Error Bounds and the Asymptotic Setting in Kernel-Based Approximation
DOLOMITES RESEARCH NOTES ON APPROXIMATION(2022)
摘要
We use ideas from Gaussian process regression to derive computable error bounds that can be used as stopping criteria in kernel-based approximation. The proposed bounds are based on maximum likelihood estimation and cross-validation of a kernel scale parameter and take the form of a product of the scale parameter estimate and the worst-case approximation error in the reproducing kernel Hilbert space induced by the kernel. We also use known results on the so-called asymptotic setting to argue that such worst-case type error bounds are not necessarily conservative.
更多查看译文
AI 理解论文
溯源树
样例
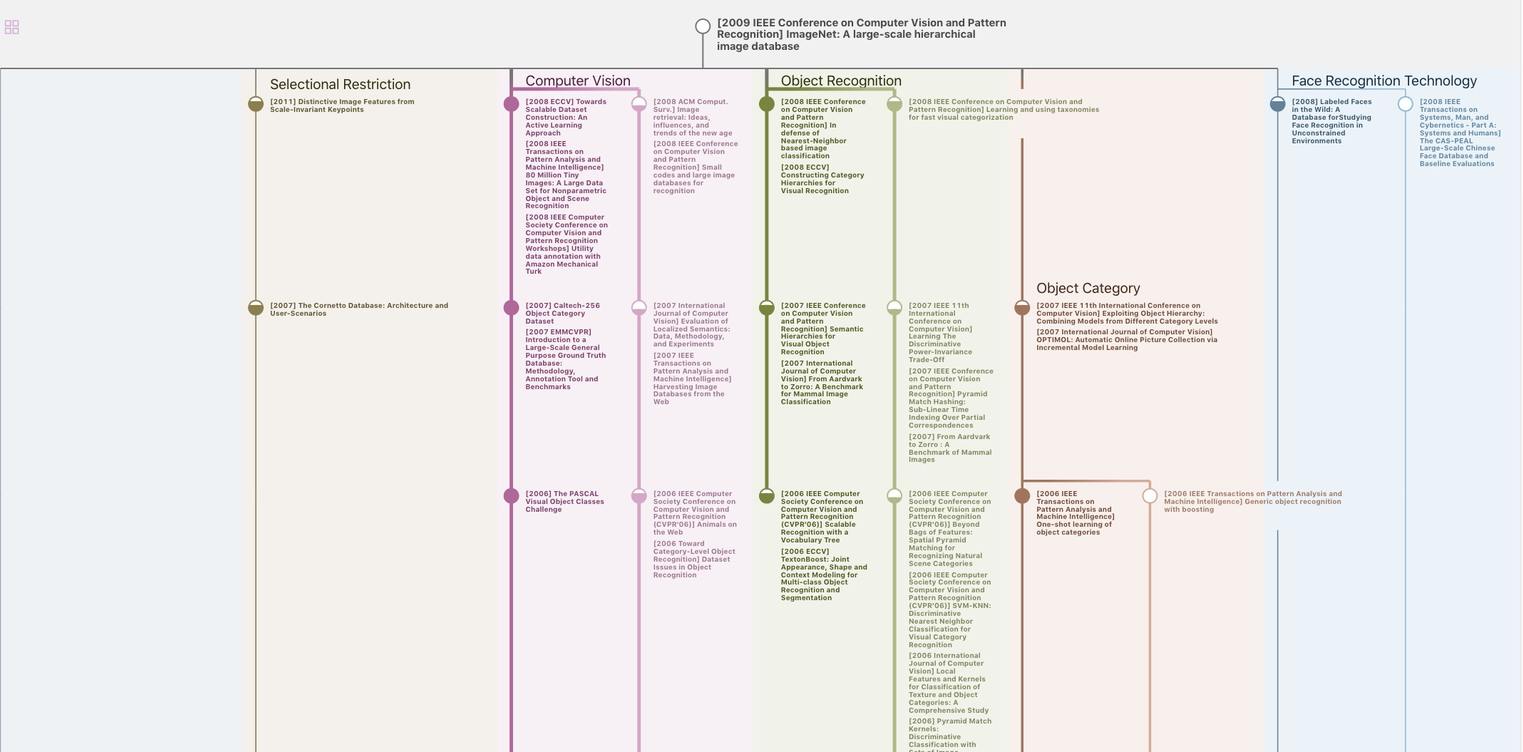
生成溯源树,研究论文发展脉络
Chat Paper
正在生成论文摘要