Automated Morphological Grading of Human Blastocysts From Multi-Focus Images
IEEE TRANSACTIONS ON AUTOMATION SCIENCE AND ENGINEERING(2023)
摘要
This paper reports, for the first time, automated grading of human blastocysts (day-5 embryos) from multi-focus images. Based on a novel attention module, a convolutional neural network (CNN) was developed to predict the morphological grade of a blastocyst. The attention module integrates high-level features extracted from the blastocyst's multi-focus images. Experimental results revealed that multi-focus blastocyst images help improve the grading accuracies than a single blastocyst image. Comparisons of the accuracy achieved by the model and the average accuracy of five embryologists demonstrated that the proposed model can outperform embryologists in the morphological grading of blastocysts (88% versus 86% for development stage prediction, 83% versus 79% for inner cell mass grade prediction, 89% versus 82% for trophectoderm grade prediction). Note to Practitioners-This work was motivated by the subjectivity and significant intra- and inter-evaluator variations in manual morphological grading of blastocysts. Existing approaches to automate the grading process mainly use a single blastocyst image although multi-focus images captured at different focal planes reveal more morphological features of a blastocyst than a single blastocyst image. This paper describes a new CNN-based method using multi-focus images to improve the grading accuracy. The accuracy of the proposed method was verified on multi-focus images of human blastocysts captured by a standard time-lapse incubator at fixed focal depths (-45 mu m, -30 mu m, -15 mu m, 0 mu m, 15 mu m, 30 mu m, 45 mu m).
更多查看译文
关键词
Blastocyst grading,multi-focus images,embryo grading,convolutional neural network (CNN),attention module
AI 理解论文
溯源树
样例
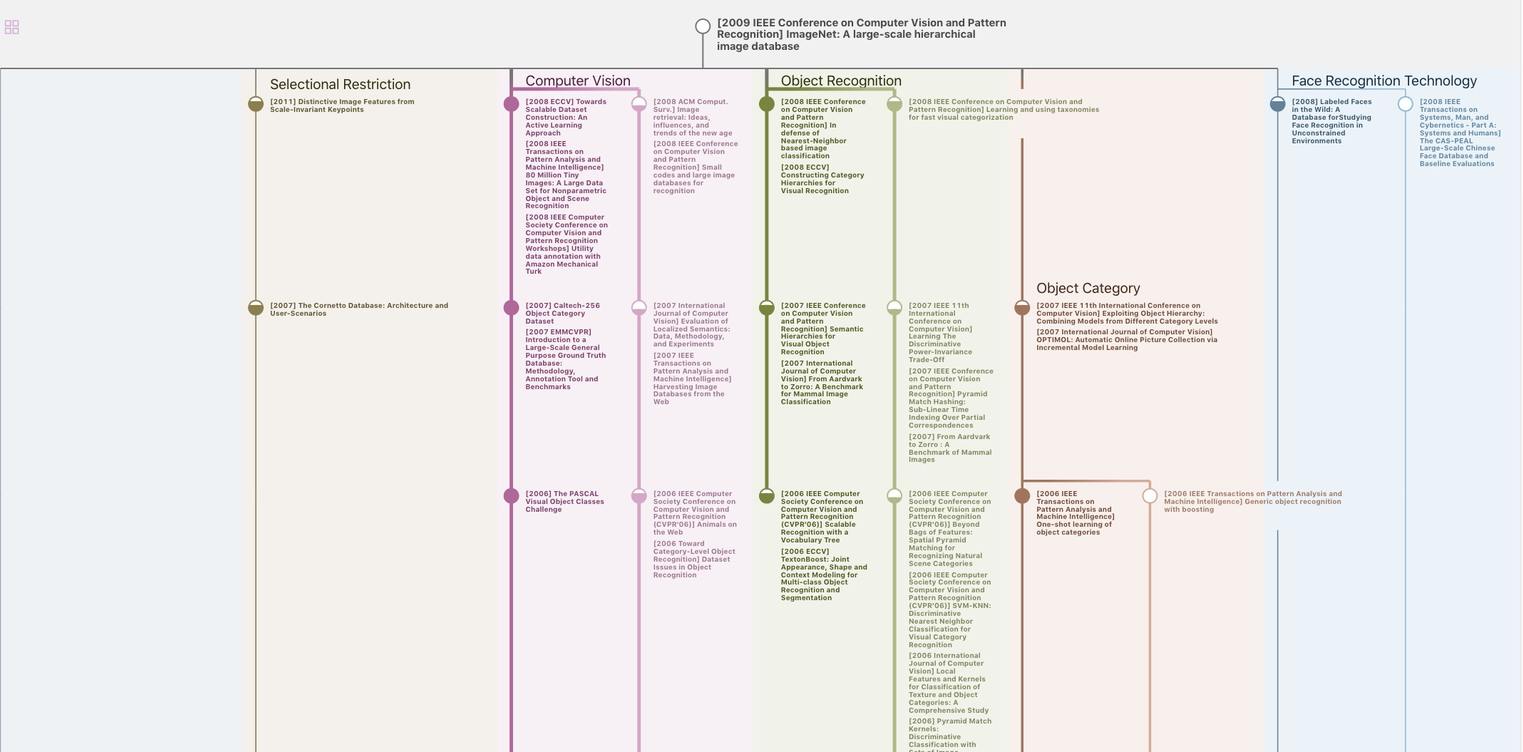
生成溯源树,研究论文发展脉络
Chat Paper
正在生成论文摘要