Designing a fault detection classifier framework for an industrial dynamic ultrafiltration membrane process using wavelet-based feature analysis
PROCESS SAFETY AND ENVIRONMENTAL PROTECTION(2023)
摘要
In recent years, due to the increase in population and the number of industrial sites, water reclaim has become more relevant. Dynamic membrane filtration systems can play an important role for water reuse. Therefore, process monitoring of dynamic membrane systems is of great importance to ensure the water quality while considering environmental and economic factors. The exploitation of on-line monitoring of dynamic membrane systems in industrial operation is challenged by intentional disturbances such as backflush and backshock (i.e. used as cleaning strategies) and unintentional input fluctuations. Consequently, process inherent behaviour is hidden within very complex sensors signals. Therefore, critical flux identification or fouling characterization for process monitoring is not straightforward, leading to suboptimal operation. The present study establishes a fault detection framework from a classification viewpoint, capable of handling industrial data that is prone to noise and disturbances, while offering an effective yet straightforward approach. The case study is a pilot-scale dynamic ultrafiltration process which has been tested previously within an industrial facility for produced water reclamation. The dataset contains 18 experiments, where three of the experiments are faulty. These experiments were implemented in an oil recovery facility located in the Orinoquia region, Colombia. A feature analysis approach based on wavelets is developed to identify the key characteristics of the installed sensors while alleviating the noise effects. The process conditions are pinpointed by feeding the extracted features into several widely used classification methods including Multilayer Perceptron Neural Network (MLPNN), Support Vector Machine (SVM), and Principal Component Analysis (PCA) based classifiers. The results indicated that the MLPNN classifier has the highest detection accuracy of 99.7% with a low percentage of false alarms. The framework developed in this study is a vital part of a membrane system digitalization strategy, which can be integrated into automated surveillance strategies for monitoring of membrane systems toward effective fault detection.
更多查看译文
关键词
Fault detection,Dynamic ultrafiltration,Produced water management,Wavelets analysis,Classification
AI 理解论文
溯源树
样例
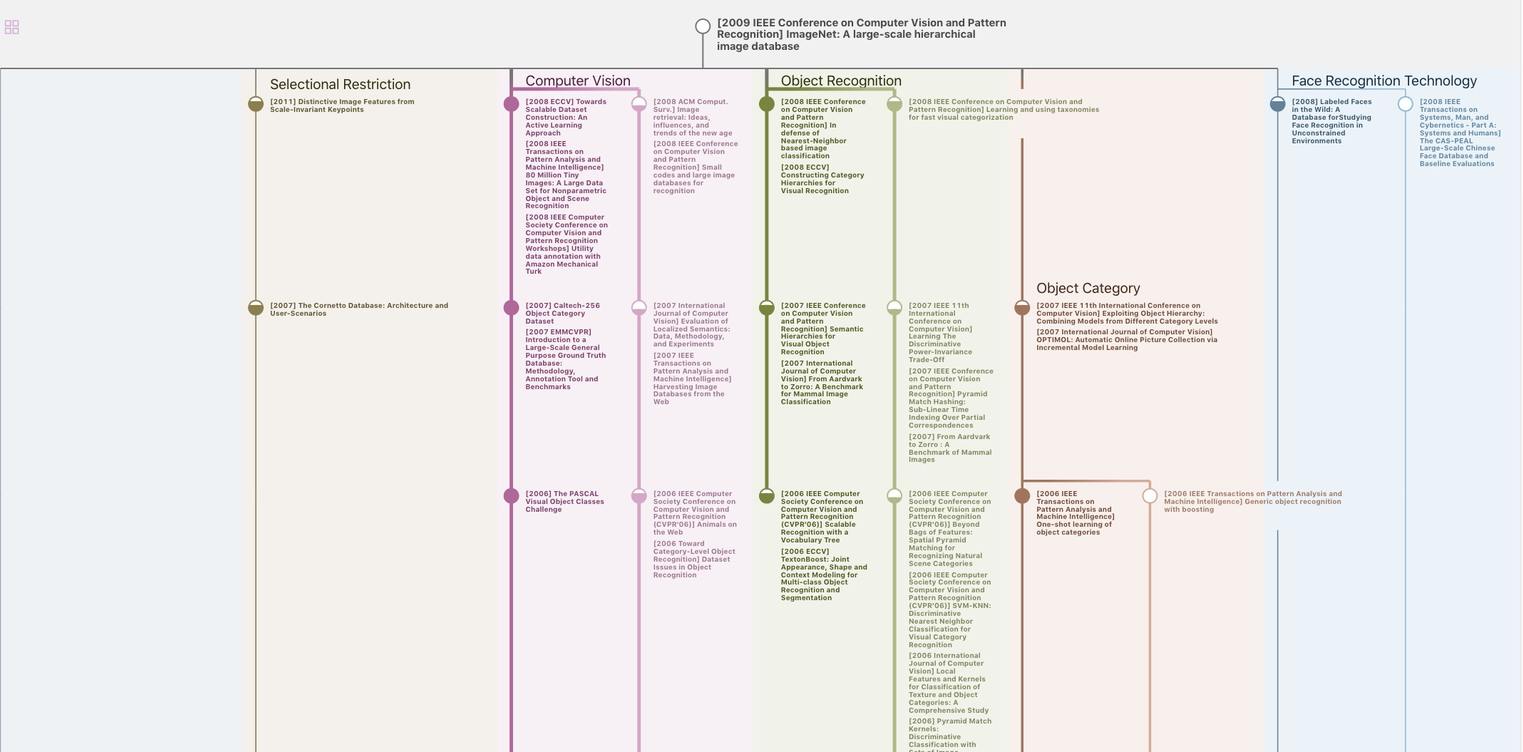
生成溯源树,研究论文发展脉络
Chat Paper
正在生成论文摘要