Towards automatic Privacy-Preserving Record Linkage: A Transfer Learning based classification step
DATA & KNOWLEDGE ENGINEERING(2023)
摘要
Privacy-Preserving Record Linkage (PPRL) intends to identify records that match the same real-world entities across disparate data sources while preserving the privacy of the individual entities. To identify matching records across different data sources and still preserve the privacy of the information, PPRL needs to consider several restrictions due to privacy limitations. For instance, PPRL is executed over anonymized (or encrypted) data to avoid re-identification. Moreover, the classification step of PPRL does not have access to labeled information (indicating if a pair of records is a match) and an oracle (specialist) to label a few instances. These limitations make it hard to employ automatic classification techniques. Most PPRL techniques use a simple threshold (defined by a specialist) to define whether a pair of records represent the same real-world entity or not. To overcome these problems, we present a Transfer Learning -based unsupervised classification step to PPRL, which leverages the information available in public (or synthetic) datasets to train accurate classifiers in a privacy-preserving context. We evaluate our approach using real-world and synthetic data, and the results demonstrate that our unsupervised classification step is able to overcome the most used classification strategies in PPRL.
更多查看译文
关键词
Entity resolution, Machine learning, Domain adaptation, Data privacy
AI 理解论文
溯源树
样例
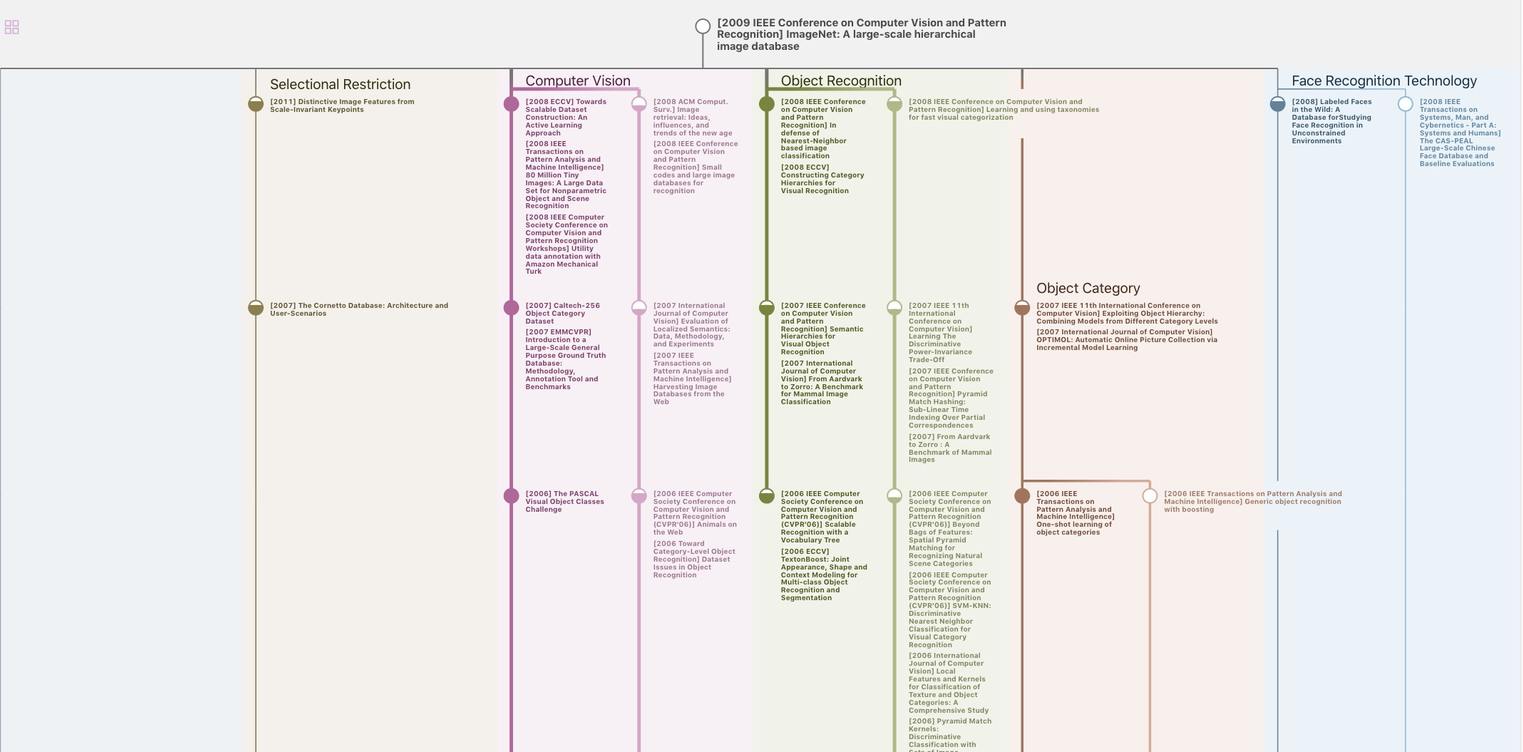
生成溯源树,研究论文发展脉络
Chat Paper
正在生成论文摘要