Edge-featured graph attention network with dependency features for causality detection of events
EXPERT SYSTEMS(2023)
摘要
Causality detection, as a more fine-grained task than causality extraction, which aims to detect the components that represent the cause and effect in sentence-level texts with causality, is a significant task in the field of Natural Language Processing (NLP). Previous research on causality detection has concentrated on text token features whilst ignoring the dependency attributes between tokens. In this paper, we propose a model that uses the Edge-featured Graph Attention Network based on dependency-directed graphs for the causality detection task. To begin, we convert the texts with causality into the representation of dependency-directed graphs (DDG), which regard the dependency attributes between tokens as edge features. Then we use Edge-featured Graph Attention Network to aggregate the node and edge features of DDG. Finally, we put the graph embedding into Bi-directional Long Short-Term Memory (BiLSTM) layer to learn the dependencies between forward and backward long-distance nodes in DDG. Experiments on three datasets prove that this method achieves better performance in precision, recall, and other evaluation metrics compared with other methods.
更多查看译文
关键词
graph attention network,causality detection,dependency features,events
AI 理解论文
溯源树
样例
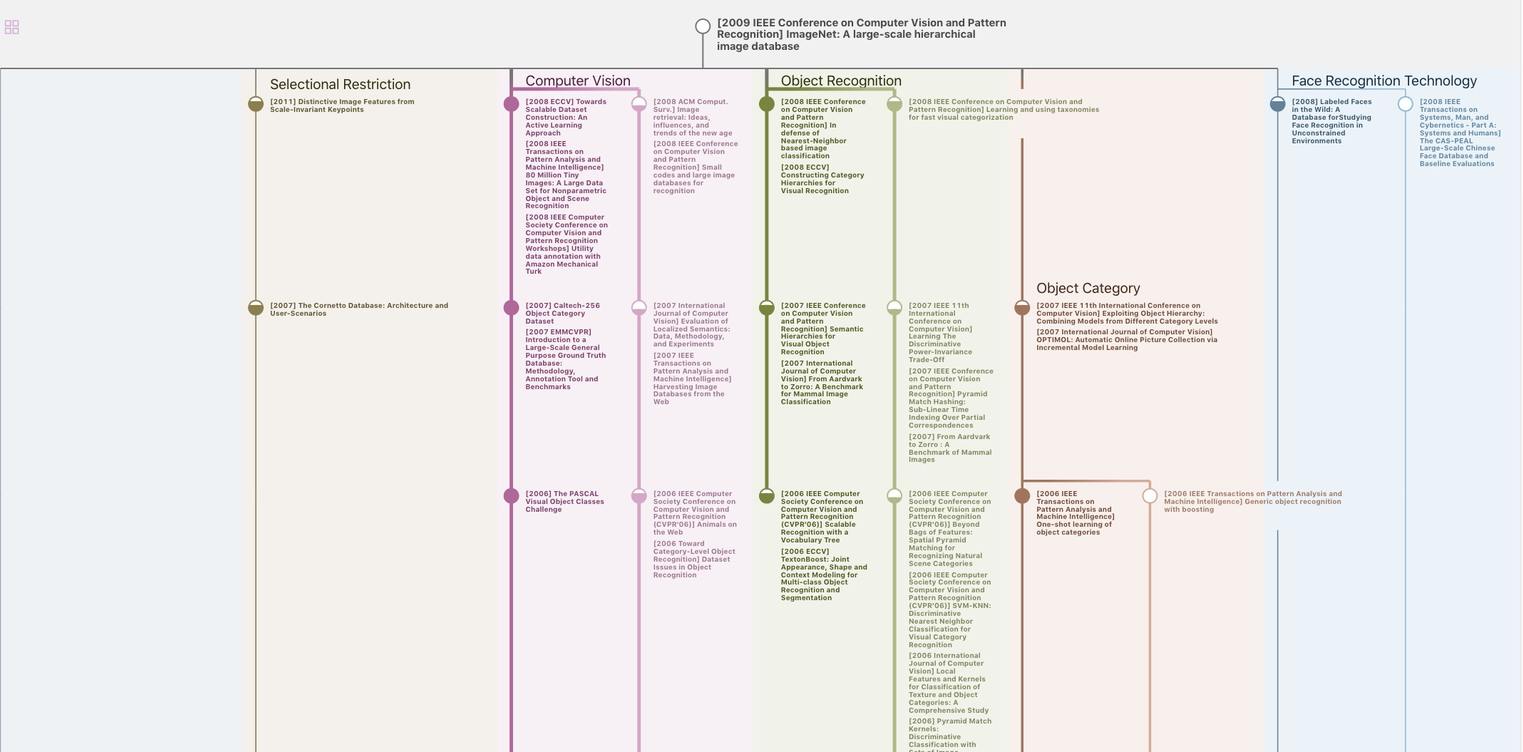
生成溯源树,研究论文发展脉络
Chat Paper
正在生成论文摘要