Go Multivariate: Recommendations on Bayesian Multilevel Hidden Markov Models with Categorical Data.
Multivariate behavioral research(2023)
摘要
The multilevel hidden Markov model (MHMM) is a promising method to investigate intense longitudinal data obtained within the social and behavioral sciences. The MHMM quantifies information on the latent dynamics of behavior over time. In addition, heterogeneity between individuals is accommodated with the inclusion of individual-specific random effects, facilitating the study of individual differences in dynamics. However, the performance of the MHMM has not been sufficiently explored. We performed an extensive simulation to assess the effect of the number of dependent variables (1-8), number of individuals (5-90), and number of observations per individual (100-1600) on the estimation performance of a Bayesian MHMM with categorical data including various levels of state distinctiveness and separation. We found that using multivariate data generally alleviates the sample size needed and improves the stability of the results. Moreover, including variables only consisting of random noise was generally not detrimental to model performance. Regarding the estimation of group-level parameters, the number of individuals and observations largely compensate for each other. However, only the former drives the estimation of between-individual variability. We conclude with guidelines on the sample size necessary based on the level of state distinctiveness and separation and study objectives of the researcher.
更多查看译文
关键词
hidden markov models,recommendations,multivariate
AI 理解论文
溯源树
样例
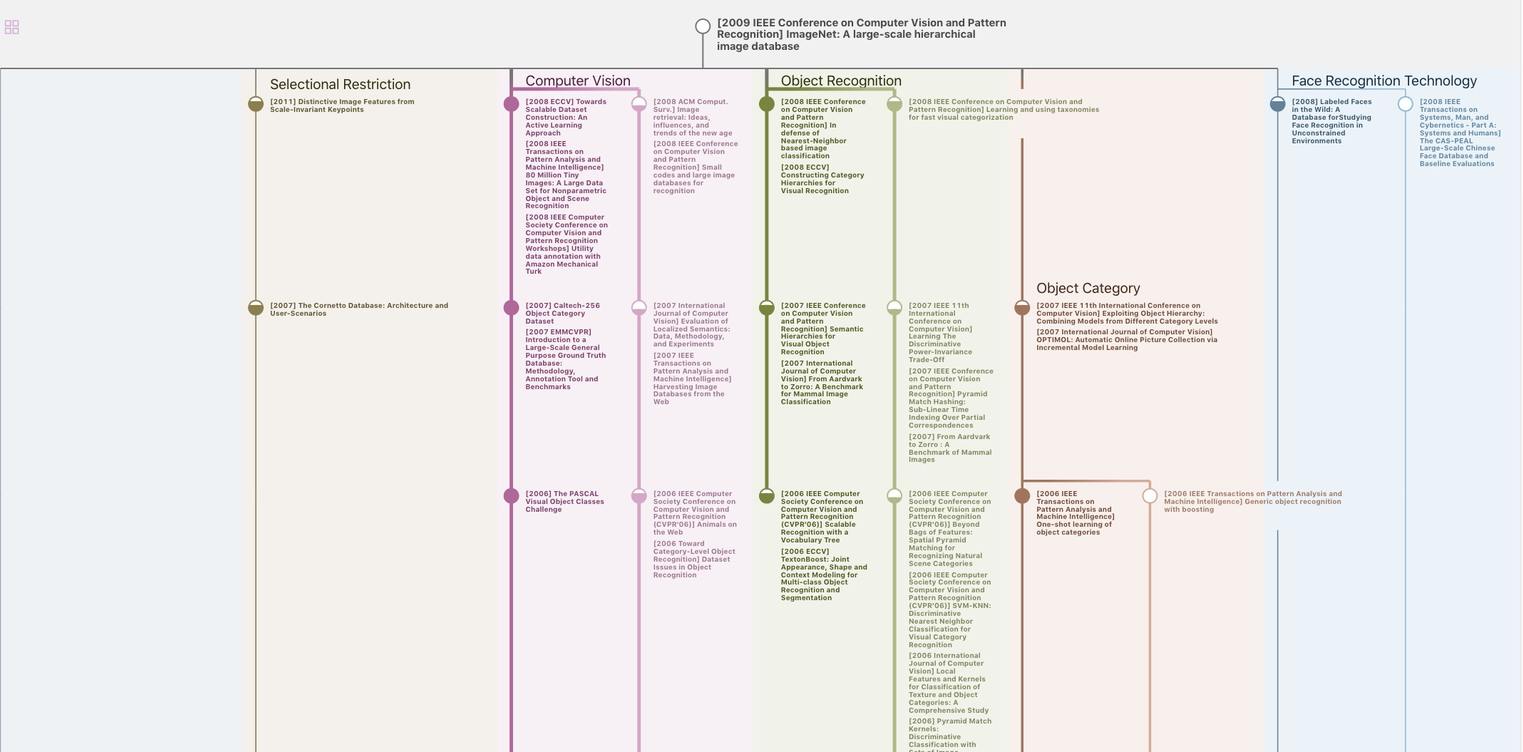
生成溯源树,研究论文发展脉络
Chat Paper
正在生成论文摘要