Multi-Scale Annulus Clustering for Multi-Label Classification
MATHEMATICS(2023)
摘要
Label-specific feature learning has become a hot topic as it induces classification models by accounting for the underlying features of each label. Compared with single-label annotations, multi-label annotations can describe samples from more comprehensive perspectives. It is generally believed that the compelling classification features of a data set often exist in the aggregation of label distribution. In this in-depth study of a multi-label data set, we find that the distance between all samples and the sample center is a Gaussian distribution, which means that the label distribution has the tendency to cluster from the center and spread to the surroundings. Accordingly, the double annulus field based on this distribution trend, named DEPT for double annulusfield and label-specific features for multi-label classification, is proposed in this paper. The double annulus field emphasizes that samples of a specific size can reflect some unique features of the data set. Through intra-annulus clustering for each layer of annuluses, the distinctive feature space of these labels is captured and formed. Then, the final classification model is obtained by training the feature space. Contrastive experiments on 10 benchmark multi-label data sets verify the effectiveness of the proposed algorithm.
更多查看译文
关键词
annulus model, hierarchical clustering, label-specific features, multi-label classification
AI 理解论文
溯源树
样例
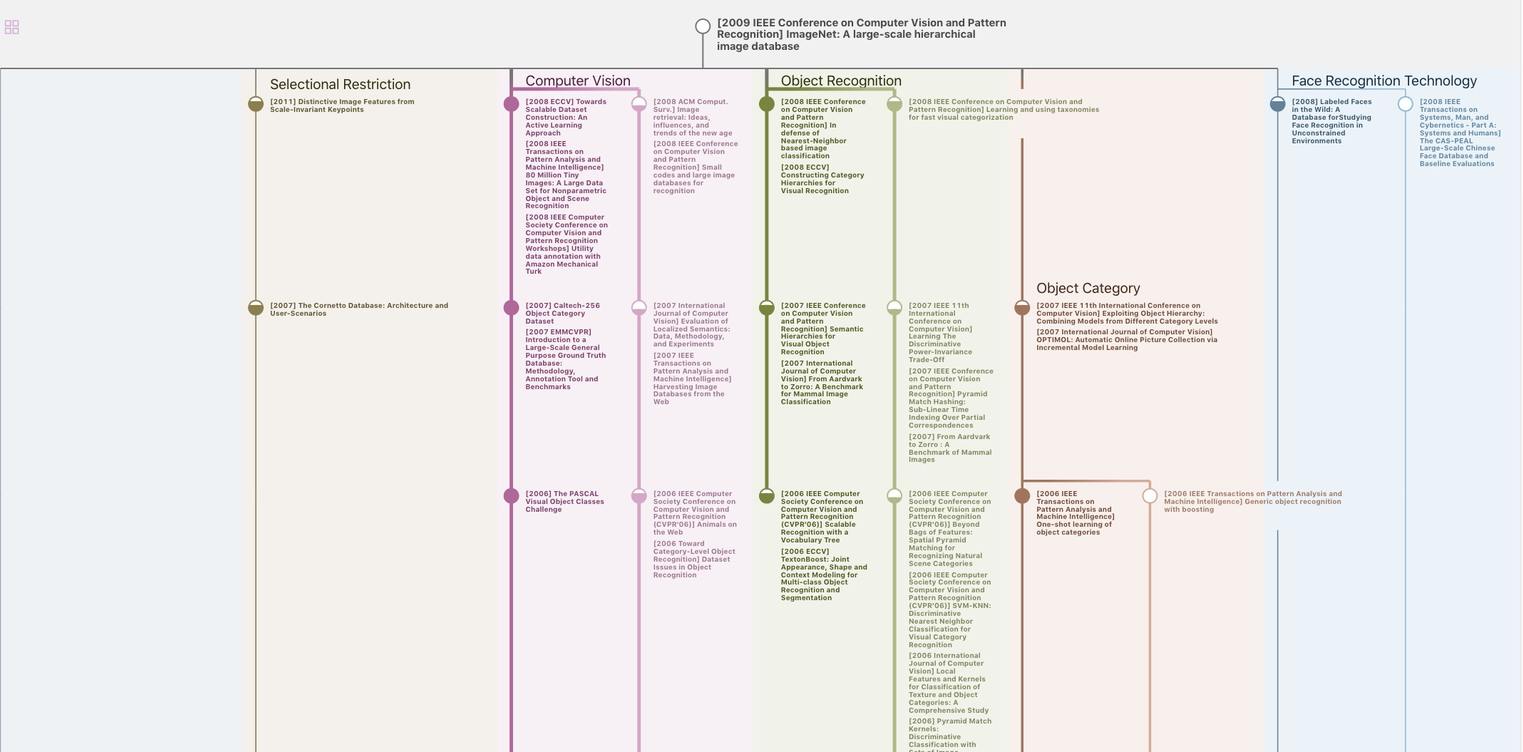
生成溯源树,研究论文发展脉络
Chat Paper
正在生成论文摘要