DeepGCSS: a robust and explainable contour classifier providing generalized curvature scale space features
NEURAL COMPUTING & APPLICATIONS(2023)
摘要
In this paper, we build a novel, robust, and explainable deep neural network architecture for contour classification whose feature extraction layers are a deep version of the Generalized CSS (Generalized Curvature Scale Space) descriptors. For particular kernels, the proposed model behaves exactly like GCSS when extracting areas with strong curvatures. Such architecture is firstly essential to establish a comparison between the efficiency of hand-crafted kernels and the learned ones and secondly to study the ability of the classifier to map the input data into an invariant representation. Experimental results on MPEG-7 and MNIST contour datasets prove that the feature extraction block with hand-crafted kernels leads to an invariant and explainable CSS-based representation. Even though the number of parameters in the DeepGCSS model is much smaller compared to the conventional contour classifiers, the performance remains close. The robustness study was carried out using the ContourVerifier and proves that the features extraction block with hand-crafted kernels leads to a more robust GCSS-based representation model.
更多查看译文
关键词
Contour classification,Explainable AI,Curvature scale space: CSS,Generalized curvature scale space: GCSS,Convolutional neural network: CNN
AI 理解论文
溯源树
样例
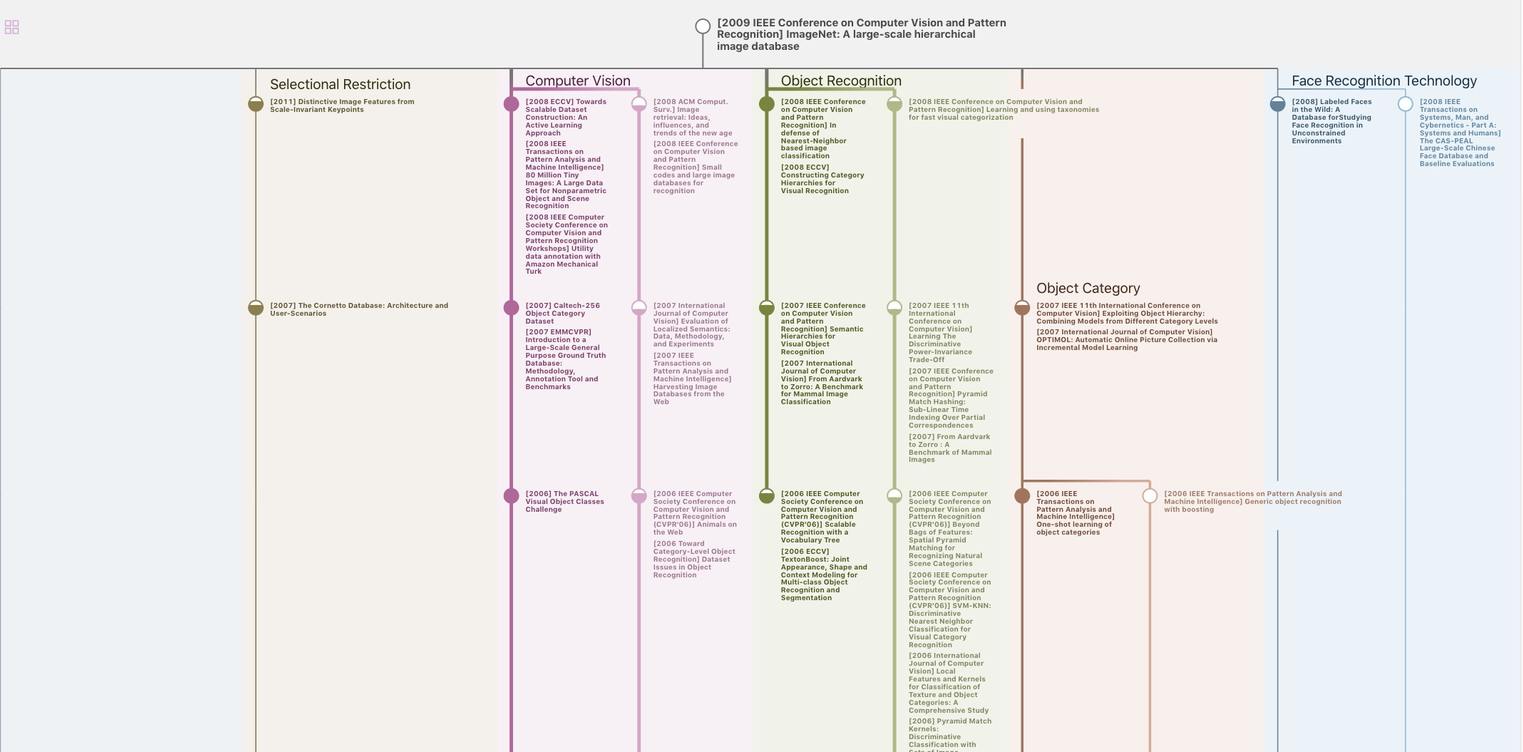
生成溯源树,研究论文发展脉络
Chat Paper
正在生成论文摘要