Building detection algorithm in multi-scale remote sensing images based on attention mechanism
EVOLUTIONARY INTELLIGENCE(2023)
摘要
The seismic fortification intensity requirements of buildings of various heights are different. Identifying the heights of different buildings using a specific method can reduce secondary disasters such as building damage and house collapse caused by destructive earthquakes. The traditional building object detection model uses the feature expression of the dataset based on manually sketching the shape of the building in the remote sensing images, which will cause less acquired image information, unclear target features of buildings, and the decrease of target detection accuracy of identifying building types. The real-time target detection algorithm YOLO has fast detection speed and high accuracy, but its main disadvantage is the inaccurate positioning of the building bounding box and the misjudgment of partially overlapping building objects. Image feature retrieval using large data set domain adaptive fast algorithm is a new research hotspot in current image recognition. This research proposes a multi-branch network with the addition of a module using attention mechanism to coordinate the salient and sub-salient information of images. The algorithm adds channel and spatial attention mechanisms to the feature extraction network, and uses weighting and filtering methods to perform residual fusion on the original features vectors in images. The model is deployed on the remote sensing geographic information evaluation platform to conduct various building target detection experiments. The average detection accuracy of the urban buildings can reach 81.8%, and the detection speed for remote sensing segmentation images is 26 per second. The mAP@0.5 of the YOLOv5 algorithm with the attention mechanism is 12% higher than that of the unimproved YOLOv5 algorithm on the urban street view image dataset.
更多查看译文
关键词
YOLO V5, Remote sensing image, Attention mechanism, Building detection
AI 理解论文
溯源树
样例
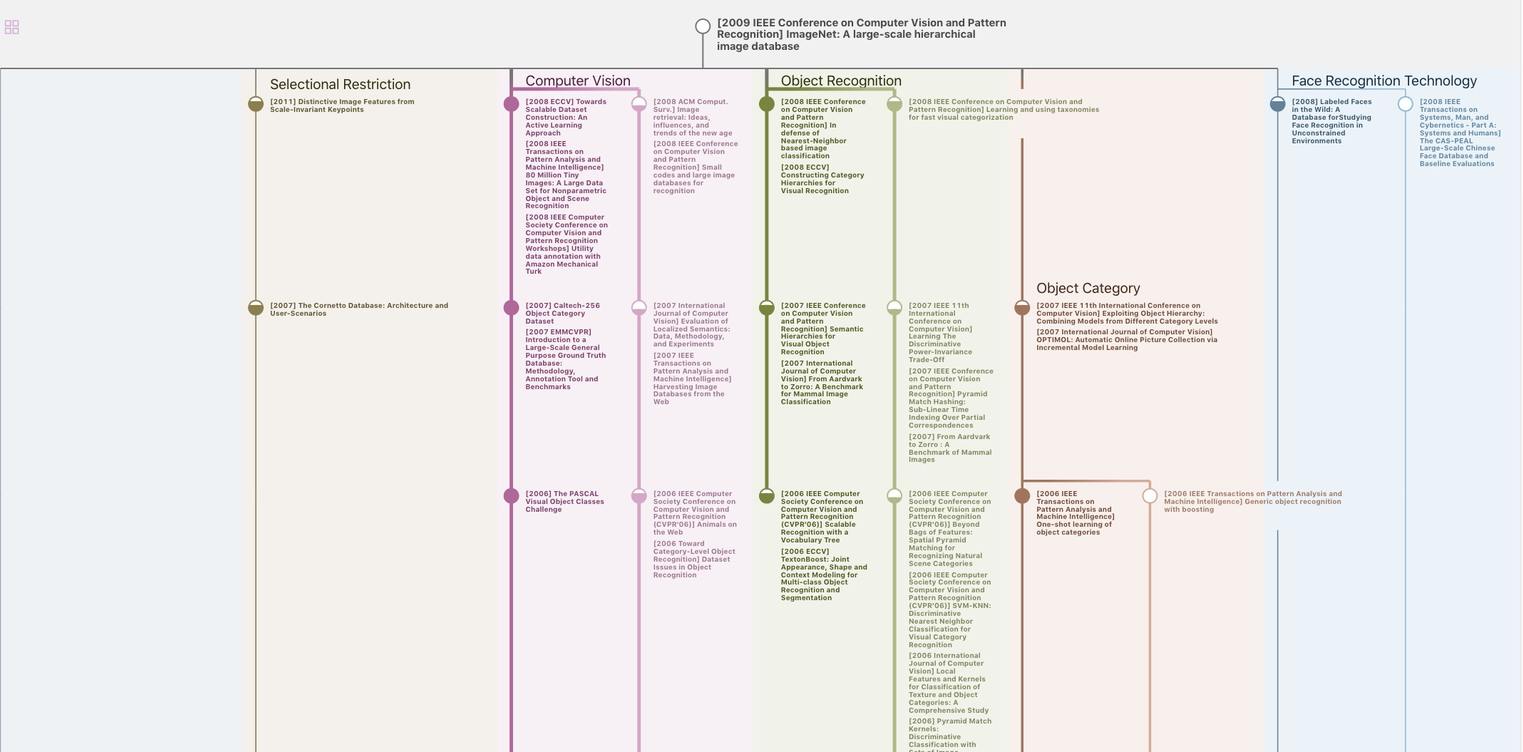
生成溯源树,研究论文发展脉络
Chat Paper
正在生成论文摘要