Artificial enabled communications in optimization for transitive energy framework using maximum probability distribution
SOFT COMPUTING(2023)
摘要
In big power systems, the distribution transformer plays a key role in determining the power flow. Higher power system efficiency and improved power transfer capacity are implied by their improved performance. However, numerous distribution transformer failures in the recent past have disrupted the power supply and attracted a lot of interest from electrical experts. This research proposes novel technique in optimization of distribution transformer based on maximum probability in load with fault assessment based on artificial enabled communications in optimization for transactive energy framework using Nissan Leaf BEVs with a 24-kWh battery capacity which were utilized in the test system. Here, the load distribution of transformer has been carried out using maximum probability, and load distribution-based fault detection is carried out using regressive reinforcement Boltzmann neural network. Additionally, it is shown how the load distribution affects the needed amplitude of the second harmonic component of the circulating currents, which can be used to assure balancing capabilities within each MMC arm. Experimental analysis has been carried out in terms of load balancing, RMSE, MAPE, reliability, energy storage, and voltage drop. The proposed technique attained load balancing of 77%, RMSE of 69%, MAPE of 77%, reliability of 93%, energy storage of 97%, and voltage drop of 96%.
更多查看译文
关键词
Distribution transformer,Transactive energy framework,Maximum probability,Artificial enabled communications
AI 理解论文
溯源树
样例
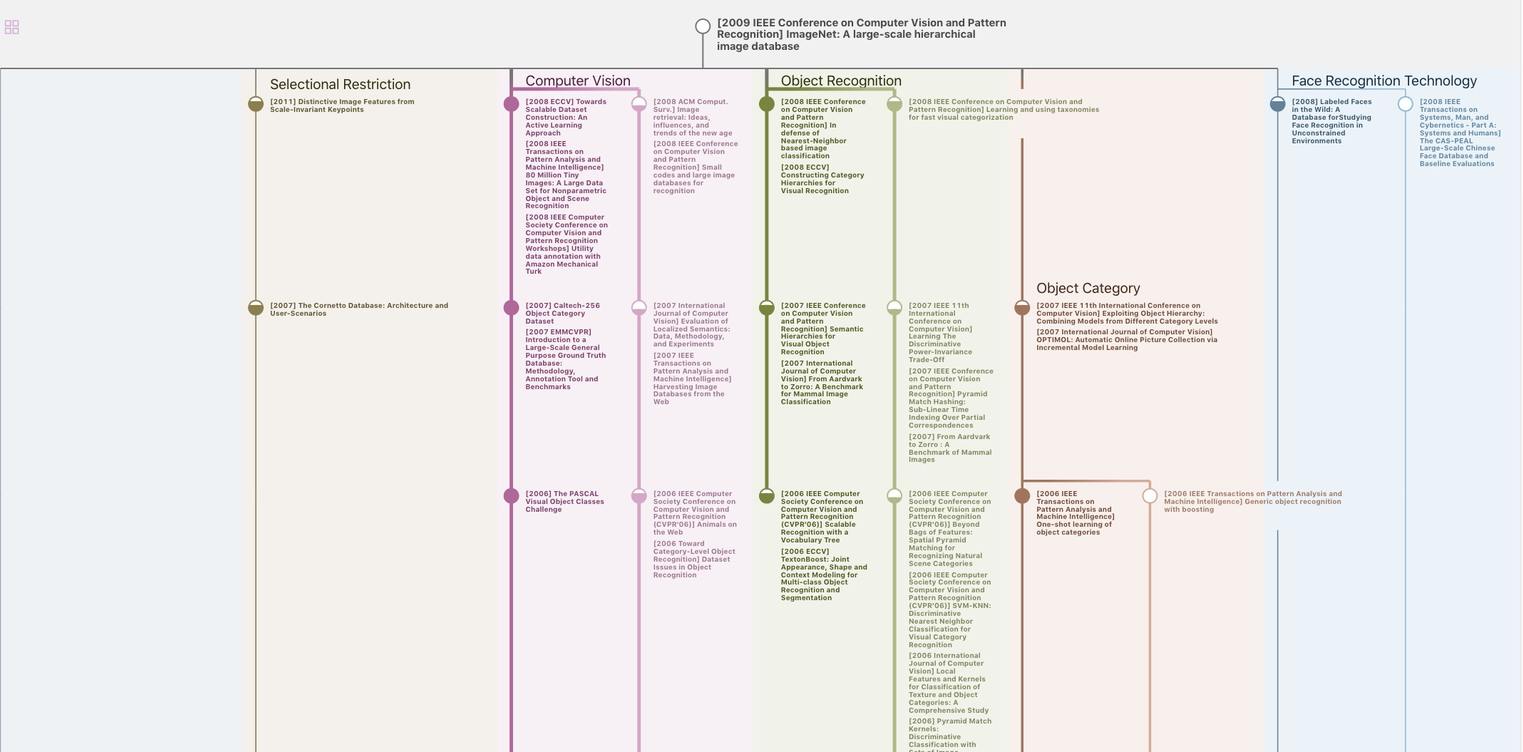
生成溯源树,研究论文发展脉络
Chat Paper
正在生成论文摘要