Early diagnosis and clinical score prediction of Parkinson's disease based on longitudinal neuroimaging data
NEURAL COMPUTING & APPLICATIONS(2023)
摘要
Parkinson's disease (PD) is an irreversible neurodegenerative disease that has serious impacts on patients' lives. To provide timely accurate treatment and delay the deterioration of the disease, the accurate early diagnosis and clinical score prediction of PD are extremely important. Differing from previous studies on PD, we propose a network combining feature selection method with feature learning to obtain the most discriminative feature representation for longitudinal early diagnosis and clinical score prediction. Specifically, we first preprocess the multi-modal neuroimaging data at multiple time points to extract original longitudinal multi-modal features. Then, the feature selection method is performed to preliminary reduce the feature dimensions. Finally, the stacked sparse nonnegative autoencoder (SSNAE) is employed to obtain more discriminative longitudinal multi-modal features to improve the accuracy of early diagnosis and clinical score prediction at multiple time points. To verify our proposed network, diverse and extensive experiments are performed on the Parkinson's Progression Markers Initiative dataset, which aims to identify biological markers of PD risk, onset and progression. The results demonstrate that our proposed method is more efficient and achieves promising performance on both longitudinal early diagnosis and clinical scores prediction compared to state-of-the-art methods.
更多查看译文
关键词
Parkinson's disease, Early diagnosis, Classification, Prediction, Longitudinal data
AI 理解论文
溯源树
样例
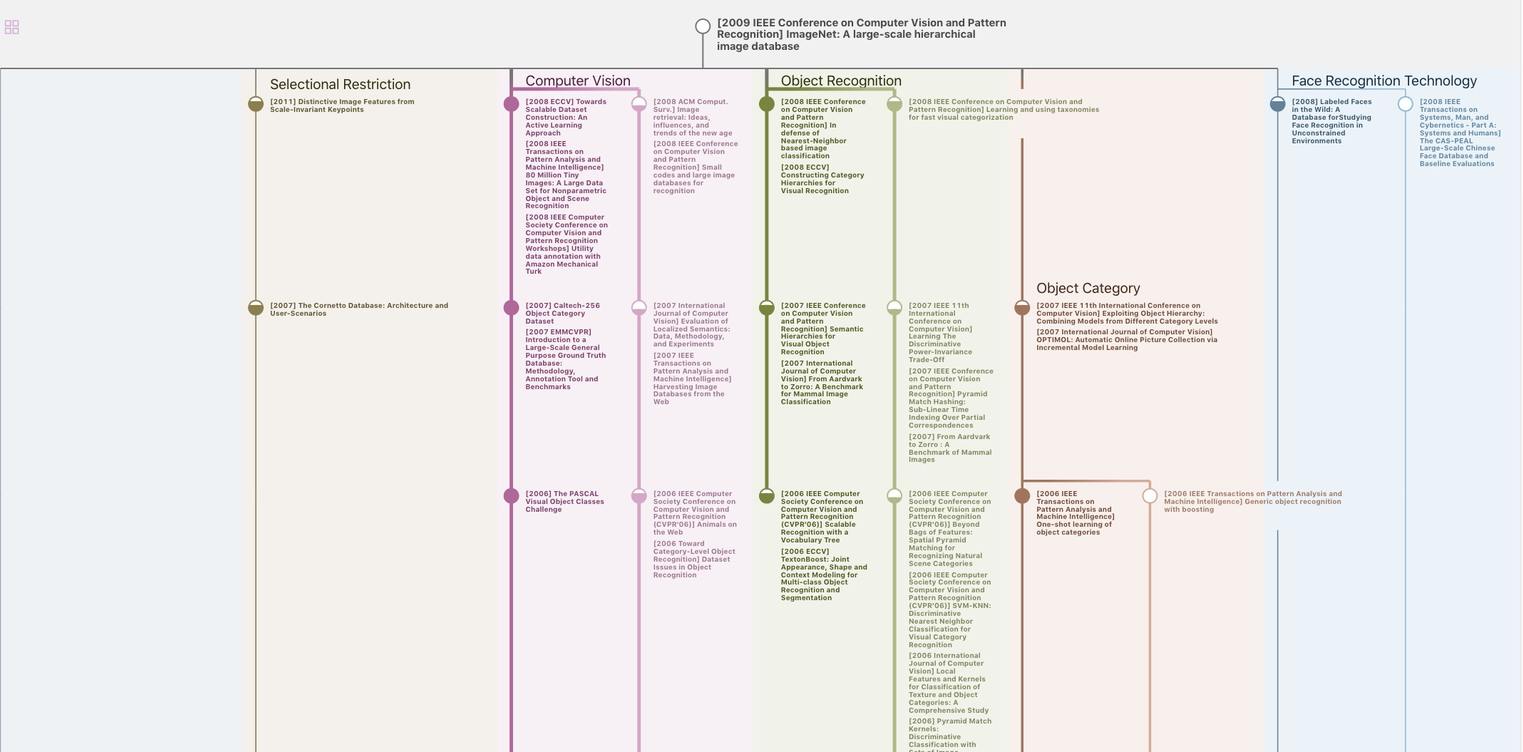
生成溯源树,研究论文发展脉络
Chat Paper
正在生成论文摘要