Predicting Driver Errors during Automated Vehicle Takeovers
TRANSPORTATION RESEARCH RECORD(2023)
摘要
The transition of control between partially automated vehicles and drivers is an important part of the operational design domain and poses unique and important design issues. One approach for enhancing the design of the transition of control mechanisms is to predict driver behavior during a takeover by analyzing his/her state before a takeover. Although there is a wealth of existing literature on modeling the prediction of driver behavior, little is known about the prediction of takeover performance (e.g., driver error) and its underlying data structure (e.g., window sizes or the inclusion of certain features). Thus, the goal of this study is to predict driver error during a takeover event using supervised machine learning algorithms for various window sizes. Three machine learning algorithms (i.e., decision tree, random forest, and support vector machine with a radial basis kernel) were applied to granular driving performance, physiological, and glance data from a driving simulator experiment examining automated vehicle driving. The results showed that a random forest algorithm with an area under the receiver operating characteristic curve of 0.72, trained on a 3 s window before the takeover time, had the best performance with regard to classifying driver error accurately. In addition, we identified the 10 most important predictors that resulted in the best error prediction performance. The results of this study could be useful in developing algorithms for driver state that could be integrated into highly automated systems and, potentially, improve the takeover process.
更多查看译文
关键词
driver behavior, automated driving, transfer of control, machine learning algorithm, predictive modeling, physiological measures
AI 理解论文
溯源树
样例
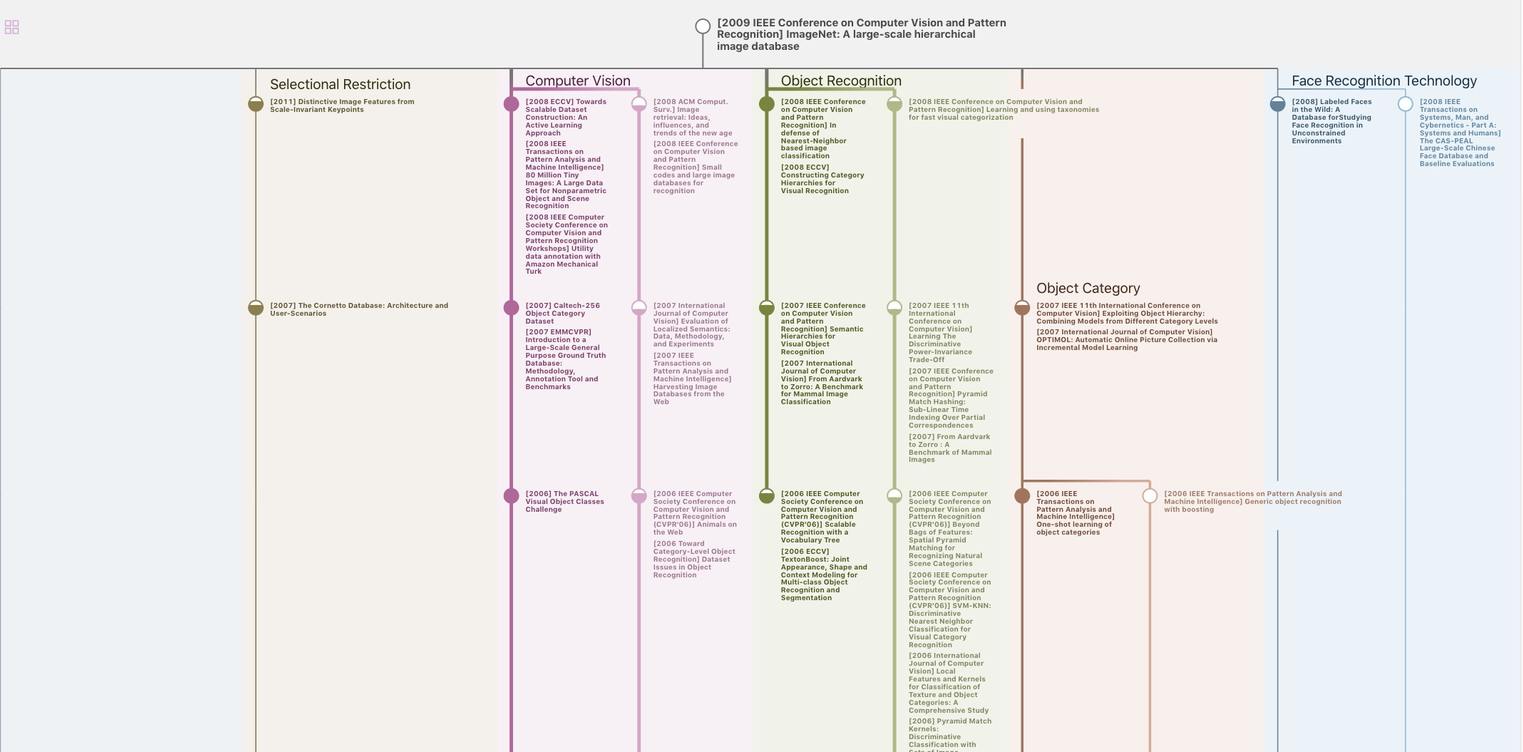
生成溯源树,研究论文发展脉络
Chat Paper
正在生成论文摘要