATIONAL JOURNAL OF INFORMATION SECURITY(2023)
摘要
The recent trend in network intrusion detection leverages key features of machine learning (ML) algorithms to detect network traffic anomalies. Network traffic flows contain high dimensional features which significantly affect data-driven approaches. Therefore, the performance of ML-based approaches mainly depends on the appropriate set of features of network data. Different feature selection and extraction methods are extensively employed to attain the informative and compact set of features. Existing methods often suffer from achieving the expected performance due to the lacking of effectively removing redundant features as well as incorporating features with complementary information. In this paper, we present a cluster-based feature extraction method using Mahalanobis distance (cFEM) that clusters the correlated features and extracts new feature representations based on a distance metric. The extracted features on the transformed dimensions are employed to train different machine learning classifiers. We conducted extensive experiments using three renowned datasets. The results show that cFEM outperforms the state-of-the-art intrusion detection methods in several performance metrics such as detection rate (99.61%) and false alarm rate (0.26%). Further experiments on extracted features show that our extracted features are discriminative, free of redundancy, and able to capture complementary information.
更多查看译文
关键词
Anomaly detection,Clustering,Feature extraction,Mahalanobis distance
AI 理解论文
溯源树
样例
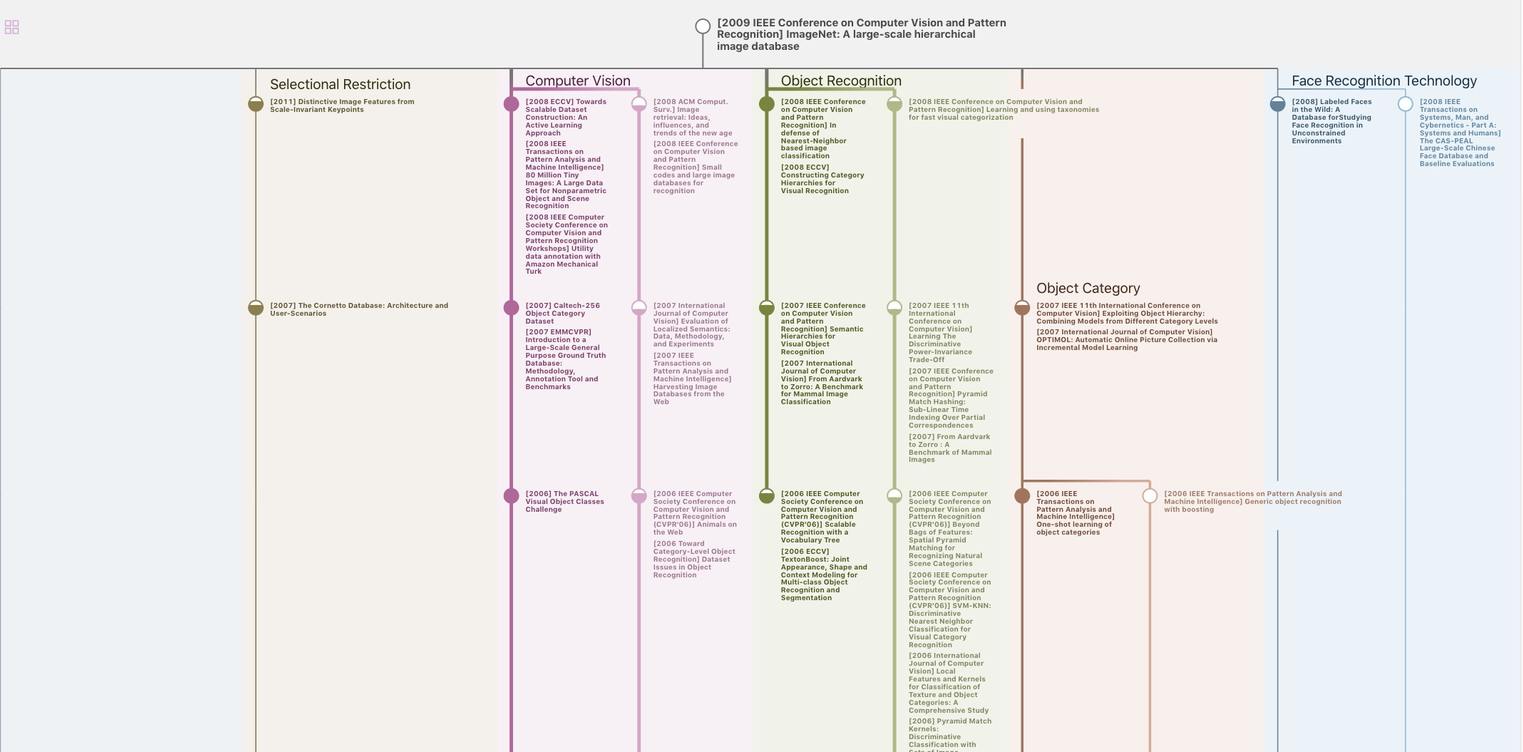
生成溯源树,研究论文发展脉络
Chat Paper
正在生成论文摘要