Transformer-based contrastive learning framework for image anomaly detection
INTERNATIONAL JOURNAL OF MACHINE LEARNING AND CYBERNETICS(2023)
摘要
Anomaly detection refers to the problem of uncovering patterns in a given data set that do not conform to the expected behavior. Recently, owing to the continuous development of deep representation learning, a large number of anomaly detection approaches based on deep learning models have been developed and achieved promising performance. In this work, an image anomaly detection approach based on contrastive learning framework is proposed. Rather than adopting ResNet or other CNN-based deep neural networks as in most of the previous deep learning-based image anomaly detection approaches to learn representations from training samples, a contrastive learning framework is developed for anomaly detection in which Transformer is adopted for extracting better representations. Then, we develop a triple contrastive loss function and embed it into the proposed contrastive learning framework to alleviate the problem of catastrophic collapse that is often encountered in many anomaly detection approaches. Furthermore, a nonlinear Projector is integrated with our model to improve the performance of anomaly detection. The effectiveness of our image anomaly detection approach is validated through experiments on multiple benchmark data sets. According to the experimental results, our approach can obtain better or comparative performance in comparison with state-of-the-art anomaly detection approaches.
更多查看译文
关键词
Anomaly detection,Contrastive learning,Transformer,Triple contrastive loss
AI 理解论文
溯源树
样例
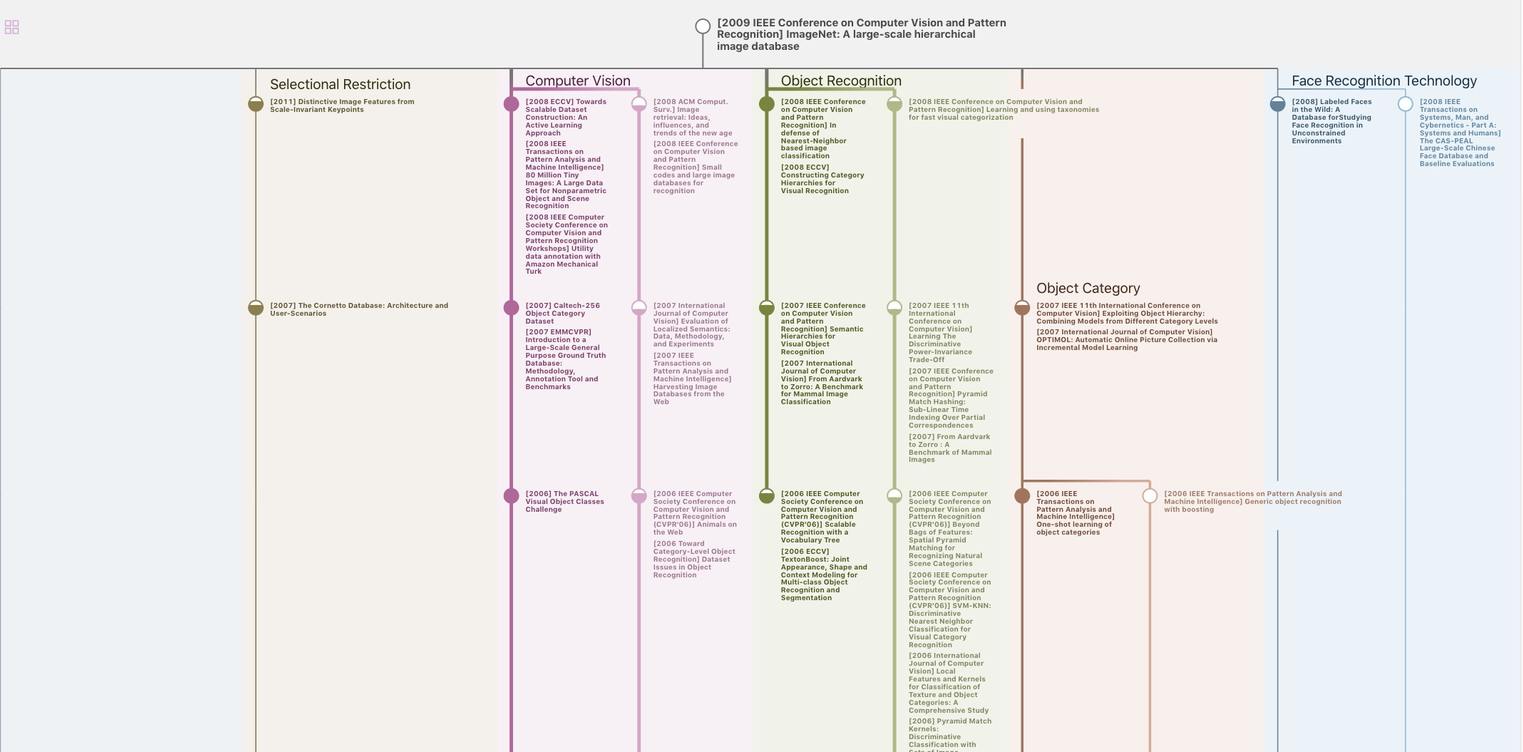
生成溯源树,研究论文发展脉络
Chat Paper
正在生成论文摘要