Guided Dropout: Improving Deep Networks Without Increased Computation
INTELLIGENT AUTOMATION AND SOFT COMPUTING(2023)
摘要
Deep convolution neural networks are going deeper and deeper. However, the complexity of models is prone to overfitting in training. Dropout, one of the crucial tricks, prevents units from co-adapting too much by randomly dropping neurons during training. It effectively improves the performance of deep networks but ignores the importance of the differences between neurons. To optimize this issue, this paper presents a new dropout method called guided dropout, which selects the neurons to switch off according to the differences between the convolution kernel and preserves the informative neurons. It uses an unsupervised clustering algorithm to cluster similar neurons in each hidden layer, and dropout uses a certain probability within each cluster. Thereby this would preserve the hidden layer neurons with different roles while maintaining the model's scarcity and generalization, which effectively improves the role of the hidden layer neurons in learning the features. We evaluated our approach compared with two standard dropout networks on three well-established public object detection datasets. Experimental results on multiple datasets show that the method proposed in this paper has been improved on false positives, precision-recall curve and average precision without increasing the amount of computation. It can be seen that the increased performance of guided dropout is thanks to shallow learning in the networks. The concept of guided dropout would be beneficial to the other vision tasks.
更多查看译文
关键词
deep networks
AI 理解论文
溯源树
样例
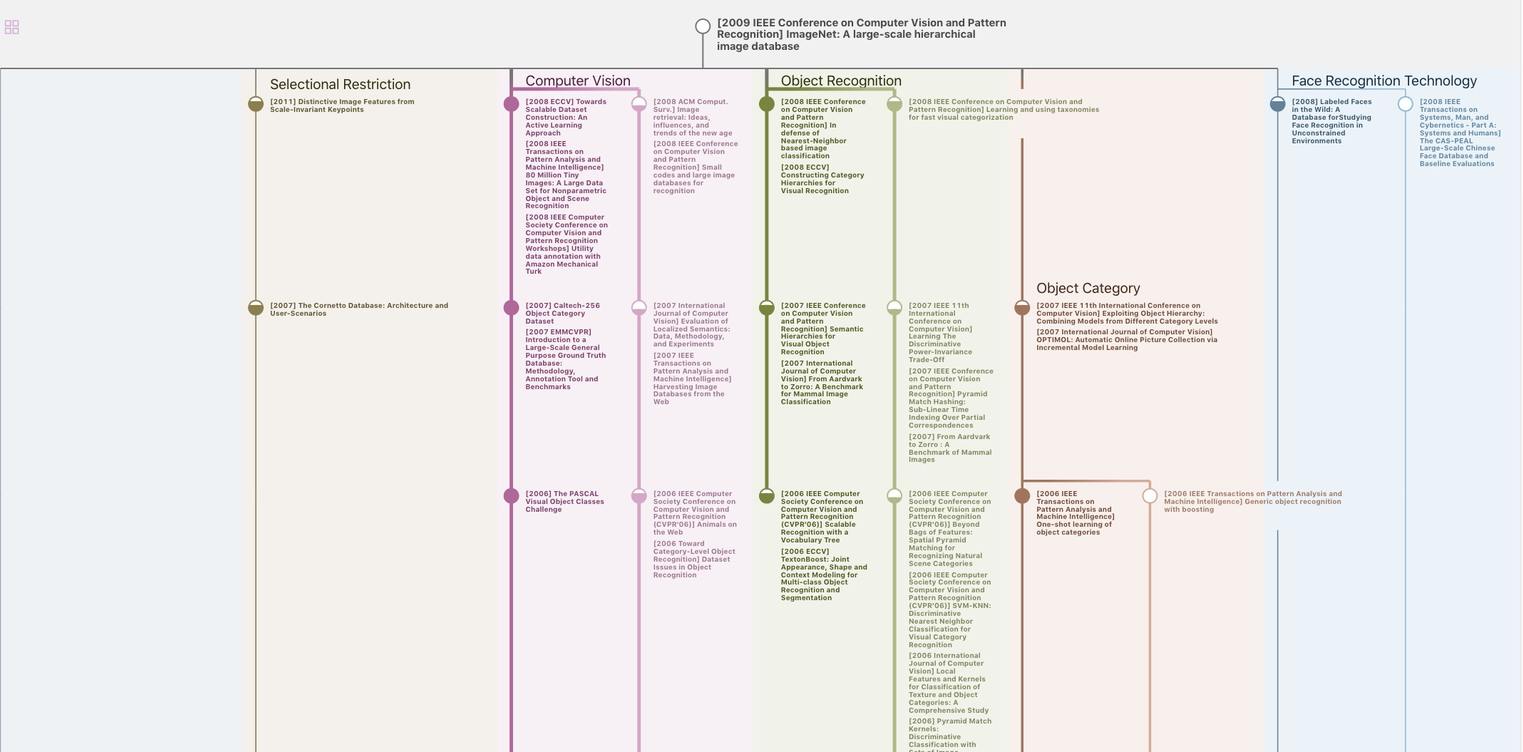
生成溯源树,研究论文发展脉络
Chat Paper
正在生成论文摘要