Covariance discriminative power of kernel clustering methods
ELECTRONIC JOURNAL OF STATISTICS(2023)
摘要
Let x(1), center dot center dot center dot, x(n) be independent observations of size p, each of them belonging to one of c distinct classes. We assume that observa-tions within the class a are characterized by their distribution N(0, 1/pC(a)) where here C-1, center dot center dot center dot, C-c are some non-negative definite p x p matrices. This paper studies the asymptotic behavior of the symmetric matrix (Phi) over tilde (kl) = root p ((x(k)(T) x(l))(2)delta(k)not equal l) when p and n grow to infinity with n/p -> c(0). Particularly, we prove that, if the class covariance matrices are sufficiently close in a certain sense, the matrix (Phi) over tilde behaves like a low-rank perturbation of a Wigner matrix, presenting possibly some isolated eigenvalues outside the bulk of the semi-circular law. We carry out a careful analysis of some of the isolated eigenvalues of (Phi) over tilde and their associated eigenvectors and illustrate how these results can help understand spectral clustering methods that use (Phi) over tilde as a kernel matrix.
更多查看译文
关键词
discriminative power,kernel
AI 理解论文
溯源树
样例
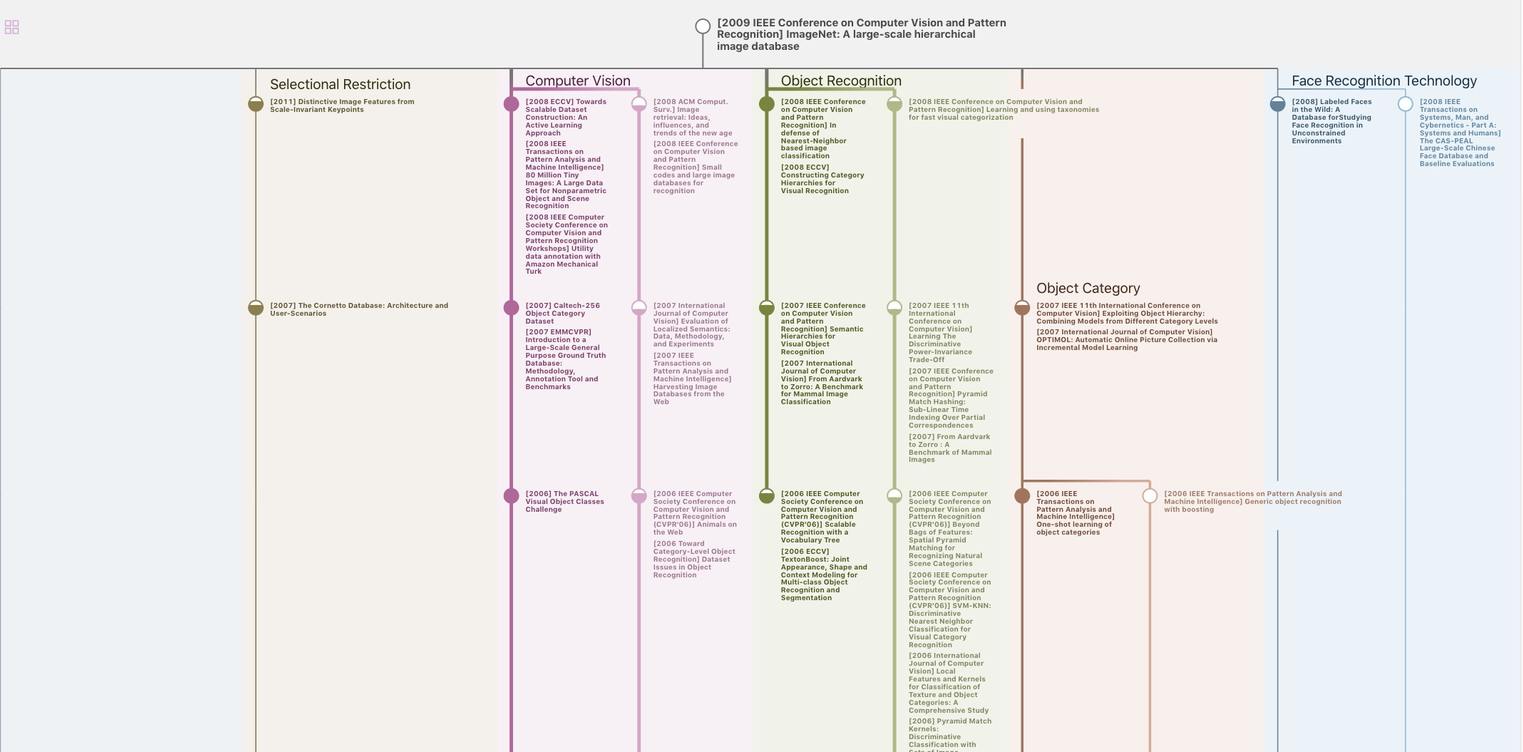
生成溯源树,研究论文发展脉络
Chat Paper
正在生成论文摘要