Distributed regularized online optimization using forward–backward splitting
CONTROL THEORY AND TECHNOLOGY(2023)
摘要
This paper considers the problem of distributed online regularized optimization over a network that consists of multiple interacting nodes. Each node is endowed with a sequence of loss functions that are time-varying and a regularization function that is fixed over time. A distributed forward–backward splitting algorithm is proposed for solving this problem and both fixed and adaptive learning rates are adopted. For both cases, we show that the regret upper bounds scale as 𝒪(√(T)) , where T is the time horizon. In particular, those rates match the centralized counterpart. Finally, we show the effectiveness of the proposed algorithms over an online distributed regularized linear regression problem.
更多查看译文
关键词
Distributed online optimization,Regularized online learning,Regret,Forward-backward splitting
AI 理解论文
溯源树
样例
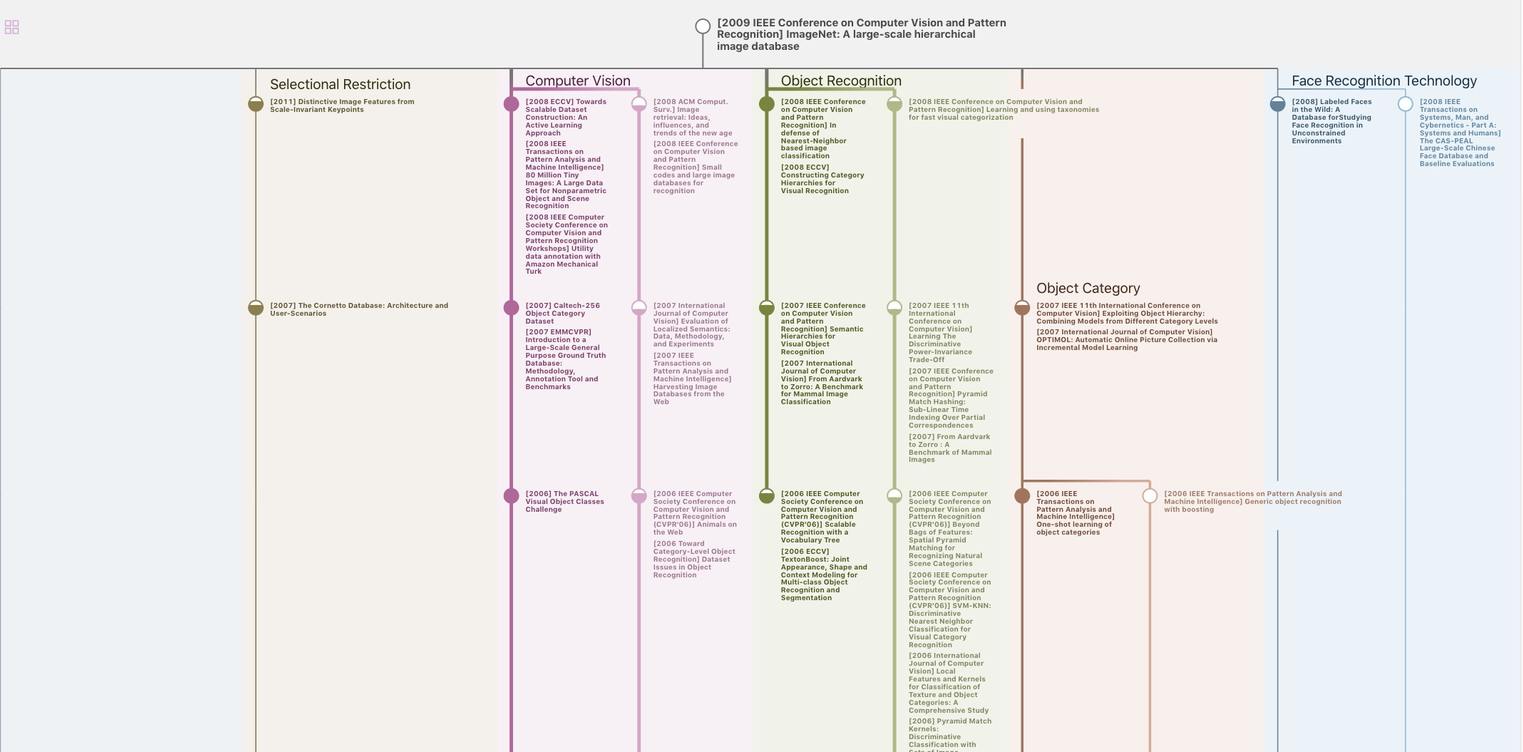
生成溯源树,研究论文发展脉络
Chat Paper
正在生成论文摘要