Automatic detection of photovoltaic facilities from Sentinel-2 observations by the enhanced U-Net method
JOURNAL OF APPLIED REMOTE SENSING(2023)
摘要
With the enactment of supportive government policies and the increasing maturity of solar photovoltaic (PV) technologies, solar PV energy has become the most cost-effective new energy resource worldwide. Geospatial information on existing solar PV power systems is necessary to manage and optimize the deployment of new PV facilities. In this study, we propose a new deep-learning network, named the enhanced U-Net (E-UNET), to detect PV facilities from Sentinel-2 multi-spectral remote sensing data. Our E-UNET features an enhanced encoder-decoder structure that can efficiently extract spectral and spatial features simultaneously by combining a multi-spectral three-dimensional convolution path and a multi-scale pooling block. We compare the performance of the E-UNET with other semantic segmentation deep-learning networks and a pixel-based random forest classifier. The experimental results show that the E-UNET performs better than the other methods. It achieves an overall accuracy, Matthews correlation coefficient, F1, kappa coefficient, and recall of 0.989, 0.862, 0.869, 0.934, and 0.875, respectively. The experimental results also indicate that the E-UNET accurately detects PV facilities from various complex environments with high accuracy in terms of PV integrity and details.
更多查看译文
关键词
enhanced U-Net,semantic segmentation,Sentinel-2,photovoltaic,remote sensing
AI 理解论文
溯源树
样例
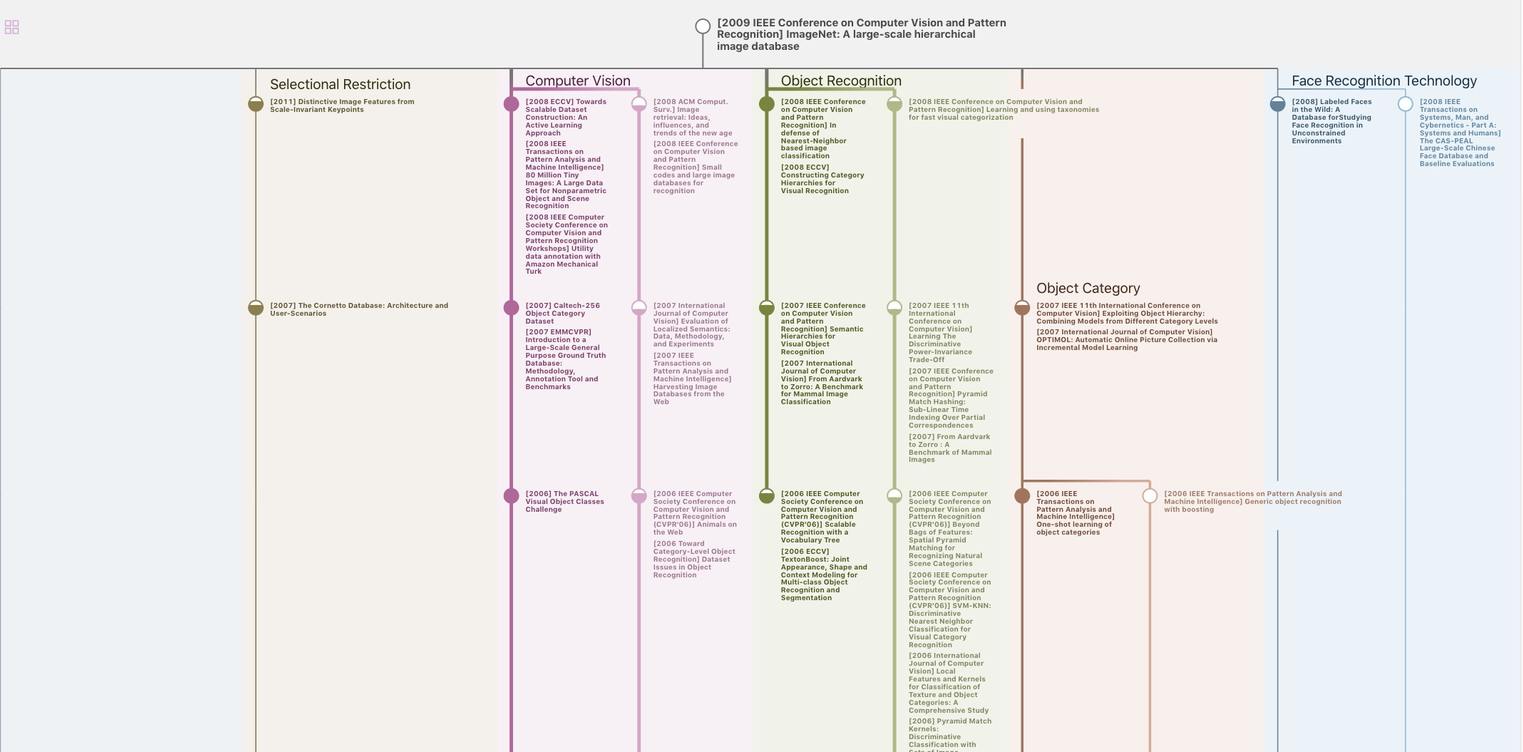
生成溯源树,研究论文发展脉络
Chat Paper
正在生成论文摘要