Multivariate functional outlier detection using the fast massive unsupervised outlier detection indices
STAT(2023)
摘要
We present definitions and properties of the fast massive unsupervised outlier detection (FastMUOD) indices, used for outlier detection (OD) in functional data. FastMUOD detects outliers by computing, for each curve, an amplitude, magnitude, and shape index meant to target the corresponding types of outliers. Some methods adapting FastMUOD to outlier detection in multivariate functional data are then proposed. These include applying FastMUOD on the components of the multivariate data and using random projections. Moreover, these techniques are tested on various simulated and real multivariate functional datasets. Compared with the state of the art in multivariate functional OD, the use of random projections showed the most effective results with similar, and in some cases improved, OD performance. Based on the proportion of random projections that flag each multivariate function as an outlier, we propose a new graphical tool, the magnitude-shape-amplitude (MSA) plot, useful for visualizing the magnitude, shape and amplitude outlyingness of multivariate functional data.
更多查看译文
关键词
functional outlier detection
AI 理解论文
溯源树
样例
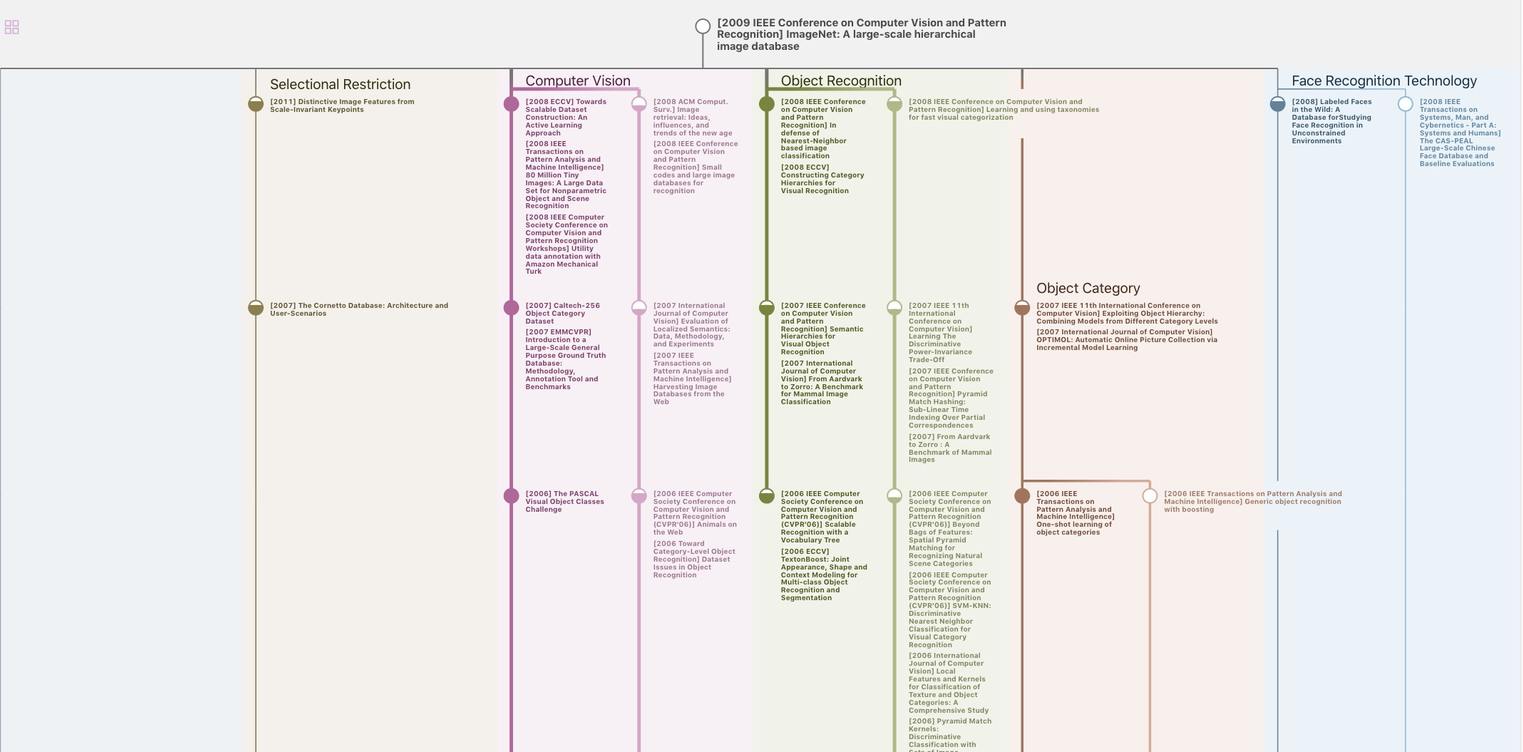
生成溯源树,研究论文发展脉络
Chat Paper
正在生成论文摘要