Correlation embedding learning with dynamic semantic enhanced sampling for knowledge graph completion
WORLD WIDE WEB-INTERNET AND WEB INFORMATION SYSTEMS(2023)
摘要
Knowledge graph completion aims to solve the problem of incompleteness and sparsity in knowledge graphs. However, the negative sampling strategy in current completion methods samples entities with equal probability and cannot guarantee the quality of negative samples, causing gradient disappearance during the training process. In addition, existing embedding learning methods ignore the diversity and complexity of the entities and relations in a knowledge graph. Therefore, we design a dynamic semantic sampling and correlation embedding completion framework that includes a negative sampling algorithm based on dynamic semantic similarity and a correlation embedding model. The negative-sampling algorithm can gradually explore high-quality negative samples to participate in model training. The embedding model can enrich embeddings by learning the sequential and correlated information of entities and relations in the knowledge graph. Finally, we conducted experiments on two public datasets, and the experimental results proved the performance of our method.
更多查看译文
关键词
Knowledge graph,Knowledge graph completion,Dynamic negative sampling,Sequence-correlated embedding learning
AI 理解论文
溯源树
样例
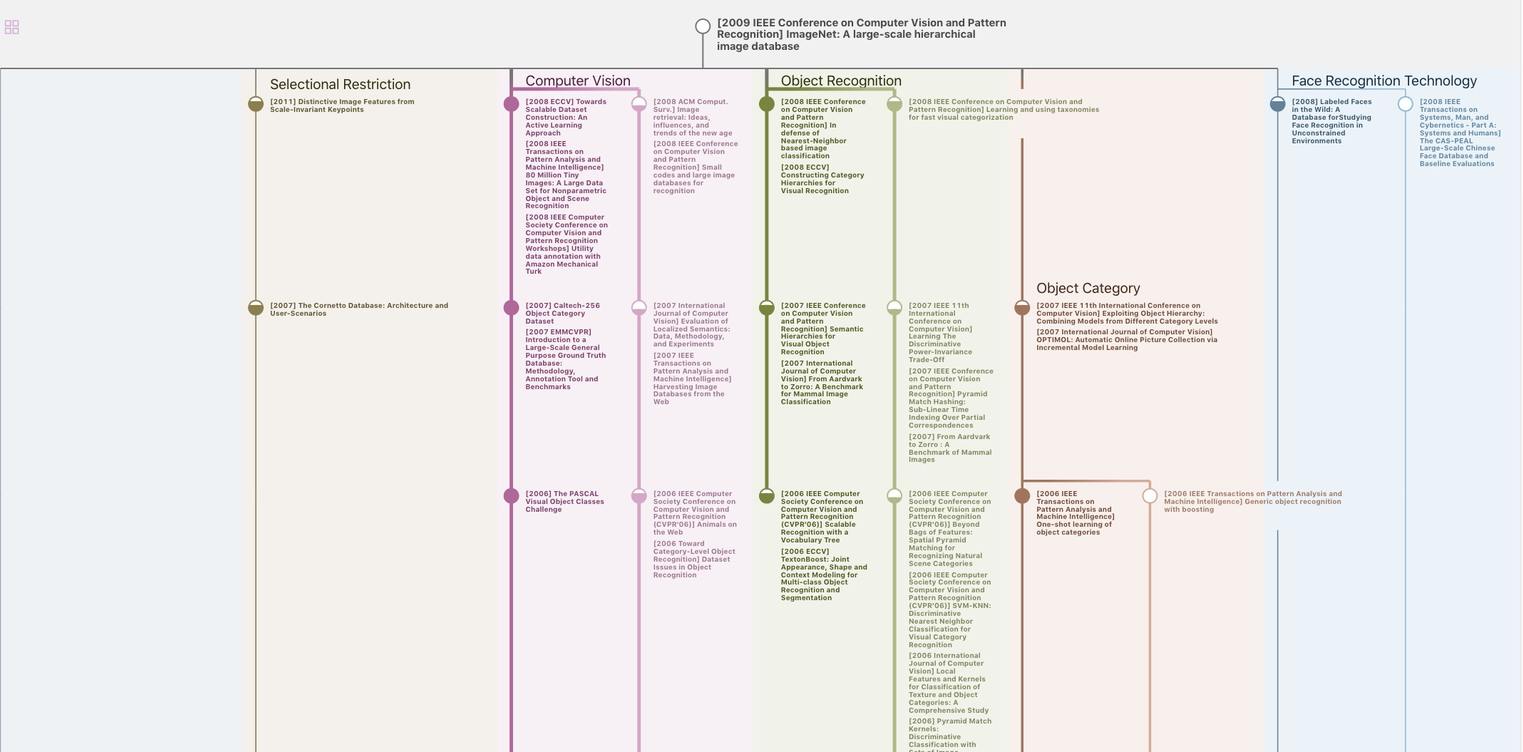
生成溯源树,研究论文发展脉络
Chat Paper
正在生成论文摘要