Distributed Censored Quantile Regression
Journal of Computational and Graphical Statistics(2023)
摘要
This article discusses an extension of censored quantile regression to a distributed setting. With the growing availability of massive datasets, it is oftentimes an arduous task to analyze all the data with limited computational facilities efficiently. Our proposed method, which attempts to overcome this challenge, is comprised of two key steps, namely: (i) estimation of both Kaplan-Meier estimator and model coefficients in a parallel computing environment; (ii) aggregation of coefficient estimations from individual machines. We study the upper limit of the order of the number of machines for this computing environment, which, if fulfilled, guarantees that the proposed estimator converges at a comparable rate to that of the oracle estimator. In addition, we also provide two further modifications for distributed systems including (i) a communication-facilitated adaptation in the sense of Chen, Liu, and Zhang and (ii) a nonparametric counterpart along the direction of Kong and Xia for censored quantile regression. Numerical experiments are conducted to compare the proposed and the existing estimators. The promising results demonstrate the computation efficiency of the proposed methods. Finally, for practical concerns, a cross-validation procedure is also developed which can better select the hyperparameters for the proposed methodologies. for this article are available online.
更多查看译文
关键词
Distributed computing,Lifetime and survival analysis,Quantile estimation regression
AI 理解论文
溯源树
样例
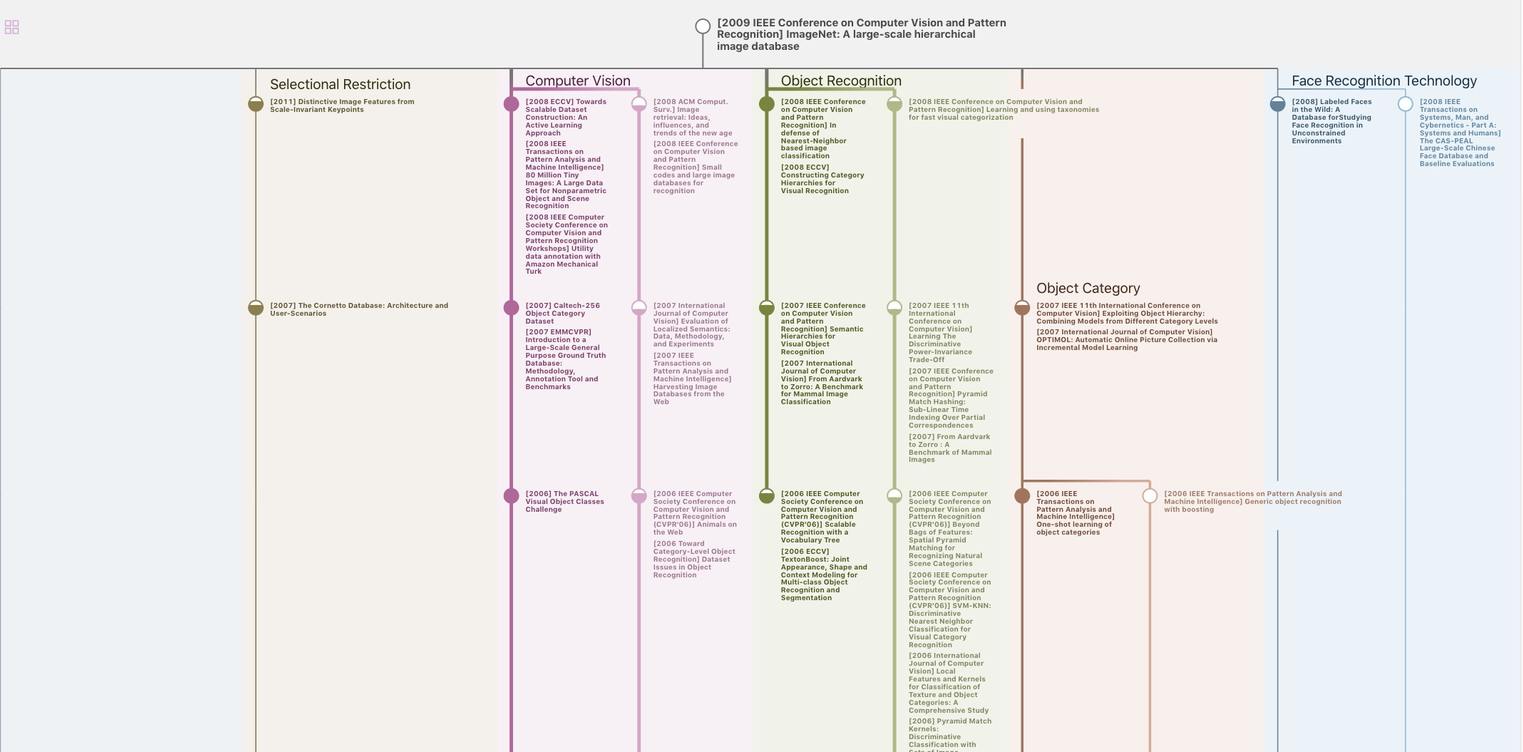
生成溯源树,研究论文发展脉络
Chat Paper
正在生成论文摘要