TMPF: A Two-Stage Merging Planning Framework for Dense Traffic.
ADVANCED INTELLIGENT SYSTEMS(2023)
摘要
Planning for autonomous vehicles to merge into high-density traffic flows within limited mileage is quite challenging. Specifically, the driving trajectory will inevitably have intersections with other vehicles whose driving intentions can't be directly observed. Herein, a two-stage algorithm framework that is decomposed into the longitudinal and lateral planning processes for online merging planning is proposed. An improved particle filter is used to estimate the driving models of surrounding vehicles for predicting their future driving intentions. Based on Monte Carlo tree search (MCTS), different action spaces are evaluated for longitudinal merging gap selection and lateral interactive merging operation, while heuristic pruning is used to reduce the computation cost. Moreover, the coefficients related to the driving styles are introduced, and their influences on merging performance are analyzed. Finally, the proposed algorithm is implemented in a two-lane simulation environment. The results show that the proposal has outperformed other baseline methods.
更多查看译文
关键词
autonomous vehicles,decision-making,Monte Carlo tree search,particle filters
AI 理解论文
溯源树
样例
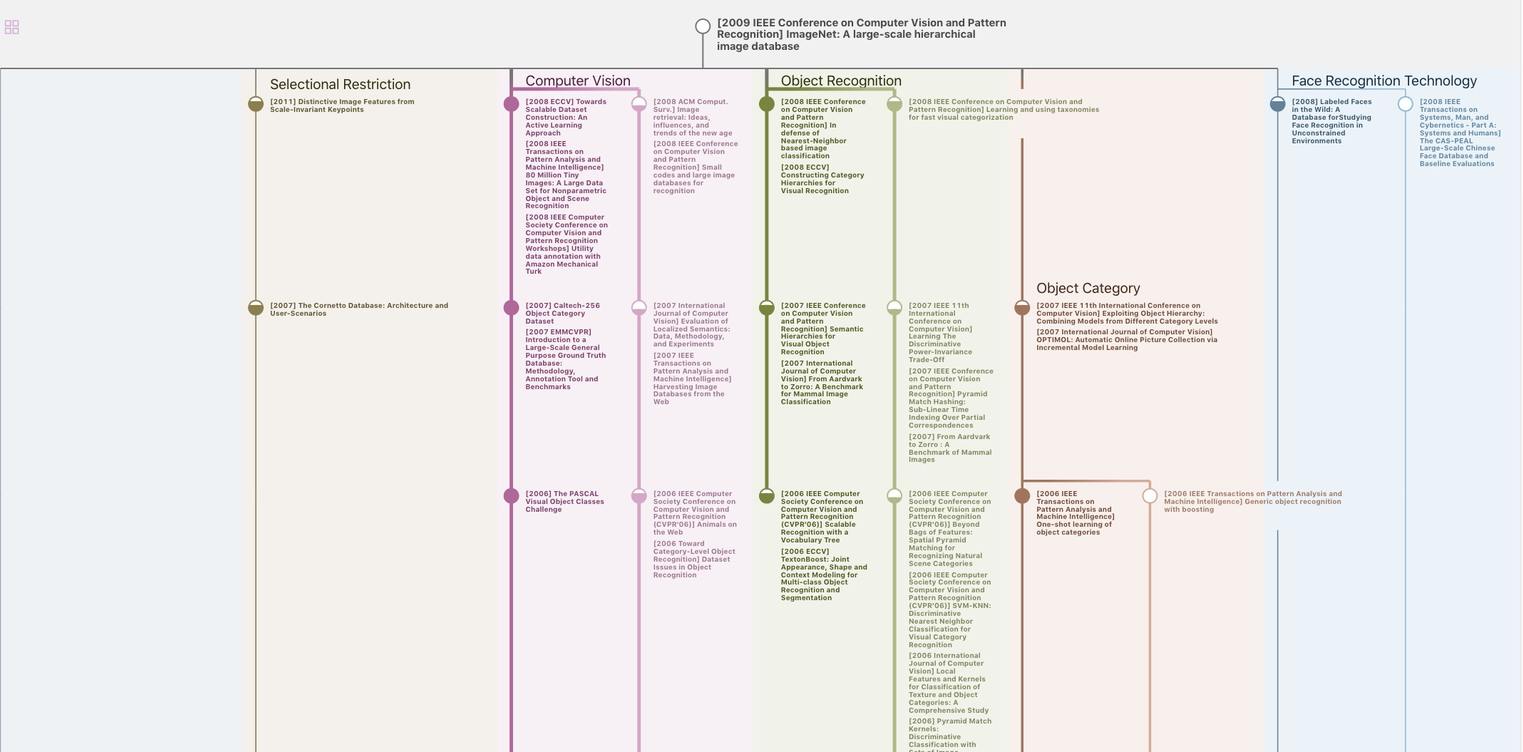
生成溯源树,研究论文发展脉络
Chat Paper
正在生成论文摘要