Dual-scale cnn architecture for covid-19 detection from lung ct images
BIOMEDICAL ENGINEERING-APPLICATIONS BASIS COMMUNICATIONS(2023)
摘要
Coronavirus Disease 2019 (COVID-19) is a terrible illness affecting the respiratory systems of animals and humans. By 2020, this sickness had become a pandemic, affecting millions worldwide. Prevention of the spread of the virus by conducting fast tests for many suspects has become difficult. Recently, many deep learning-based methods have been developed to automatically detect COVID-19 infection from lung Computed Tomography (CT) images of the chest. This paper proposes a novel dual-scale Convolutional Neural Network (CNN) architecture to detect COVID-19 from CT images. The network consists of two different convolutional blocks. Each path is similarly constructed with multi-scale feature extraction layers. The primary path consists of six convolutional layers. The extracted features from multipath networks are flattened with the help of dropout, and these relevant features are concatenated. The sigmoid function is used as the classifier to identify whether the input image is diseased. The proposed network obtained an accuracy of 99.19%, with an Area Under the Curve (AUC) value of 0.99. The proposed network has a lower computational cost than the existing methods regarding learnable parameters, the number of FLOPS, and memory requirements. The proposed CNN model inherits the benefits of densely linked paths and residuals by utilizing effective feature reuse methods. According to our experiments, the proposed approach outperforms previous algorithms and achieves state-of-the-art results.
更多查看译文
关键词
architecture images,lung,dual-scale
AI 理解论文
溯源树
样例
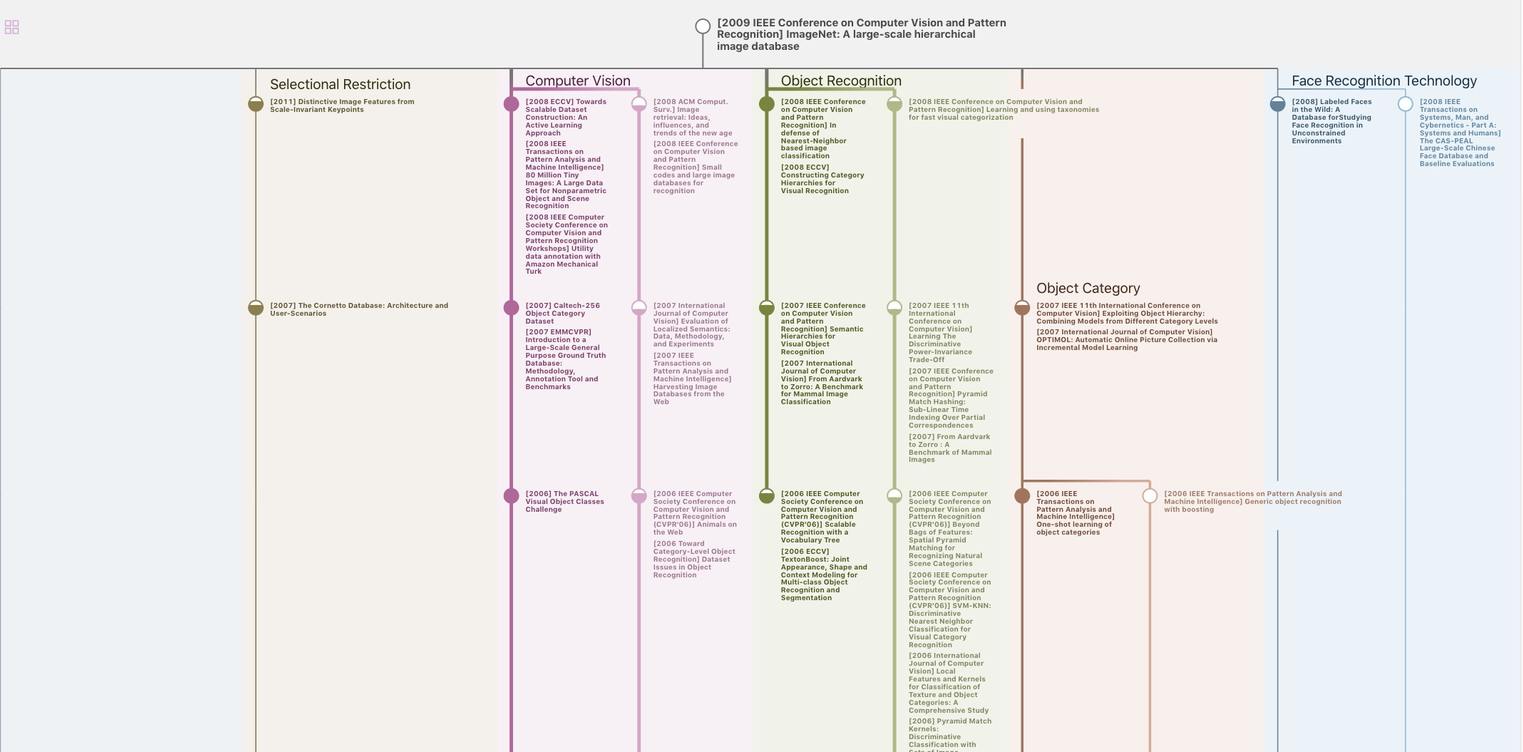
生成溯源树,研究论文发展脉络
Chat Paper
正在生成论文摘要