Sonic Well-Log Imputation Through Machine-Learning-Based Uncertainty Models
PETROPHYSICS(2023)
摘要
Sonic well logs provide critical information to calibrate seismic data and support geomechanical characterization. Advanced subsurface data analytics and machine learning enable new methods and workflows for property estimation, regression, and classification for geoscience and subsurface engineering applications. However, current applications for imputation of well -logging values rely only on model accuracy and low error predictions.Traditional model validation techniques are not enough to validate models and account for the substantial uncertainty in the subsurface. Well-logging imputation estimates and their associated uncertainty models are essential to the field development planning and decision -making workflows, such as reservoir modeling, volumetric resource assessment, predrill prediction with uncertainty, remaining resource mapping, and production allocation.When performing subsurface feature imputation with machine learning, we must expand our machine-learning model training and complexity tuning workflows to check the entire uncertainty model to ensure uncertainty distributions are precise and accurate. We propose a workflow that integrates the goodness metric to calculate accurate and precise uncertainty models of sonic well-log predictions based on ensembles of the machine-learning estimates. Our workflow combines model evaluation and visualization of the estimates and the uncertainty model with respect to measured depth. Our proposed method provides intuitive diagnostics and metrics to evaluate estimation accuracy and uncertainty model goodness.
更多查看译文
关键词
uncertainty,models,well-log,machine-learning-based
AI 理解论文
溯源树
样例
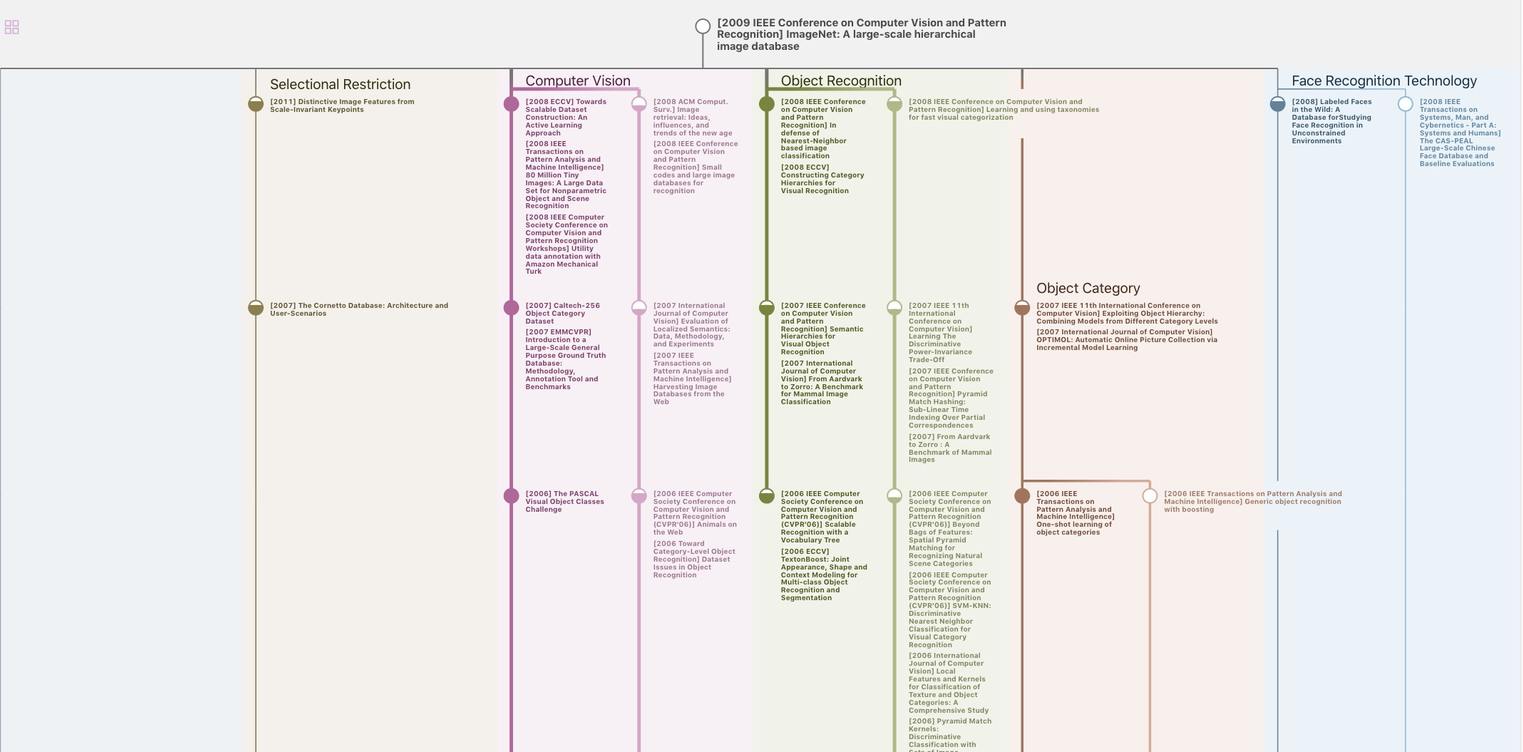
生成溯源树,研究论文发展脉络
Chat Paper
正在生成论文摘要