Geometric and Statistical Models for Analysis of Two-Object Complexes
INTERNATIONAL JOURNAL OF COMPUTER VISION(2023)
摘要
Correlated shape features involving nearby objects often contain important anatomic information. However, it is difficult to capture shape information within and between objects for a joint analysis of multi-object complexes. This paper proposes (1) capturing between-object shape based on an explicit mathematical model called a linking structure, (2) capturing shape features that are invariant to rigid transformation using local affine frames and (3) capturing Correlation of Within- and Between-Object (CoWBO) shape features using a statistical method called NEUJIVE. The resulting correlated shape features give comprehensive understanding of multi-object complexes from various perspectives. First, these features explicitly account for the positional and geometric relations between objects that can be anatomically important. Second, the local affine frames give rise to rich interior geometric features that are invariant to global alignment. Third, the joint analysis of within- and between-object shape yields robust and useful features. To demonstrate the proposed methods, we classify individuals with autism and controls using the extracted shape features of two functionally related brain structures, the hippocampus and the caudate. We found that the CoWBO features give the best classification performance among various choices of shape features. Moreover, the group difference is statistically significant in the feature space formed by the proposed methods.
更多查看译文
关键词
Joint shape representations,Multi-object shape analysis,Local reference frames,Shape classification,S-reps
AI 理解论文
溯源树
样例
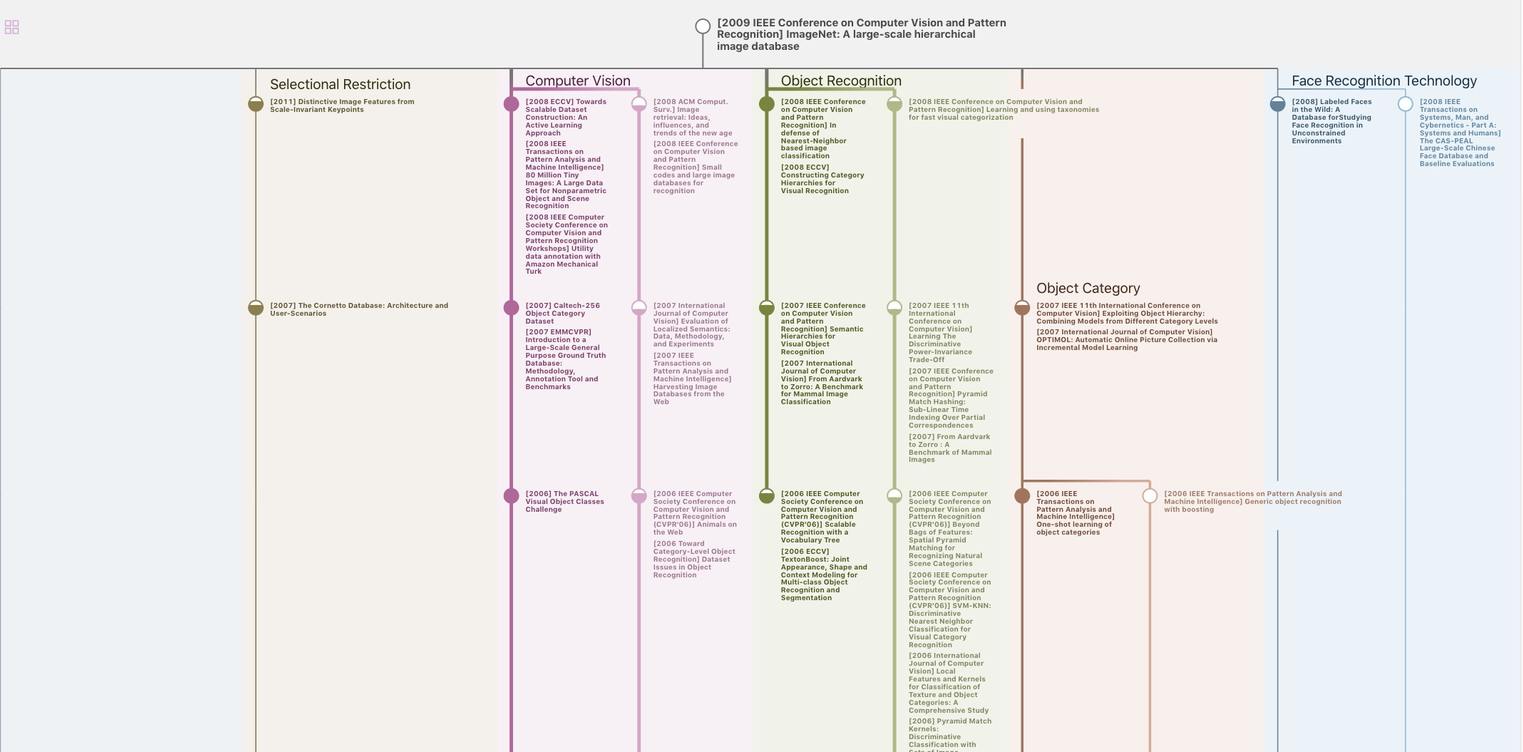
生成溯源树,研究论文发展脉络
Chat Paper
正在生成论文摘要