Discovery of High-Performing Metal-Organic Frameworks for Efficient SF6/N2 Separation: A Combined Computational Screening, Machine Learning, and Experimental Study
INDUSTRIAL & ENGINEERING CHEMISTRY RESEARCH(2023)
摘要
Effective capture and recovery of sulfur hexafluoride (SF6) from SF6/N2 mixture is an urgent challenge. Considering the existence of a large number of metal- organic frameworks (MOFs), the computational screening of MOFs is strongly desired before experimental efforts. In this work, the top-performance MOF adsorbents were identified from the most recent computation-ready, experimental metal-organic frameworks (CoRE MOFs) based on various metrics. The degree of unsaturation (unsat) and the number of hydrogen per unit cell (H) revealed with the optimal machine learning (ML) model are important factors for effective SF6/N2 separation. One of the screened MOF candidates, FIRNAX01(TKL-107), was synthesized and the separation performance exceeded all the reported MOFs. Our computational screening not only offers effective prediction but also paves the way for accelerating the development of novel MOFs.
更多查看译文
AI 理解论文
溯源树
样例
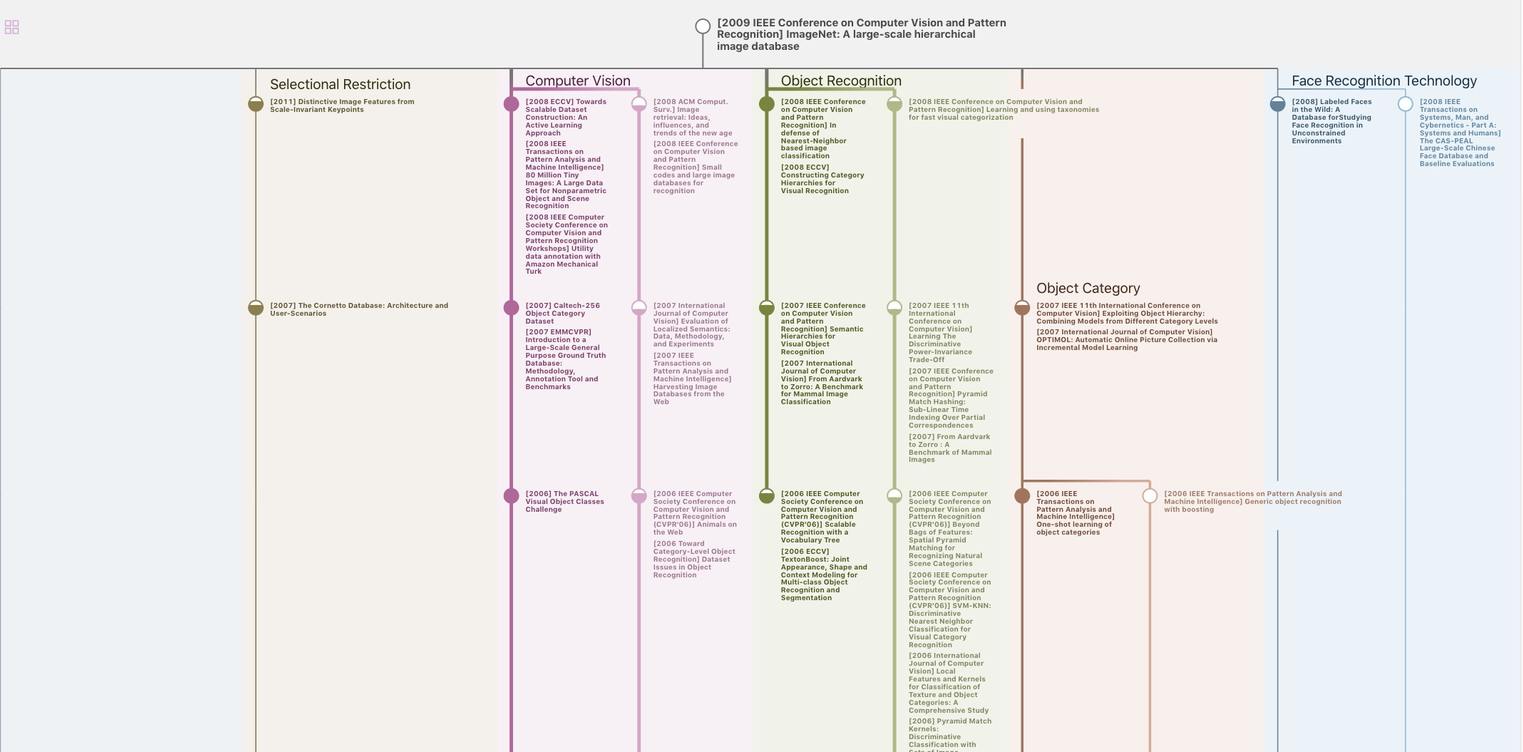
生成溯源树,研究论文发展脉络
Chat Paper
正在生成论文摘要