Hierarchical Spatio-Temporal Change-Point Detection
AMERICAN STATISTICIAN(2023)
摘要
Detecting change-points in multivariate settings is usually carried out by analyzing all marginals either independently, via univariate methods, or jointly, through multivariate approaches. The former discards any inherent dependencies between different marginals and the latter may suffer from domination/masking among different change-points of distinct marginals. As a remedy, we propose an approach which groups marginals with similar temporal behaviors, and then performs group-wise multivariate change-point detection. Our approach groups marginals based on hierarchical clustering using distances which adjust for inherent dependencies. Through a simulation study we show that our approach, by preventing domination/masking, significantly enhances the general performance of the employed multivariate change-point detection method. Finally, we apply our approach to two datasets: (i) Land Surface Temperature in Spain, during the years 2000-2021, and (ii) The WikiLeaks Afghan War Diary data.
更多查看译文
关键词
Clustering, Functional data, Land surface temperature, Multivariate analysis, Point patterns, Satellite images, Trace-variogram
AI 理解论文
溯源树
样例
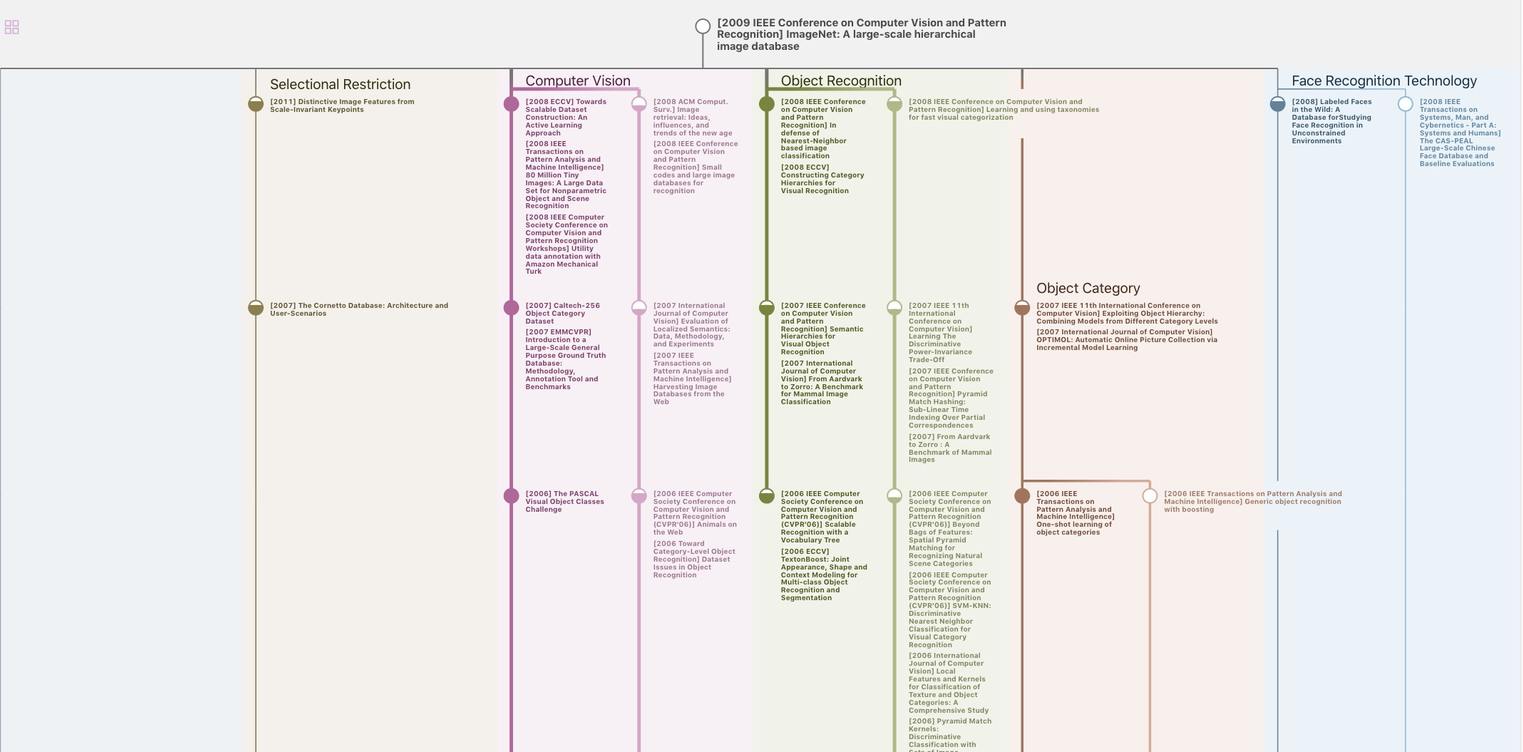
生成溯源树,研究论文发展脉络
Chat Paper
正在生成论文摘要