Global mean-square exponential stability and random periodicity of discrete-time stochastic inertial neural networks with discrete spatial diffusions and Dirichlet boundary condition.
Comput. Math. Appl.(2023)
摘要
This article considers the dual hybrid influences of both discrete spatial diffusions and discrete time in a stochastic inertial neural network via first order exponential Euler difference and second order central finite difference. Based on a non-decomposed constant variation formula, global existence, global mean square boundedness and stability are addressed to the discrete space and time stochastic inertial neural networks. In particular, the optimal exponential convergence rate is discussed by solving nonlinear optimization problem under nonlinear constraints. Further, an implementable computer algorithm is given to calculate the optimal exponential convergence rate. Finally, random periodic solution to the discrete space and time stochastic inertial neural networks is researched by the theories of semi-flow and metric dynamical system and two numerical examples are given to illustrate the feasibility of the derived main results. The research findings show that the spatial diffusions with nonnegative smaller intensive coefficients have no influence on global mean square boundedness and stability, random periodicity of the networks under some suitable assumptions. Meanwhile, the results tell us the bigger strength of the neurons can better ensure global mean square boundedness and stability, random periodicity of the networks. This discussion is pioneering in considering discrete spatial diffusions and offers a studying base for future researches.
更多查看译文
关键词
Inertial neural networks,Discrete spatial diffusion,Stochastic,Random periodic solution,Optimal convergence rate
AI 理解论文
溯源树
样例
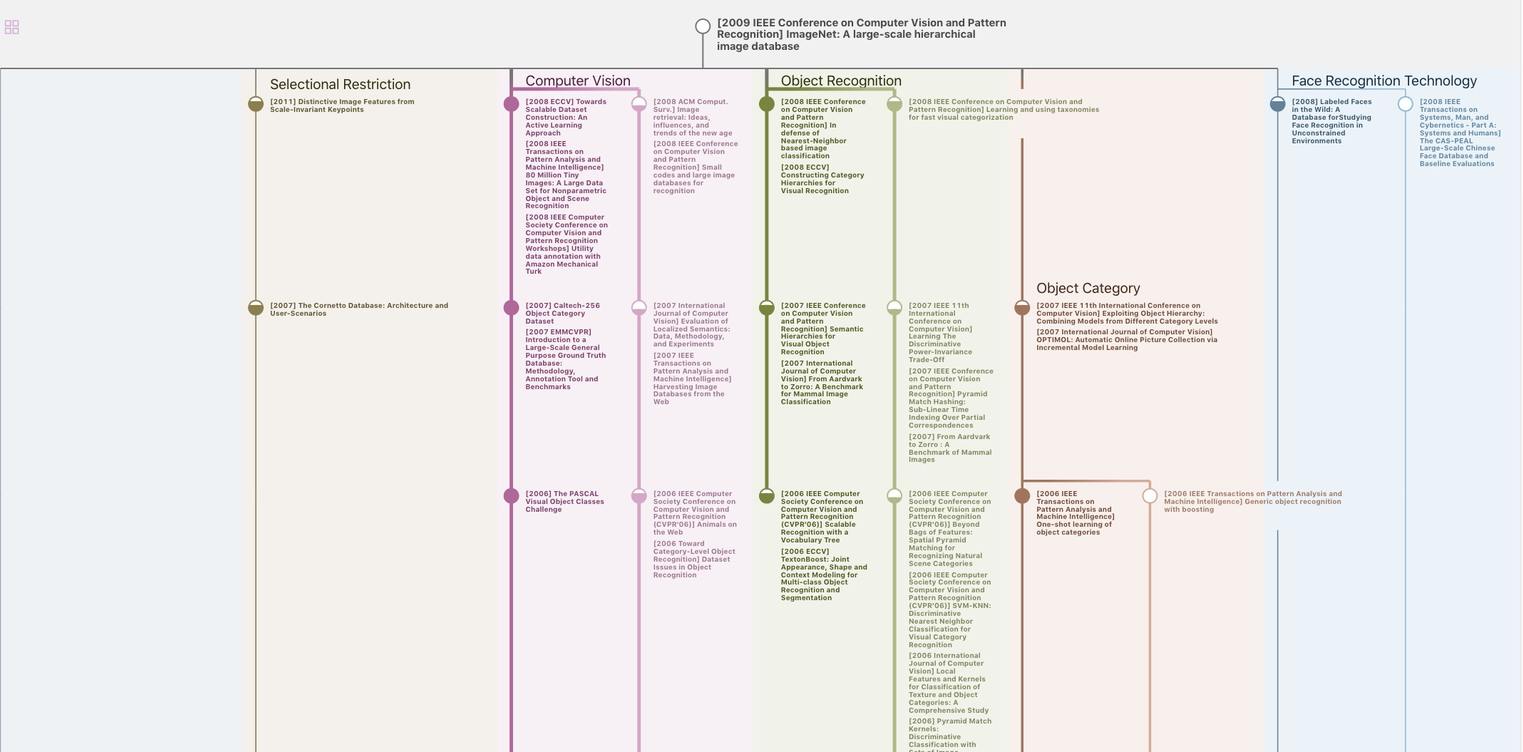
生成溯源树,研究论文发展脉络
Chat Paper
正在生成论文摘要