Diversity maximization-based transfer diagnosis approach of rotating machinery
STRUCTURAL HEALTH MONITORING-AN INTERNATIONAL JOURNAL(2024)
摘要
The existing transfer diagnosis methods based on entropy minimization are easy to lead to trivial solution. To solve this problem, a deep diversity maximization-based adversarial transfer diagnosis approach for rotating machinery is presented in this paper. Firstly, the deep convolution neural network is utilized as the feature encoder to learn the characteristics of vibration signals in different working conditions. The diversity maximization strategy is taken to balance the entropy minimization, so as to avoid trivial local minimum. The categories predicted by nontrivial domain adaptation method are more diverse. Moreover, the entropy is conducted to evaluate the uncertainty of the predicted result of the classifier. Using this deterministic strategy based on entropy to adjust the domain discriminator. The experimental study demonstrates the effectiveness of the developed method.
更多查看译文
关键词
Transfer learning,fault diagnosis,variable working conditions,condition monitoring
AI 理解论文
溯源树
样例
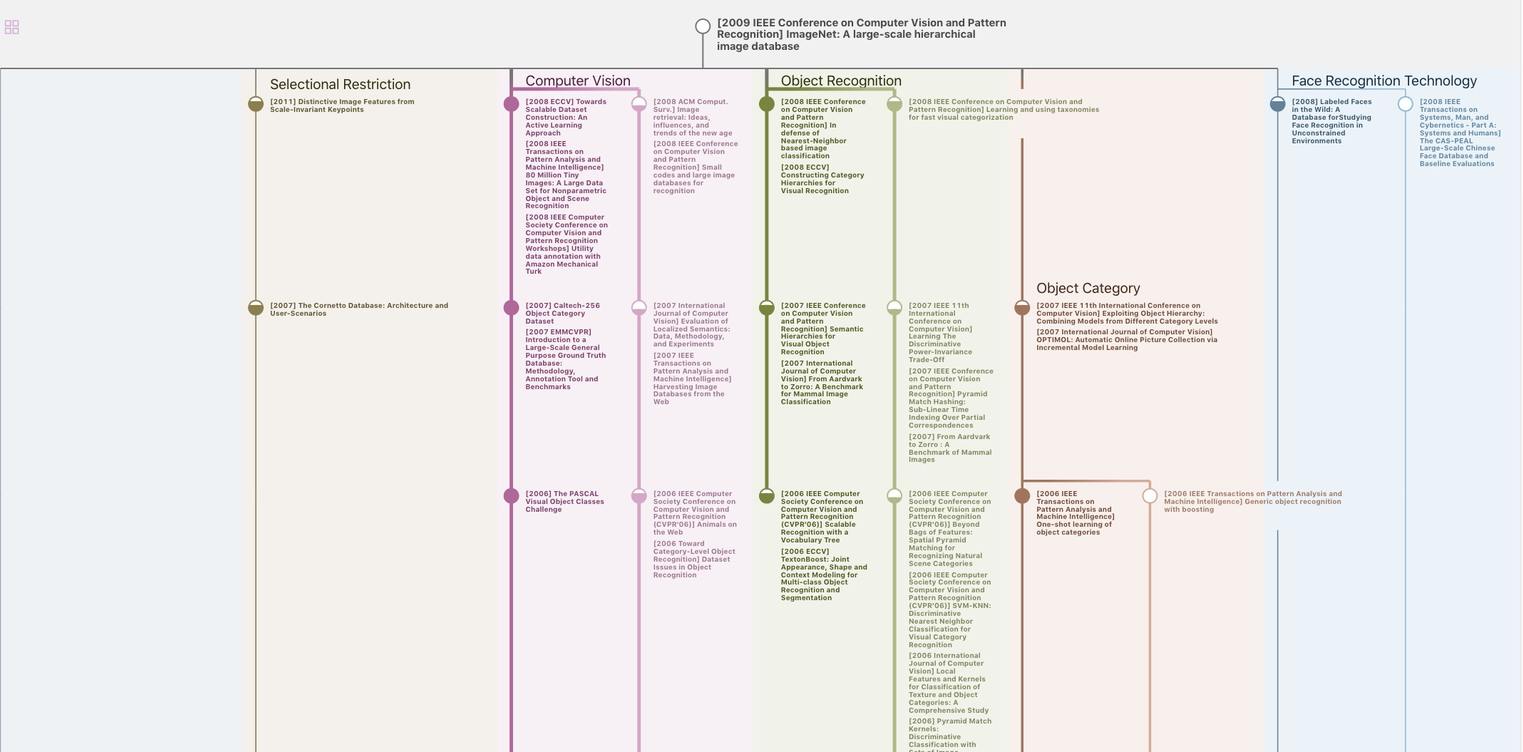
生成溯源树,研究论文发展脉络
Chat Paper
正在生成论文摘要