High-efficient prediction of state of health for lithium-ion battery based on AC impedance feature tuned with Gaussian process regression
Journal of Power Sources(2023)
摘要
The safety of lithium-ion battery (LIB)-powered electric vehicles and stationary energy storage devices relies on a high-efficient state of health (SOH) prediction of the LIB system. In this regard, the health indicators (HIs) play a critical role in determining the SOH prediction performance. Due to the rich kinetic and dynamic information of LIB system measured by electrochemical impedance spectroscopy (EIS), valuable HIs can be extracted from EIS for SOH prediction based on machine learning. The present work is concentrated on how to extract and tune HIs for a high-efficient SOH prediction based on the Gaussian process regression (GPR) model. First, 6-dimension feature xDRT are extracted from 120-dimension impedance xEIS with distribution of relaxation times (DRT) for 4 battery samples; secondly, the extracted xDRT is tuned into xARD by the GPR with automatic relevance determination (ARD) kernels; thirdly, the SOH prediction results show that the tuned xARD needs less training time than the raw xEIS does for the ARD-GPR model. Furthermore, xARD has stronger robustness than xEIS with respect to kernel functions, training sets, and samples. Summarily, the method addressed in the present work to extract HIs may offer a promising solution for battery SOH prediction with a stronger robustness and less training time.
更多查看译文
关键词
State of health,Health indicator,Electrochemical impedance spectroscopy,Distribution of relaxation times,Gaussian process regression
AI 理解论文
溯源树
样例
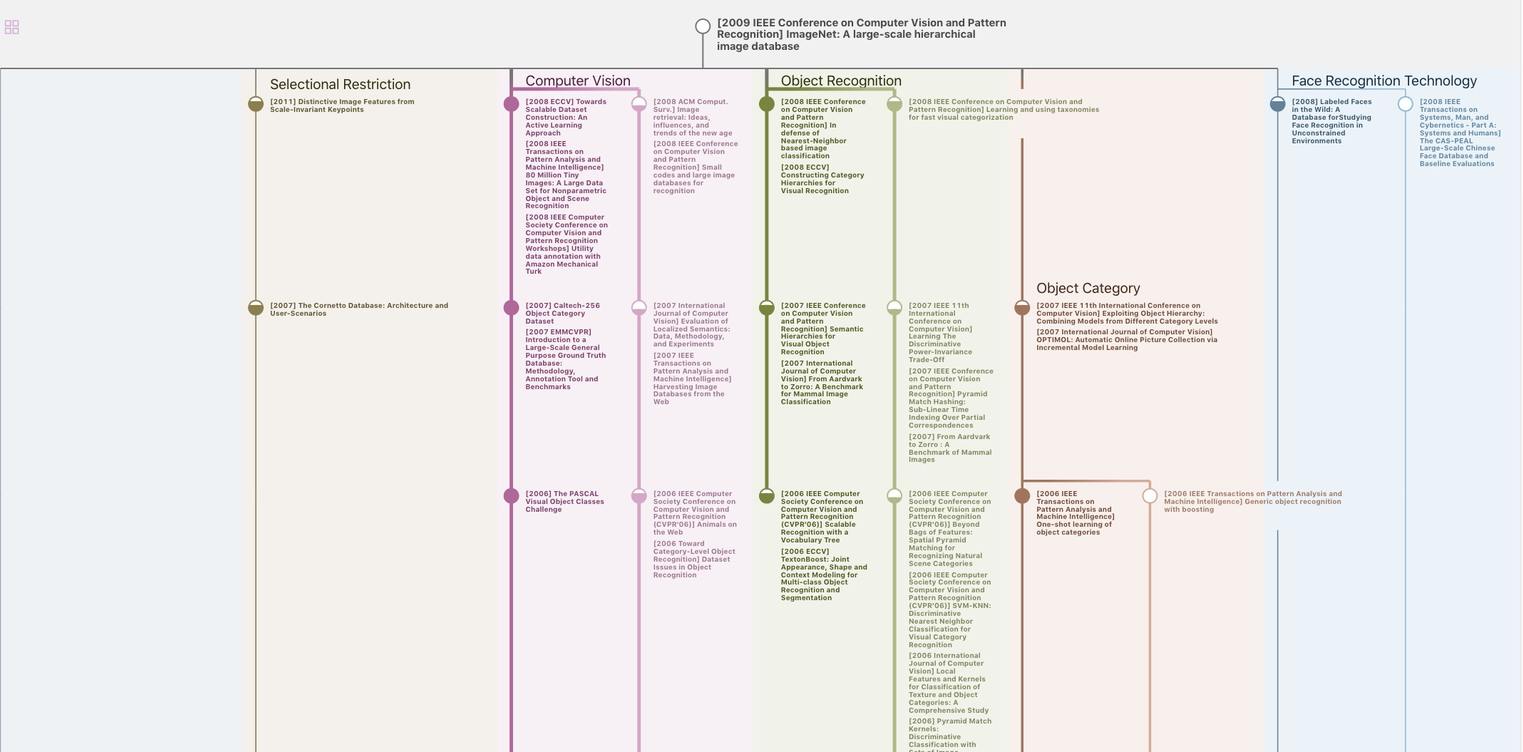
生成溯源树,研究论文发展脉络
Chat Paper
正在生成论文摘要